Ecosyste.ms: Awesome
An open API service indexing awesome lists of open source software.
https://github.com/antononcube/raku-llm-functions
Raku package for functions and function objects to access, interact, and utilize LLMs.
https://github.com/antononcube/raku-llm-functions
chatgpt functional-programming large-language-models llm openai palm-api raku web-api webapi
Last synced: about 2 months ago
JSON representation
Raku package for functions and function objects to access, interact, and utilize LLMs.
- Host: GitHub
- URL: https://github.com/antononcube/raku-llm-functions
- Owner: antononcube
- License: artistic-2.0
- Created: 2023-07-18T14:06:11.000Z (over 1 year ago)
- Default Branch: main
- Last Pushed: 2024-09-08T13:37:11.000Z (3 months ago)
- Last Synced: 2024-10-11T20:41:41.376Z (2 months ago)
- Topics: chatgpt, functional-programming, large-language-models, llm, openai, palm-api, raku, web-api, webapi
- Language: Raku
- Homepage: https://raku.land/zef:antononcube/LLM::Functions
- Size: 645 KB
- Stars: 0
- Watchers: 2
- Forks: 0
- Open Issues: 0
-
Metadata Files:
- Readme: README-work.md
- License: LICENSE
Awesome Lists containing this project
README
# LLM::Functions
[](https://github.com/antononcube/Raku-LLM-Functions/actions/workflows/macos.yml)
[](https://github.com/antononcube/Raku-LLM-Functions/actions/workflows/linux.yml)
[](https://github.com/antononcube/Raku-LLM-Functions/actions/workflows/windows.yml)
[](https://raku.land/zef:antononcube/LLM::Functions)## In brief
This Raku package provides functions and function objects to access, interact, and utilize
Large Language Models (LLMs), like
[OpenAI](https://platform.openai.com), [OAI1],
[PaLM](https://developers.generativeai.google/products/palm), [ZG1],
and
[MistralAI](https://docs.mistral.ai), [MAI1].For more details how the concrete LLMs are accessed see the packages
["WWW::OpenAI"](https://raku.land/zef:antononcube/WWW::OpenAI), [AAp2],
["WWW::PaLM"](https://raku.land/zef:antononcube/WWW::PaLM), [AAp3],
["WWW::MistralAI"](https://raku.land/zef:antononcube/WWW::MistralAI), [AAp9], and
["WWW::Gemini"](https://raku.land/zef:antononcube/WWW::Gemini), [AAp11].The LLM functions built by this package can have evaluators that use "sub-parsers" -- see
["Text::SubParsers"](https://raku.land/zef:antononcube/Text::SubParsers), [AAp4].The primary motivation to have handy, configurable functions for utilizing LLMs
came from my work on the packages
["ML::FindTextualAnswer"](https://raku.land/zef:antononcube/ML::FindTextualAnswer), [AAp6], and
["ML::NLPTemplateEngine"](https://raku.land/zef:antononcube/ML::NLPTemplateEngine), [AAp7].A very similar system of functionalities is developed by Wolfram Research Inc.;
see the paclet
["LLMFunctions"](https://resources.wolframcloud.com/PacletRepository/resources/Wolfram/LLMFunctions/), [WRIp1].For well curated and instructive examples of LLM prompts see the
[Wolfram Prompt Repository](https://resources.wolframcloud.com/PromptRepository/).
Many of those prompts (≈220) are available in Raku and Python --
see ["LLM::Prompts"](https://raku.land/zef:antononcube/LLM::Prompts), [AAp8], and
["LLMPrompts"](https://pypi.org/project/LLMPrompts/), [AAp10], respectively.The article
["Generating documents via templates and LLMs"](https://rakuforprediction.wordpress.com/2023/07/11/generating-documents-via-templates-and-llms/), [AA1],
shows an alternative way of streamlining LLMs usage. (Via Markdown, Org-mode, or Pod6 templates.)-----
## Installation
Package installations from both sources use [zef installer](https://github.com/ugexe/zef)
(which should be bundled with the "standard" Rakudo installation file.)To install the package from [Zef ecosystem](https://raku.land/) use the shell command:
```
zef install LLM::Functions
```To install the package from the GitHub repository use the shell command:
```
zef install https://github.com/antononcube/Raku-LLM-Functions.git
```------
## Design
"Out of the box"
["LLM::Functions"](https://raku.land/zef:antononcube/LLM::Functions) uses
["WWW::OpenAI"](https://raku.land/zef:antononcube/WWW::OpenAI), [AAp2],
["WWW::PaLM"](https://raku.land/zef:antononcube/WWW::PaLM), [AAp3], and
["WWW::MistralAI"](https://raku.land/zef:antononcube/WWW::MistralAI), [AAp9].
