Ecosyste.ms: Awesome
An open API service indexing awesome lists of open source software.
https://github.com/ahmedfgad/torchga
Train PyTorch Models using the Genetic Algorithm with PyGAD
https://github.com/ahmedfgad/torchga
cnn deep-learning evolutionary-algorithms genetic-algorithm machine-learning neural-networks neuroscience pygad python pytorch torch
Last synced: 6 days ago
JSON representation
Train PyTorch Models using the Genetic Algorithm with PyGAD
- Host: GitHub
- URL: https://github.com/ahmedfgad/torchga
- Owner: ahmedfgad
- Created: 2021-01-03T14:42:52.000Z (about 4 years ago)
- Default Branch: main
- Last Pushed: 2024-09-21T14:41:06.000Z (5 months ago)
- Last Synced: 2025-02-06T23:10:03.997Z (13 days ago)
- Topics: cnn, deep-learning, evolutionary-algorithms, genetic-algorithm, machine-learning, neural-networks, neuroscience, pygad, python, pytorch, torch
- Language: Python
- Homepage: https://pygad.readthedocs.io
- Size: 3.08 MB
- Stars: 97
- Watchers: 5
- Forks: 15
- Open Issues: 2
-
Metadata Files:
- Readme: README.md
- Funding: .github/FUNDING.yml
Awesome Lists containing this project
README
# TorchGA: Training PyTorch Models using the Genetic Algorithm
[TorchGA](https://github.com/ahmedfgad/TorchGA) is part of the [PyGAD](https://pypi.org/project/pygad) library for training [PyTorch](https://pytorch.org) models using the genetic algorithm (GA). This feature is supported starting from [PyGAD](https://pypi.org/project/pygad) 2.10.0.The [TorchGA](https://github.com/ahmedfgad/TorchGA) project has a single module named `torchga.py` which has a class named `TorchGA` for preparing an initial population of PyTorch model parameters.
[PyGAD](https://pypi.org/project/pygad) is an open-source Python library for building the genetic algorithm and training machine learning algorithms. Check the library's documentation at [Read The Docs](https://pygad.readthedocs.io/): https://pygad.readthedocs.io
# Donation
- [Credit/Debit Card](https://donate.stripe.com/eVa5kO866elKgM0144): https://donate.stripe.com/eVa5kO866elKgM0144
- [Open Collective](https://opencollective.com/pygad): [opencollective.com/pygad](https://opencollective.com/pygad)
- PayPal: Use either this link: [paypal.me/ahmedfgad](https://paypal.me/ahmedfgad) or the e-mail address [email protected]
- Interac e-Transfer: Use e-mail address [email protected]# Installation
To install [PyGAD](https://pypi.org/project/pygad), simply use pip to download and install the library from [PyPI](https://pypi.org/project/pygad) (Python Package Index). The library lives a PyPI at this page https://pypi.org/project/pygad.
```python
pip3 install pygad
```To get started with PyGAD, please read the documentation at [Read The Docs](https://pygad.readthedocs.io/) https://pygad.readthedocs.io.
# PyGAD Source Code
The source code of the `PyGAD` modules is found in the following GitHub projects:
- [pygad](https://github.com/ahmedfgad/GeneticAlgorithmPython): (https://github.com/ahmedfgad/GeneticAlgorithmPython)
- [pygad.nn](https://github.com/ahmedfgad/NumPyANN): https://github.com/ahmedfgad/NumPyANN
- [pygad.gann](https://github.com/ahmedfgad/NeuralGenetic): https://github.com/ahmedfgad/NeuralGenetic
- [pygad.cnn](https://github.com/ahmedfgad/NumPyCNN): https://github.com/ahmedfgad/NumPyCNN
- [pygad.gacnn](https://github.com/ahmedfgad/CNNGenetic): https://github.com/ahmedfgad/CNNGenetic
- [pygad.kerasga](https://github.com/ahmedfgad/KerasGA): https://github.com/ahmedfgad/KerasGA
- [pygad.torchga](https://github.com/ahmedfgad/TorchGA): https://github.com/ahmedfgad/TorchGAThe documentation of PyGAD is available at [Read The Docs](https://pygad.readthedocs.io/) https://pygad.readthedocs.io.
# PyGAD Documentation
The documentation of the PyGAD library is available at [Read The Docs](https://pygad.readthedocs.io) at this link: https://pygad.readthedocs.io. It discusses the modules supported by PyGAD, all its classes, methods, attribute, and functions. For each module, a number of examples are given.
