https://github.com/KarelZe/tclf
A scikit-learn compatible classifier to perform trade classification in Python.
https://github.com/KarelZe/tclf
empirical finance microstructure python rule-based-classifier scikit-learn trade-classification
Last synced: 7 months ago
JSON representation
A scikit-learn compatible classifier to perform trade classification in Python.
- Host: GitHub
- URL: https://github.com/KarelZe/tclf
- Owner: KarelZe
- License: bsd-3-clause
- Created: 2023-07-23T13:39:15.000Z (almost 2 years ago)
- Default Branch: main
- Last Pushed: 2024-12-02T17:41:30.000Z (7 months ago)
- Last Synced: 2024-12-02T18:50:17.748Z (7 months ago)
- Topics: empirical, finance, microstructure, python, rule-based-classifier, scikit-learn, trade-classification
- Language: Python
- Homepage: https://karelze.github.io/tclf/
- Size: 2.84 MB
- Stars: 16
- Watchers: 4
- Forks: 1
- Open Issues: 2
-
Metadata Files:
- Readme: README.md
- Changelog: CHANGELOG.md
- License: LICENSE
- Citation: CITATION.cff
- Codeowners: .github/CODEOWNERS
Awesome Lists containing this project
README
# Trade Classification With Python
[](https://github.com/KarelZe/tclf/actions)
[](https://codecov.io/gh/KarelZe/tclf/tree/main/graph)
[](https://sonarcloud.io/summary/new_code?id=KarelZe_tclf)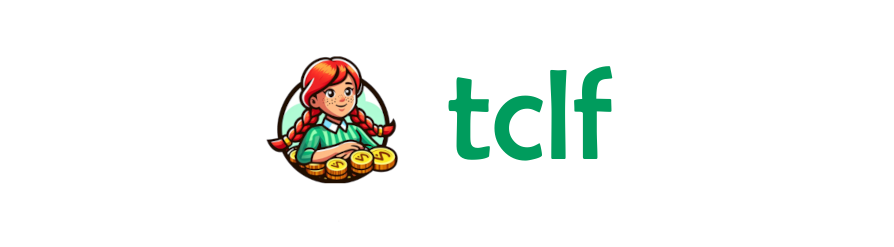
**Documentation βοΈ:** [https://karelze.github.io/tclf/](https://karelze.github.io/tclf/)
**Source Code π:** [https://github.com/KarelZe/tclf](https://github.com/KarelZe/tclf)
`tclf` is a [`scikit-learn`](https://scikit-learn.org/stable/)-compatible implementation of trade classification algorithms to classify financial markets transactions into buyer- and seller-initiated trades.
The key features are:
* **Easy**: Easy to use and learn.
* **Sklearn-compatible**: Compatible to the sklearn API. Use sklearn metrics and visualizations.
* **Feature complete**: Wide range of supported algorithms. Use the algorithms individually or stack them like LEGO blocks.## Installation
**pip**
```console
pip install tclf
```**[uvβ‘](https://github.com/astral-sh/uv)**
```console
uv pip install tclf
```## Supported Algorithms
- (Rev.) CLNV rule[^1]
- (Rev.) EMO rule[^2]
- (Rev.) LR algorithm[^6]
- (Rev.) Tick test[^5]
- Depth rule[^3]
- Quote rule[^4]
- Tradesize rule[^3]For a primer on trade classification rules visit the [rules section π](https://karelze.github.io/tclf/rules/) in our docs.
## Minimal Example
Let's start simple: classify all trades by the quote rule and all other trades, which cannot be classified by the quote rule, randomly.