Other LLM access packages can be utilized via appropriate LLM configurations.Configurations:
- Are instances of the class `LLM::Functions::Configuration`
- Are used by instances of the class `LLM::Functions::Evaluator`
- Can be converted to Hash objects (i.e. have a `.Hash` method)New LLM functions are constructed with the function `llm-function`.
The function `llm-function`:
- Has the option "llm-evaluator" that takes evaluators, configurations, or string shorthands as values
- Returns anonymous functions (that access LLMs via evaluators/configurations.)
- Gives result functions that can be applied to different types of arguments depending on the first argument
- Can take a (sub-)parser argument for post-processing of LLM results
- Takes as a first argument a prompt that can be a:
- String
- Function with positional arguments
- Function with named argumentsHere is a sequence diagram that follows the steps of a typical creation procedure of
LLM configuration- and evaluator objects, and the corresponding LLM-function that utilizes them:```mermaid
sequenceDiagram
participant User
participant llmfunc as llm-function
participant llmconf as llm-configuration
participant LLMConf as LLM configuration
participant LLMEval as LLM evaluator
participant AnonFunc as Anonymous function
User ->> llmfunc: ・prompt
・conf spec
llmfunc ->> llmconf: conf spec
llmconf ->> LLMConf: conf spec
LLMConf ->> LLMEval: wrap with
LLMEval ->> llmfunc: evaluator object
llmfunc ->> AnonFunc: create with:
・evaluator object
・prompt
AnonFunc ->> llmfunc: handle
llmfunc ->> User: handle
```Here is a sequence diagram for making a LLM configuration with a global (engineered) prompt,
and using that configuration to generate a chat message response:```mermaid
sequenceDiagram
participant WWWOpenAI as WWW::OpenAI
participant User
participant llmfunc as llm-function
participant llmconf as llm-configuration
participant LLMConf as LLM configuration
participant LLMChatEval as LLM chat evaluator
participant AnonFunc as Anonymous function
User ->> llmconf: engineered prompt
llmconf ->> User: configuration object
User ->> llmfunc: ・prompt
・configuration object
llmfunc ->> LLMChatEval: configuration object
LLMChatEval ->> llmfunc: evaluator object
llmfunc ->> AnonFunc: create with:
・evaluator object
・prompt
AnonFunc ->> llmfunc: handle
llmfunc ->> User: handle
User ->> AnonFunc: invoke with
message argument
AnonFunc ->> WWWOpenAI: ・engineered prompt
・message
WWWOpenAI ->> User: LLM response
```------
## Configurations
### OpenAI-based
Here is the default, OpenAI-based configuration:
```perl6
use LLM::Functions;
.raku.say for llm-configuration('OpenAI').Hash;
```Here is the ChatGPT-based configuration:
```perl6
.say for llm-configuration('ChatGPT').Hash;
```**Remark:** `llm-configuration(Whatever)` is equivalent to `llm-configuration('OpenAI')`.