If there is an issue using PyGAD, feel free to post at issue in this [GitHub repository](https://github.com/ahmedfgad/GeneticAlgorithmPython) https://github.com/ahmedfgad/GeneticAlgorithmPython or by sending an e-mail to [email protected].
If you built a project that uses PyGAD, then please drop an e-mail to [email protected] with the following information so that your project is included in the documentation.
- Project title
- Brief description
- Preferably, a link that directs the readers to your projectPlease check the **Contact Us** section for more contact details.
# Life Cycle of PyGAD
The next figure lists the different stages in the lifecycle of an instance of the `pygad.GA` class. Note that PyGAD stops when either all generations are completed or when the function passed to the `on_generation` parameter returns the string `stop`.
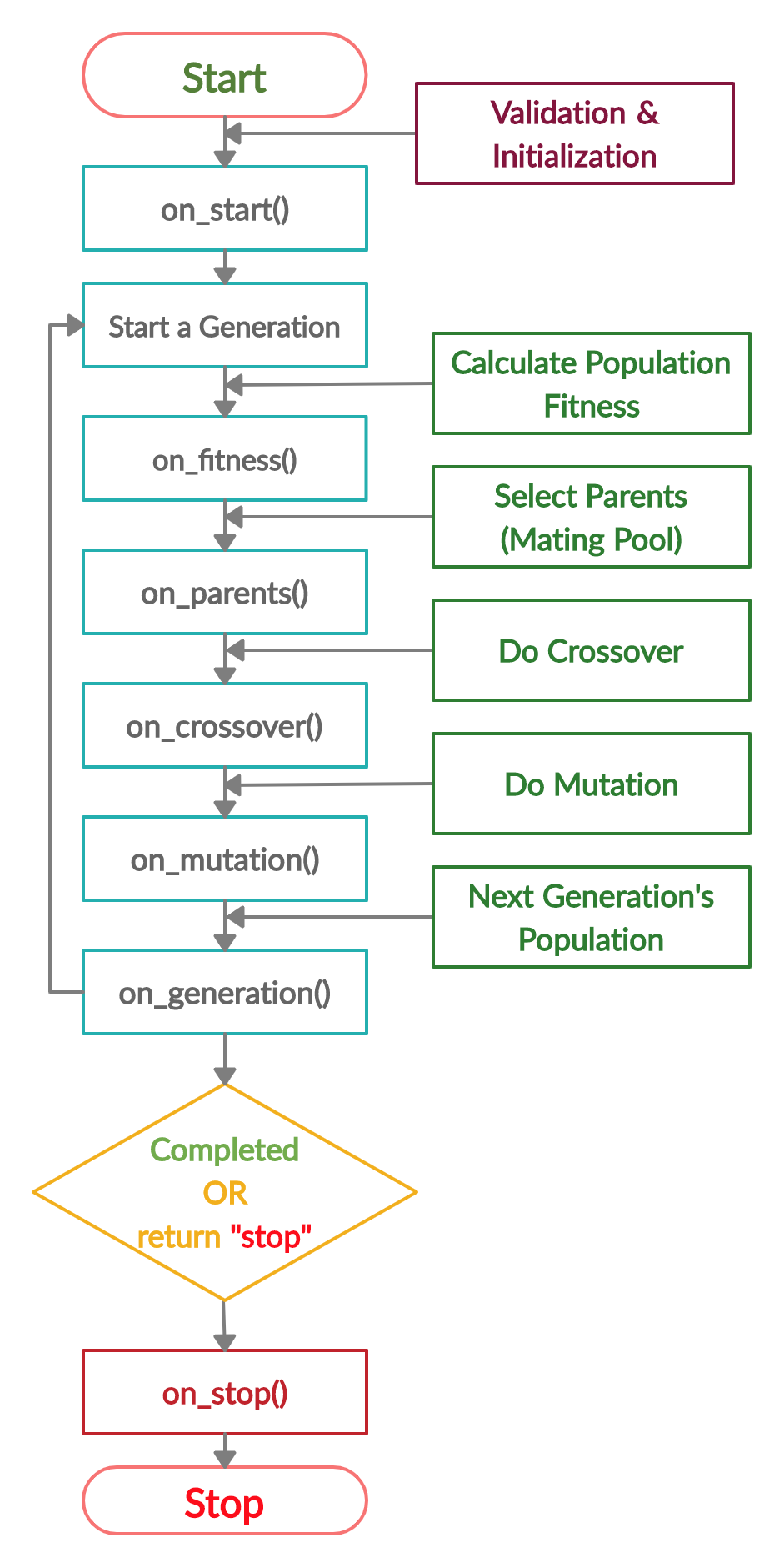
The next code implements all the callback functions to trace the execution of the genetic algorithm. Each callback function prints its name.
