https://github.com/bbuf/how-to-optim-algorithm-in-cuda
how to optimize some algorithm in cuda.
https://github.com/bbuf/how-to-optim-algorithm-in-cuda
cuda llm
Last synced: about 2 months ago
JSON representation
how to optimize some algorithm in cuda.
- Host: GitHub
- URL: https://github.com/bbuf/how-to-optim-algorithm-in-cuda
- Owner: BBuf
- Created: 2018-07-18T07:55:40.000Z (almost 7 years ago)
- Default Branch: master
- Last Pushed: 2024-10-29T15:01:34.000Z (8 months ago)
- Last Synced: 2024-10-29T15:57:14.542Z (8 months ago)
- Topics: cuda, llm
- Language: Cuda
- Homepage:
- Size: 83.3 MB
- Stars: 1,550
- Watchers: 26
- Forks: 128
- Open Issues: 3
-
Metadata Files:
- Readme: README.md
Awesome Lists containing this project
README
## how-to-optim-algorithm-in-cuda
> 我也维护了一个学习深度学习框架(PyTorch和OneFlow)的仓库 https://github.com/BBuf/how-to-learn-deep-learning-framework 以及一个如何学习深度学习编译器(TVM/MLIR/LLVM)的学习仓库 https://github.com/BBuf/tvm_mlir_learn , 有需要的小伙伴可以**点一点star**
本工程记录如何基于 cuda 优化一些常见的算法。请注意,下面的介绍都分别对应了子目录的代码实现,所以想复现性能的话请查看对应子目录下面的 README 。
> 友情链接:https://github.com/DefTruth/CUDA-Learn-Notes
### 0. **cuda-mode**
- 课程的 Slides 和 脚本:https://github.com/cuda-mode/lectures
- 课程地址:https://www.youtube.com/@CUDAMODE