**Remark:** Both the "OpenAI" and "ChatGPT" configuration use functions of the package "WWW::OpenAI", [AAp2].
The "OpenAI" configuration is for text-completions;
the "ChatGPT" configuration is for chat-completions.### PaLM-based
Here is the default PaLM configuration:
```perl6
.say for llm-configuration('PaLM').Hash;
```-----
## Basic usage of LLM functions
### Textual prompts
Here we make a LLM function with a simple (short, textual) prompt:
```perl6
my &func = llm-function('Show a recipe for:');
```Here we evaluate over a message:
```perl6
say &func('greek salad');
```### Positional arguments
Here we make a LLM function with a function-prompt and numeric interpreter of the result:
```perl6
my &func2 = llm-function(
{"How many $^a can fit inside one $^b?"},
form => Numeric,
llm-evaluator => 'palm');
```Here were we apply the function:
```perl6
my $res2 = &func2("tennis balls", "toyota corolla 2010");
```Here we show that we got a number:
```perl6
$res2 ~~ Numeric
```### Named arguments
Here the first argument is a template with two named arguments:
```perl6
my &func3 = llm-function(-> :$dish, :$cuisine {"Give a recipe for $dish in the $cuisine cuisine."}, llm-evaluator => 'palm');
```Here is an invocation:
```perl6
&func3(dish => 'salad', cuisine => 'Russian', max-tokens => 300);
```--------
## LLM example functions
The function `llm-example-function` can be given a training set of examples in order
to generating results according to the "laws" implied by that training set.Here a LLM is asked to produce a generalization:
```perl6
llm-example-function([ 'finger' => 'hand', 'hand' => 'arm' ])('foot')
```Here is an array of training pairs is used:
```perl6
'Oppenheimer' ==> (["Einstein" => "14 March 1879", "Pauli" => "April 25, 1900"] ==> llm-example-function)()
```Here is defined a LLM function for translating WL associations into Python dictionaries:
```perl6
my &fea = llm-example-function( '<| A->3, 4->K1 |>' => '{ A:3, 4:K1 }');
&fea('<| 23->3, G->33, T -> R5|>');
```The function `llm-example-function` takes as a first argument:
- Single `Pair` object of two scalars
- Single `Pair` object of two `Positional` objects with the same length
- A `Hash`
- A `Positional` object of pairs**Remark:** The function `llm-example-function` is implemented with `llm-function` and suitable prompt.
Here is an example of using hints:
```perl6
my &fec = llm-example-function(
["crocodile" => "grasshopper", "fox" => "cardinal"],
hint => 'animal colors');say &fec('raccoon');
```--------
## Using predefined prompts
Using predefined prompts of the package ["LLM::Prompts"](https://raku.land/zef:antononcube/LLM::Prompts), [AAp8],
can be very convenient in certain (many) cases.Here is an example using "Fixed That For You" synthesis:
```perl6
use LLM::Prompts;llm-synthesize([llm-prompt('FTFY'), 'Wha is ther population?'])
```--------
## Using chat-global prompts
The configuration objects can be given prompts that influence the LLM responses
"globally" throughout the whole chat. (See the second sequence diagram above.)For detailed examples see the documents:
- ["Using engineered prompts"](./docs/Using-engineered-prompts_woven.md)
- ["Expand tests into documentation examples"](./docs/Expand-tests-into-doc-examples_woven.md)--------
## Chat objects
Here we create chat object that uses OpenAI's ChatGPT:
```perl6
my $prompt = 'You are a gem expert and you give concise answers.';