```python
import pygad
import numpyfunction_inputs = [4,-2,3.5,5,-11,-4.7]
desired_output = 44def fitness_func(ga_instance, solution, solution_idx):
output = numpy.sum(solution*function_inputs)
fitness = 1.0 / (numpy.abs(output - desired_output) + 0.000001)
return fitnessfitness_function = fitness_func
def on_start(ga_instance):
print("on_start()")def on_fitness(ga_instance, population_fitness):
print("on_fitness()")def on_parents(ga_instance, selected_parents):
print("on_parents()")def on_crossover(ga_instance, offspring_crossover):
print("on_crossover()")def on_mutation(ga_instance, offspring_mutation):
print("on_mutation()")def on_generation(ga_instance):
print("on_generation()")def on_stop(ga_instance, last_population_fitness):
print("on_stop()")ga_instance = pygad.GA(num_generations=3,
num_parents_mating=5,
fitness_func=fitness_function,
sol_per_pop=10,
num_genes=len(function_inputs),
on_start=on_start,
on_fitness=on_fitness,
on_parents=on_parents,
on_crossover=on_crossover,
on_mutation=on_mutation,
on_generation=on_generation,
on_stop=on_stop)ga_instance.run()
```Based on the used 3 generations as assigned to the `num_generations` argument, here is the output.
```
on_start()on_fitness()
on_parents()
on_crossover()
on_mutation()
on_generation()on_fitness()
on_parents()
on_crossover()
on_mutation()
on_generation()on_fitness()
on_parents()
on_crossover()
on_mutation()
on_generation()on_stop()
```# Examples
Check the [PyGAD's documentation](https://pygad.readthedocs.io/en/latest/gacnn.html) for more examples information. You can also find more information about the implementation of the examples.
## Example 1: Regression Model
```python
import torch
import torchga
import pygaddef fitness_func(ga_instance, solution, sol_idx):
global data_inputs, data_outputs, torch_ga, model, loss_functionmodel_weights_dict = torchga.model_weights_as_dict(model=model,
weights_vector=solution)# Use the current solution as the model parameters.
model.load_state_dict(model_weights_dict)predictions = model(data_inputs)
abs_error = loss_function(predictions, data_outputs).detach().numpy() + 0.00000001solution_fitness = 1.0 / abs_error
return solution_fitness
def callback_generation(ga_instance):
print("Generation = {generation}".format(generation=ga_instance.generations_completed))
print("Fitness = {fitness}".format(fitness=ga_instance.best_solution()[1]))# Create the PyTorch model.
input_layer = torch.nn.Linear(3, 5)
relu_layer = torch.nn.ReLU()
output_layer = torch.nn.Linear(5, 1)model = torch.nn.Sequential(input_layer,
relu_layer,
output_layer)
# print(model)# Create an instance of the pygad.torchga.TorchGA class to build the initial population.
torch_ga = torchga.TorchGA(model=model,
num_solutions=10)loss_function = torch.nn.L1Loss()
# Data inputs
data_inputs = torch.tensor([[0.02, 0.1, 0.15],
[0.7, 0.6, 0.8],
[1.5, 1.2, 1.7],
[3.2, 2.9, 3.1]])# Data outputs
data_outputs = torch.tensor([[0.1],
[0.6],
[1.3],
[2.5]])# Prepare the PyGAD parameters. Check the documentation for more information: https://pygad.readthedocs.io/en/latest/pygad.html#pygad-ga-class
num_generations = 250 # Number of generations.
num_parents_mating = 5 # Number of solutions to be selected as parents in the mating pool.
initial_population = torch_ga.population_weights # Initial population of network weightsga_instance = pygad.GA(num_generations=num_generations,
num_parents_mating=num_parents_mating,
initial_population=initial_population,
fitness_func=fitness_func,
on_generation=callback_generation)ga_instance.run()
# After the generations complete, some plots are showed that summarize how the outputs/fitness values evolve over generations.
ga_instance.plot_fitness(title="PyGAD & PyTorch - Iteration vs. Fitness", linewidth=4)# Returning the details of the best solution.