Create a `main.py` with:
```python title="main.py"
import numpy as np
import pandas as pdfrom tclf.classical_classifier import ClassicalClassifier
X = pd.DataFrame(
[
[1.5, 1, 3],
[2.5, 1, 3],
[1.5, 3, 1],
[2.5, 3, 1],
[1, np.nan, 1],
[3, np.nan, np.nan],
],
columns=["trade_price", "bid_ex", "ask_ex"],
)clf = ClassicalClassifier(layers=[("quote", "ex")], strategy="random")
clf.fit(X)
probs = clf.predict_proba(X)
```
Run your script with
```console
$ python main.py
```
In this example, input data is available as a pd.DataFrame with columns conforming to our [naming conventions](https://karelze.github.io/tclf/naming_conventions/).The parameter `layers=[("quote", "ex")]` sets the quote rule at the exchange level and `strategy="random"` specifies the fallback strategy for unclassified trades.
## Advanced Example
Often it is desirable to classify both on exchange level data and nbbo data. Also, data might only be available as a numpy array. So let's extend the previous example by classifying using the quote rule at exchange level, then at nbbo and all other trades randomly.```python title="main.py" hl_lines="6 16 17 20"
import numpy as np
from sklearn.metrics import accuracy_scorefrom tclf.classical_classifier import ClassicalClassifier
X = np.array(
[
[1.5, 1, 3, 2, 2.5],
[2.5, 1, 3, 1, 3],
[1.5, 3, 1, 1, 3],
[2.5, 3, 1, 1, 3],
[1, np.nan, 1, 1, 3],
[3, np.nan, np.nan, 1, 3],
]
)
y_true = np.array([-1, 1, 1, -1, -1, 1])
features = ["trade_price", "bid_ex", "ask_ex", "bid_best", "ask_best"]clf = ClassicalClassifier(
layers=[("quote", "ex"), ("quote", "best")], strategy="random", features=features
)
clf.fit(X)
acc = accuracy_score(y_true, clf.predict(X))
```
In this example, input data is available as np.arrays with both exchange (`"ex"`) and nbbo data (`"best"`). We set the layers parameter to `layers=[("quote", "ex"), ("quote", "best")]` to classify trades first on subset `"ex"` and remaining trades on subset `"best"`. Additionally, we have to set `ClassicalClassifier(..., features=features)` to pass column information to the classifier.Like before, column/feature names must follow our [naming conventions](https://karelze.github.io/tclf/naming_conventions/).
## Other Examples
For more practical examples, see our [examples section](https://karelze.github.io/tclf/option_trade_classification).
## Development
We are using [`pixi`](https://github.com/prefix-dev/pixi) as a dependency management and workflow tool.
```bash
pixi install
pixi run postinstall
pixi run test
```## Citation
If you are using the package in publications, please cite as:
```latex
@software{bilz_tclf_2023,
author = {Bilz, Markus},
license = {BSD 3},
month = nov,
title = {{tclf} -- trade classification with python},
url = {https://github.com/KarelZe/tclf},
version = {0.0.1},
year = {2023}
}
```## Footnotes
[^1]:
Chakrabarty, B., Li, B., Nguyen, V., & Van Ness, R. A. (2007). Trade classification algorithms for electronic communications network trades. Journal of Banking & Finance, 31(12), 3806β3821. https://doi.org/10.1016/j.jbankfin.2007.03.003
[^2]:Ellis, K., Michaely, R., & OβHara, M. (2000). The accuracy of trade classification rules: Evidence from nasdaq. The Journal of Financial and Quantitative Analysis, 35(4), 529β551. https://doi.org/10.2307/2676254
[^3]:Grauer, C., Schuster, P., & Uhrig-Homburg, M. (2023). Option trade classification. https://doi.org/10.2139/ssrn.4098475
[^4]:Harris, L. (1989). A day-end transaction price anomaly. The Journal of Financial and Quantitative Analysis, 24(1), 29. https://doi.org/10.2307/2330746
[^5]:Hasbrouck, J. (2009). Trading costs and returns for U.s. Equities: Estimating effective costs from daily data. The Journal of Finance, 64(3), 1445β1477. https://doi.org/10.1111/j.1540-6261.2009.01469.x
[^6]:Lee, C., & Ready, M. J. (1991). Inferring trade direction from intraday data. The Journal of Finance, 46(2), 733β746. https://doi.org/10.1111/j.1540-6261.1991.tb02683.x