my $chat = llm-chat(chat-id => 'gem-expert-talk', conf => 'ChatGPT', :$prompt);
``````perl6
$chat.eval('What is the most transparent gem?');
``````perl6
$chat.eval('Ok. What are the second and third most transparent gems?');
```Here are the prompt(s) and all messages of the chat object:
```perl6
$chat.say
```--------
## AI-vision functions
Consider [this image](https://raw.githubusercontent.com/antononcube/MathematicaForPrediction/master/MarkdownDocuments/Diagrams/AI-vision-via-WL/0iyello2xfyfo.png):
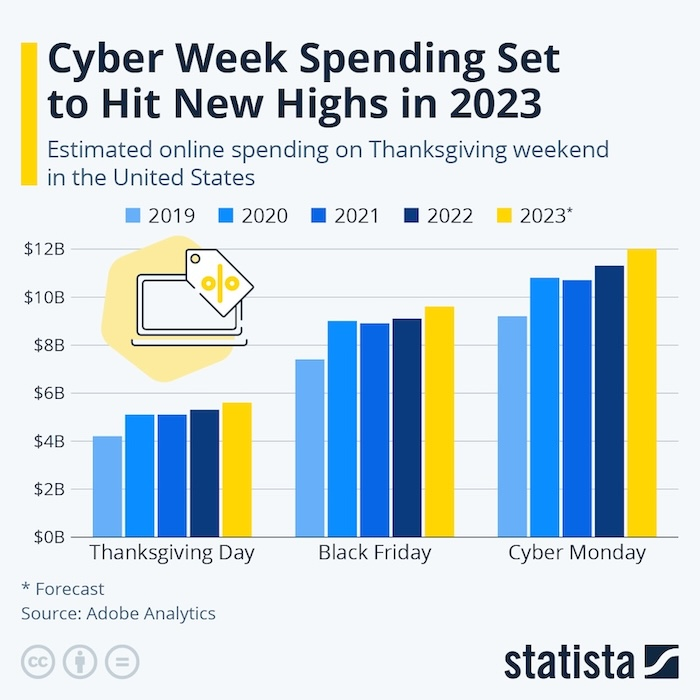
Here we import the image (as a Base64 string):
```perl6
use Image::Markup::Utilities;
my $url = 'https://raw.githubusercontent.com/antononcube/MathematicaForPrediction/master/MarkdownDocuments/Diagrams/AI-vision-via-WL/0iyello2xfyfo.png';
my $img = image-import($url);
$img.substr(^100)
```Here we apply OpenAI's AI vision model `gpt-4-vision-preview` (which is the default one) over the ***URL of the image***:
```perl6
llm-vision-synthesize('Describe the image.', $url);
```Here we apply Gemini's AI vision model `gemini-pro-vision` over the image:
```perl6
llm-vision-synthesize('Describe the image.', $img, e => 'Gemini');
```**Remark:** Currently, Gemini works with (Base64) images only (and does not with URLs.) OpenAI's vision works with both URLs and images.
The function `llm-vision-function` uses the same evaluators (configurations, models) as `llm-vision-synthesize`.
--------
## Potential problems
With PaLM with certain wrong configuration we get the error:
```
error => {code => 400, message => Messages must alternate between authors., status => INVALID_ARGUMENT}
```--------
## TODO
- [X] DONE Resources
- See ["LLM::Prompts"](https://github.com/antononcube/Raku-LLM-Prompts)
- [X] DONE Gather prompts
- [X] DONE Process prompts into a suitable database
- Using JSON.
- [ ] TODO Implementation
- [X] DONE Processing and array of prompts as a first argument
- [X] DONE Prompt class / object / record
- Again, see ["LLM::Prompts"](https://github.com/antononcube/Raku-LLM-Prompts)
- For retrieval and management of prompts.
- [X] DONE Prompts can be both plain strings or templates / functions.
- [X] DONE Each prompt has associated metadata:
- Type: persona, function, modifier
- Tool/parser
- Keywords
- Contributor?
- Topics: "Advisor bot", "AI Guidance", "For Fun", ...
- See: https://resources.wolframcloud.com/PromptRepository/
- [X] DONE Most likely, there would be a separate package "LLM::Prompts", [AAp8].
- [ ] MAYBE Random selection of LLM-evaluator
- Currently, the LLM-evaluator of the LLM-functions and LLM-chats is static, assigned at creation.
- This is easily implemented at "top-level."
- [X] DONE Chat class / object
- For long conversations
- [X] DONE Include LLaMA
- Just using a different `:$base-url` for "ChatGPT" for the configurations.
- [X] DONE Include Gemini
- [X] DONE Separate configuration
- [X] DONE Its own evaluator class
- [X] DONE LLM example function
- [X] DONE First version with the signatures:
- [X] `@pairs`
- [X] `@input => @output`
- [X] Hint option
- [X] DONE Verify works with OpenAI
- [X] DONE Verify works with PaLM
- [X] DONE Verify works with Gemini
- [X] DONE Interpreter argument for `llm-function`
- See the `formatron` attribute of `LLM::Functions::Evaluator`.
- [X] DONE Adding `form` option to chat objects evaluator
- [X] DONE Implement `llm-embedding` function
- Generic, universal function for accessing the embeddings of different providers/models.