solution, solution_fitness, solution_idx = ga_instance.best_solution()
print("Fitness value of the best solution = {solution_fitness}".format(solution_fitness=solution_fitness))
print("Index of the best solution : {solution_idx}".format(solution_idx=solution_idx))# Fetch the parameters of the best solution.
best_solution_weights = torchga.model_weights_as_dict(model=model,
weights_vector=solution)
model.load_state_dict(best_solution_weights)
predictions = model(data_inputs)
print("Predictions : \n", predictions.detach().numpy())abs_error = loss_function(predictions, data_outputs)
print("Absolute Error : ", abs_error.detach().numpy())
```## Example 2: XOR Binary Classification
```python
import torch
import torchga
import pygaddef fitness_func(ga_instance, solution, sol_idx):
global data_inputs, data_outputs, torch_ga, model, loss_functionmodel_weights_dict = torchga.model_weights_as_dict(model=model,
weights_vector=solution)# Use the current solution as the model parameters.
model.load_state_dict(model_weights_dict)predictions = model(data_inputs)
solution_fitness = 1.0 / (loss_function(predictions, data_outputs).detach().numpy() + 0.00000001)
return solution_fitness
def callback_generation(ga_instance):
print("Generation = {generation}".format(generation=ga_instance.generations_completed))
print("Fitness = {fitness}".format(fitness=ga_instance.best_solution()[1]))# Create the PyTorch model.
input_layer = torch.nn.Linear(2, 4)
relu_layer = torch.nn.ReLU()
dense_layer = torch.nn.Linear(4, 2)
output_layer = torch.nn.Softmax(1)model = torch.nn.Sequential(input_layer,
relu_layer,
dense_layer,
output_layer)
# print(model)# Create an instance of the pygad.torchga.TorchGA class to build the initial population.
torch_ga = torchga.TorchGA(model=model,
num_solutions=10)loss_function = torch.nn.BCELoss()
# XOR problem inputs
data_inputs = torch.tensor([[0.0, 0.0],
[0.0, 1.0],
[1.0, 0.0],
[1.0, 1.0]])# XOR problem outputs
data_outputs = torch.tensor([[1.0, 0.0],
[0.0, 1.0],
[0.0, 1.0],
[1.0, 0.0]])# Prepare the PyGAD parameters. Check the documentation for more information: https://pygad.readthedocs.io/en/latest/pygad.html#pygad-ga-class
num_generations = 250 # Number of generations.
num_parents_mating = 5 # Number of solutions to be selected as parents in the mating pool.
initial_population = torch_ga.population_weights # Initial population of network weights.# Create an instance of the pygad.GA class
ga_instance = pygad.GA(num_generations=num_generations,
num_parents_mating=num_parents_mating,
initial_population=initial_population,
fitness_func=fitness_func,
on_generation=callback_generation)# Start the genetic algorithm evolution.
ga_instance.run()# After the generations complete, some plots are showed that summarize how the outputs/fitness values evolve over generations.
ga_instance.plot_fitness(title="PyGAD & PyTorch - Iteration vs. Fitness", linewidth=4)# Returning the details of the best solution.
solution, solution_fitness, solution_idx = ga_instance.best_solution()
print("Fitness value of the best solution = {solution_fitness}".format(solution_fitness=solution_fitness))
print("Index of the best solution : {solution_idx}".format(solution_idx=solution_idx))# Fetch the parameters of the best solution.
best_solution_weights = torchga.model_weights_as_dict(model=model,
weights_vector=solution)
model.load_state_dict(best_solution_weights)
predictions = model(data_inputs)
print("Predictions : \n", predictions.detach().numpy())# Calculate the binary crossentropy for the trained model.
print("Binary Crossentropy : ", loss_function(predictions, data_outputs).detach().numpy())# Calculate the classification accuracy of the trained model.
a = torch.max(predictions, axis=1)
b = torch.max(data_outputs, axis=1)
accuracy = torch.sum(a.indices == b.indices) / len(data_outputs)
print("Accuracy : ", accuracy.detach().numpy())
```# For More Information
There are different resources that can be used to get started with the building CNN and its Python implementation.
## Tutorial: Implementing Genetic Algorithm in Python
To start with coding the genetic algorithm, you can check the tutorial titled [**Genetic Algorithm Implementation in Python**](https://www.linkedin.com/pulse/genetic-algorithm-implementation-python-ahmed-gad) available at these links:
- [LinkedIn](https://www.linkedin.com/pulse/genetic-algorithm-implementation-python-ahmed-gad)
- [Towards Data Science](https://towardsdatascience.com/genetic-algorithm-implementation-in-python-5ab67bb124a6)
- [KDnuggets](https://www.kdnuggets.com/2018/07/genetic-algorithm-implementation-python.html)[This tutorial](https://www.linkedin.com/pulse/genetic-algorithm-implementation-python-ahmed-gad) is prepared based on a previous version of the project but it still a good resource to start with coding the genetic algorithm.
[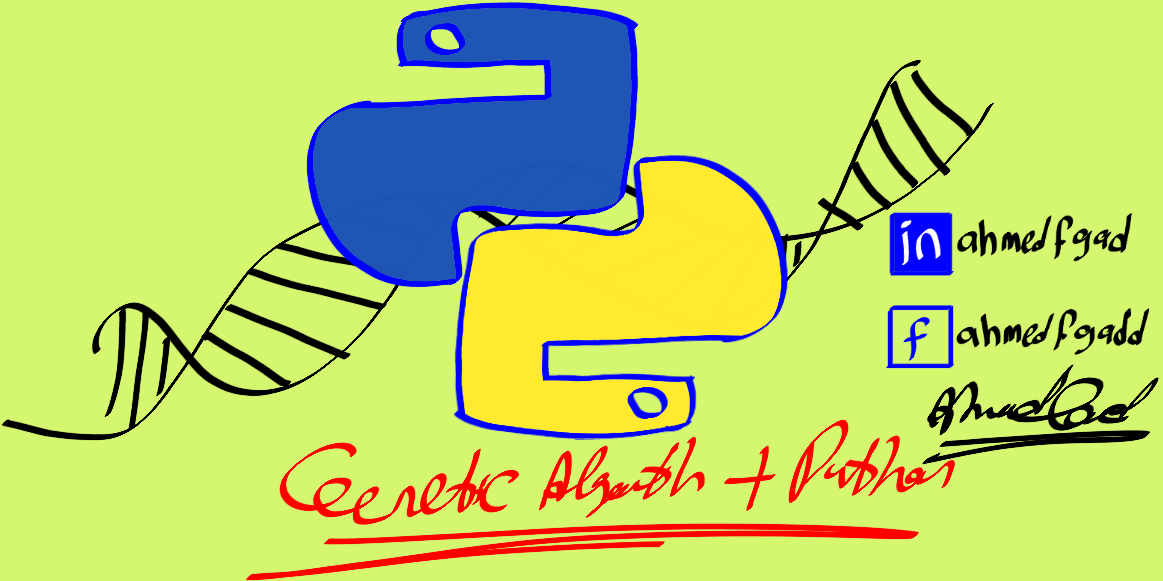](https://www.linkedin.com/pulse/genetic-algorithm-implementation-python-ahmed-gad)
## Tutorial: Introduction to Genetic Algorithm
Get started with the genetic algorithm by reading the tutorial titled [**Introduction to Optimization with Genetic Algorithm**](https://www.linkedin.com/pulse/introduction-optimization-genetic-algorithm-ahmed-gad) which is available at these links:
* [LinkedIn](https://www.linkedin.com/pulse/introduction-optimization-genetic-algorithm-ahmed-gad)
* [Towards Data Science](https://www.kdnuggets.com/2018/03/introduction-optimization-with-genetic-algorithm.html)
* [KDnuggets](https://towardsdatascience.com/introduction-to-optimization-with-genetic-algorithm-2f5001d9964b)[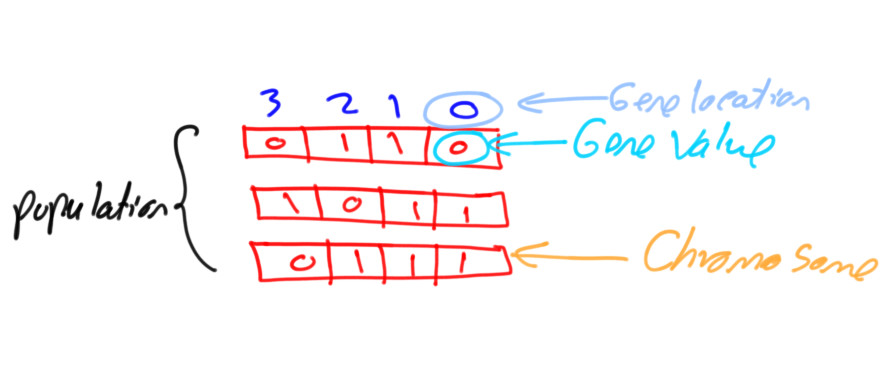](https://www.linkedin.com/pulse/introduction-optimization-genetic-algorithm-ahmed-gad)
## Tutorial: Build Neural Networks in Python
Read about building neural networks in Python through the tutorial titled [**Artificial Neural Network Implementation using NumPy and Classification of the Fruits360 Image Dataset**](https://www.linkedin.com/pulse/artificial-neural-network-implementation-using-numpy-fruits360-gad) available at these links:
* [LinkedIn](https://www.linkedin.com/pulse/artificial-neural-network-implementation-using-numpy-fruits360-gad)
* [Towards Data Science](https://towardsdatascience.com/artificial-neural-network-implementation-using-numpy-and-classification-of-the-fruits360-image-3c56affa4491)