- [ ] TODO CLI
- [ ] TODO Based on Chat objects
- [ ] TODO Storage and retrieval of chats
- [ ] TODO Has as parameters all attributes of the LLM-configuration objects.
- [ ] TODO Documentation
- [ ] TODO Detailed parameters description
- [ ] TODO Configuration
- [ ] TODO Evaluator
- [ ] TODO Chat
- [X] DONE Using engineered prompts
- [X] DONE Expand tests in documentation examples
- [X] DONE Conversion of a test file tests into Gherkin specs
- [X] DONE Number game programming
- [X] DONE Man vs Machine
- [X] DONE Machine vs Machine
- [X] DONE Using retrieved prompts
- [ ] TODO Longer conversations / chats--------
## References
### Articles
[AA1] Anton Antonov,
["Generating documents via templates and LLMs"](https://rakuforprediction.wordpress.com/2023/07/11/generating-documents-via-templates-and-llms/),
(2023),
[RakuForPrediction at WordPress](https://rakuforprediction.wordpress.com).[ZG1] Zoubin Ghahramani,
["Introducing PaLM 2"](https://blog.google/technology/ai/google-palm-2-ai-large-language-model/),
(2023),
[Google Official Blog on AI](https://blog.google/technology/ai/).### Repositories, sites
[MAI1] MistralAI team, [MistralAI platform](https://docs.mistral.ai).
[OAI1] OpenAI team, [OpenAI platform](https://platform.openai.com/).
[WRIr1] Wolfram Research, Inc.
[Wolfram Prompt Repository](https://resources.wolframcloud.com/PromptRepository/).### Packages, paclets
[AAp1] Anton Antonov,
[LLM::Functions Raku package](https://github.com/antononcube/Raku-LLM-Functions),
(2023),
[GitHub/antononcube](https://github.com/antononcube).[AAp2] Anton Antonov,
[WWW::OpenAI Raku package](https://github.com/antononcube/Raku-WWW-OpenAI),
(2023),
[GitHub/antononcube](https://github.com/antononcube).[AAp3] Anton Antonov,
[WWW::PaLM Raku package](https://github.com/antononcube/Raku-WWW-PaLM),
(2023),
[GitHub/antononcube](https://github.com/antononcube).[AAp4] Anton Antonov,
[Text::SubParsers Raku package](https://github.com/antononcube/Raku-Text-SubParsers),
(2023),
[GitHub/antononcube](https://github.com/antononcube).[AAp5] Anton Antonov,
[Text::CodeProcessing Raku package](https://github.com/antononcube/Raku-Text-CodeProcessing),
(2021),
[GitHub/antononcube](https://github.com/antononcube).[AAp6] Anton Antonov,
[ML::FindTextualAnswer Raku package](https://github.com/antononcube/Raku-ML-FindTextualAnswer),
(2023),
[GitHub/antononcube](https://github.com/antononcube).[AAp7] Anton Antonov,
[ML::NLPTemplateEngine Raku package](https://github.com/antononcube/Raku-ML-NLPTemplateEngine),
(2023),
[GitHub/antononcube](https://github.com/antononcube).[AAp8] Anton Antonov,
[LLM::Prompts Raku package](https://github.com/antononcube/Raku-LLM-Prompts),
(2023),
[GitHub/antononcube](https://github.com/antononcube).[AAp9] Anton Antonov,
[WWW::MistralAI Raku package](https://github.com/antononcube/Raku-WWW-MistralAI),
(2023),
[GitHub/antononcube](https://github.com/antononcube).[AAp10] Anton Antonov,
[LLMPrompts Python package](https://pypi.org/project/LLMPrompts/),
(2023),
[PyPI.org/antononcube](https://pypi.org/user/antononcube/).[AAp11] Anton Antonov,
[WWW::Gemini Raku package](https://github.com/antononcube/Raku-WWW-Gemini),
(2024),
[GitHub/antononcube](https://github.com/antononcube).[WRIp1] Wolfram Research, Inc.
[LLMFunctions paclet](https://resources.wolframcloud.com/PacletRepository/resources/Wolfram/LLMFunctions/),
(2023),
[Wolfram Language Paclet Repository](https://resources.wolframcloud.com/PacletRepository/).