* [KDnuggets](https://www.kdnuggets.com/2019/02/artificial-neural-network-implementation-using-numpy-and-image-classification.html)[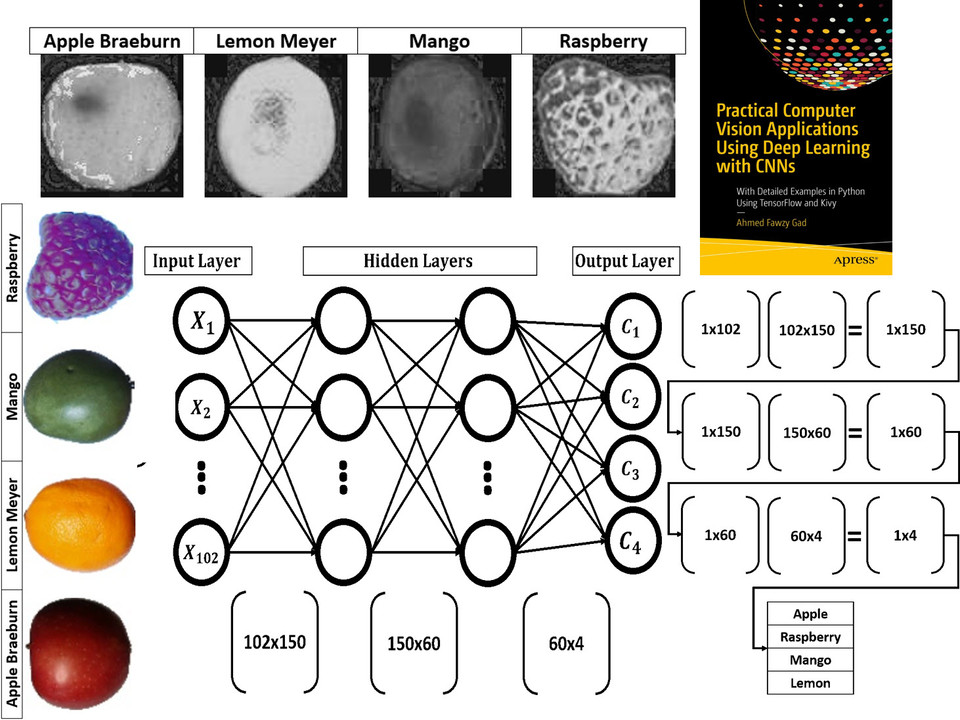](https://www.linkedin.com/pulse/artificial-neural-network-implementation-using-numpy-fruits360-gad)
## Tutorial: Optimize Neural Networks with Genetic Algorithm
Read about training neural networks using the genetic algorithm through the tutorial titled [**Artificial Neural Networks Optimization using Genetic Algorithm with Python**](https://www.linkedin.com/pulse/artificial-neural-networks-optimization-using-genetic-ahmed-gad) available at these links:
- [LinkedIn](https://www.linkedin.com/pulse/artificial-neural-networks-optimization-using-genetic-ahmed-gad)
- [Towards Data Science](https://towardsdatascience.com/artificial-neural-networks-optimization-using-genetic-algorithm-with-python-1fe8ed17733e)
- [KDnuggets](https://www.kdnuggets.com/2019/03/artificial-neural-networks-optimization-genetic-algorithm-python.html)[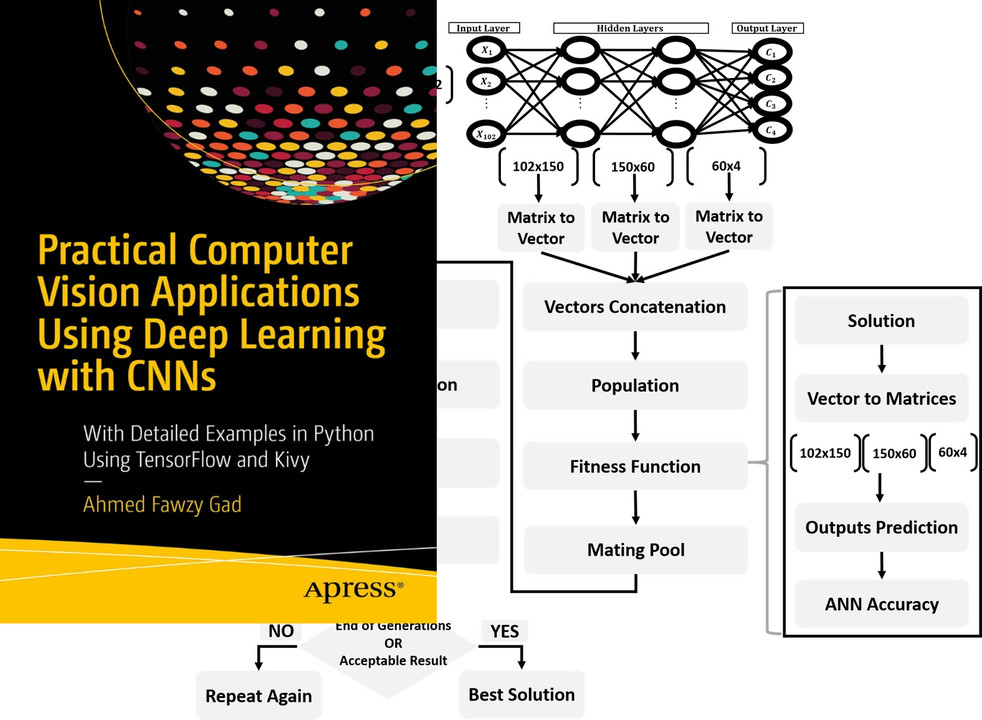](https://www.linkedin.com/pulse/artificial-neural-networks-optimization-using-genetic-ahmed-gad)
## Tutorial: Building CNN in Python
To start with coding the genetic algorithm, you can check the tutorial titled [**Building Convolutional Neural Network using NumPy from Scratch**](https://www.linkedin.com/pulse/building-convolutional-neural-network-using-numpy-from-ahmed-gad) available at these links:
- [LinkedIn](https://www.linkedin.com/pulse/building-convolutional-neural-network-using-numpy-from-ahmed-gad)
- [Towards Data Science](https://towardsdatascience.com/building-convolutional-neural-network-using-numpy-from-scratch-b30aac50e50a)
- [KDnuggets](https://www.kdnuggets.com/2018/04/building-convolutional-neural-network-numpy-scratch.html)
- [Chinese Translation](http://m.aliyun.com/yunqi/articles/585741)[This tutorial](https://www.linkedin.com/pulse/building-convolutional-neural-network-using-numpy-from-ahmed-gad)) is prepared based on a previous version of the project but it still a good resource to start with coding CNNs.
[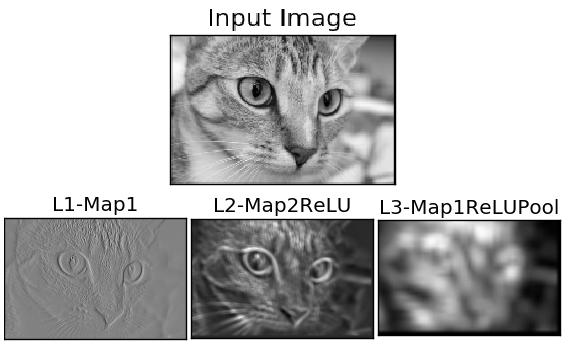](https://www.linkedin.com/pulse/building-convolutional-neural-network-using-numpy-from-ahmed-gad)
## Tutorial: Derivation of CNN from FCNN
Get started with the genetic algorithm by reading the tutorial titled [**Derivation of Convolutional Neural Network from Fully Connected Network Step-By-Step**](https://www.linkedin.com/pulse/derivation-convolutional-neural-network-from-fully-connected-gad) which is available at these links:
* [LinkedIn](https://www.linkedin.com/pulse/derivation-convolutional-neural-network-from-fully-connected-gad)
* [Towards Data Science](https://towardsdatascience.com/derivation-of-convolutional-neural-network-from-fully-connected-network-step-by-step-b42ebafa5275)
* [KDnuggets](https://www.kdnuggets.com/2018/04/derivation-convolutional-neural-network-fully-connected-step-by-step.html)[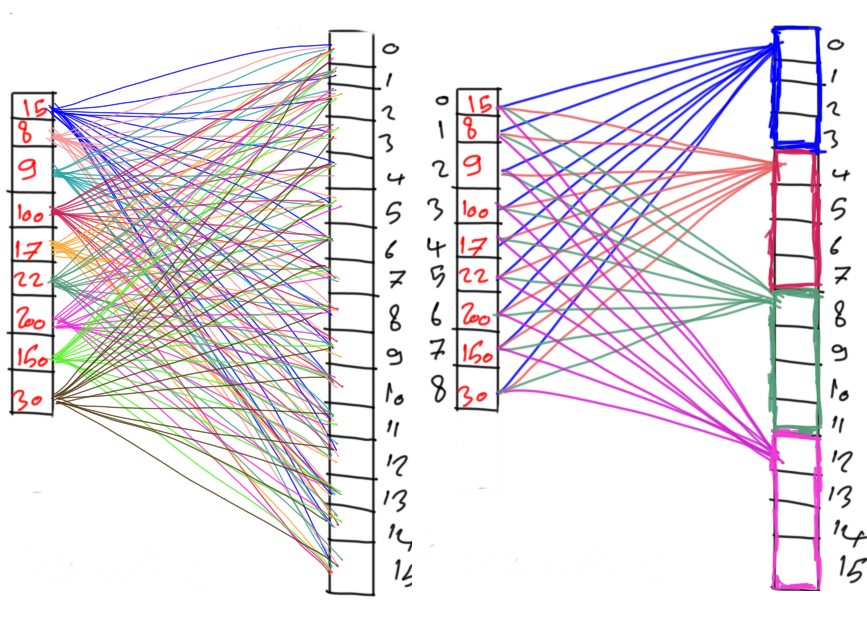](https://www.linkedin.com/pulse/derivation-convolutional-neural-network-from-fully-connected-gad)
## Book: Practical Computer Vision Applications Using Deep Learning with CNNs
You can also check my book cited as [**Ahmed Fawzy Gad 'Practical Computer Vision Applications Using Deep Learning with CNNs'. Dec. 2018, Apress, 978-1-4842-4167-7**](https://www.amazon.com/Practical-Computer-Vision-Applications-Learning/dp/1484241665) which discusses neural networks, convolutional neural networks, deep learning, genetic algorithm, and more.
Find the book at these links:
- [Amazon](https://www.amazon.com/Practical-Computer-Vision-Applications-Learning/dp/1484241665)
- [Springer](https://link.springer.com/book/10.1007/978-1-4842-4167-7)
- [Apress](https://www.apress.com/gp/book/9781484241660)
- [O'Reilly](https://www.oreilly.com/library/view/practical-computer-vision/9781484241677)
- [Google Books](https://books.google.com.eg/books?id=xLd9DwAAQBAJ)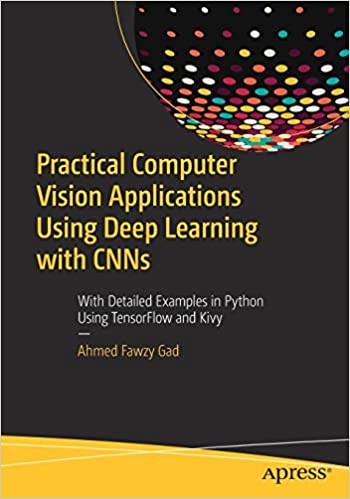
# Citing PyGAD - Bibtex Formatted Citation
If you used PyGAD, please consider adding a citation to the following paper about PyGAD:
```
@misc{gad2021pygad,
title={PyGAD: An Intuitive Genetic Algorithm Python Library},
author={Ahmed Fawzy Gad},
year={2021},
eprint={2106.06158},
archivePrefix={arXiv},
primaryClass={cs.NE}
}
```# Contact Us
* E-mail: [email protected]
* [LinkedIn](https://www.linkedin.com/in/ahmedfgad)
* [Amazon Author Page](https://amazon.com/author/ahmedgad)
* [Heartbeat](https://heartbeat.fritz.ai/@ahmedfgad)
* [Paperspace](https://blog.paperspace.com/author/ahmed)
* [KDnuggets](https://kdnuggets.com/author/ahmed-gad)
* [TowardsDataScience](https://towardsdatascience.com/@ahmedfgad)
* [GitHub](https://github.com/ahmedfgad)