- 我的课程笔记:https://github.com/BBuf/how-to-optim-algorithm-in-cuda/tree/master/cuda-mode一直想系统看一下某个课程系统和科学的学习下 CUDA ,感觉 CUDA-MODE 这个课程能满足我的需求。这个课程是几个 PyTorch 的 Core Dev 搞的,比较系统和专业。不过由于这个课程是 Youtube 上的英语课程,所以要学习和理解这个课程还是需要花不少时间的,我这里记录一下学习这个课程的每一课的笔记,希望可以通过这个笔记帮助对这个课程以及 CUDA 感兴趣的读者更快吸收这个课程的知识。这个课程相比于以前的纯教程更加关注的是我们可以利用 CUDA 做什么事情,而不是让读者陷入到 CUDA 专业术语的细节中,那会非常痛苦。伟大无需多言,感兴趣请阅读本文件夹下的各个课程的学习笔记。
### 1. how-to-compile-pytorch-from-source
记录如何手动编译 PyTorch 源码,学习 PyTorch 的一些 cuda 实现。
### 2. reduce
这里记录学习 NIVDIA 的[reduce优化官方博客](https://developer.download.nvidia.com/assets/cuda/files/reduction.pdf) 做的笔记。完整实验代码见[这里](https://github.com/BBuf/how-to-optim-algorithm-in-cuda/tree/master/reduce) , 原理讲解请看:[【BBuf的CUDA笔记】三,reduce优化入门学习笔记](https://zhuanlan.zhihu.com/p/596012674) 。后续又添加了 PyTorch BlockReduce 模板以及在这个模板的基础上额外加了一个数据 Pack ,又获得了一些带宽的提升。详细数据如下:
性能和带宽的测试情况如下 (A100 PCIE 40G):
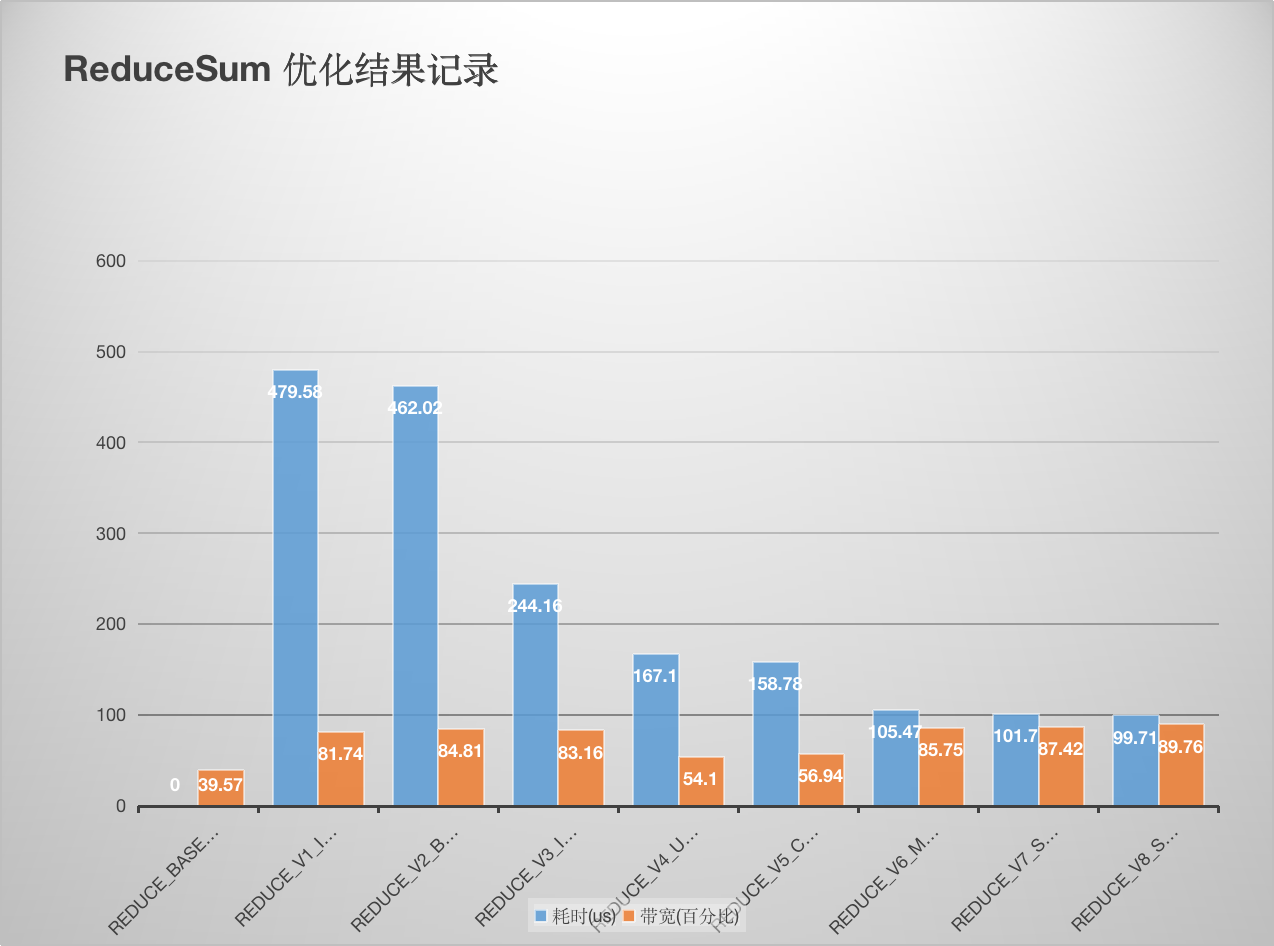
### 3. elementwise
将 oneflow 的 elementwise 模板抽出来方便大家使用,这个 elementwise 模板实现了高效的性能和带宽利用率,并且用法非常灵活。完整实验代码见[这里](https://github.com/BBuf/how-to-optim-algorithm-in-cuda/blob/master/elementwise/elementwise.cu) ,原理讲解请看:[【BBuf 的CUDA笔记】一,解析OneFlow Element-Wise 算子实现](https://zhuanlan.zhihu.com/p/591058808) 。这里以逐点乘为例,性能和带宽的测试情况如下 (A100 PCIE 40G):
|优化手段|数据类型|耗时(us)|带宽利用率|
|--|--|--|--|
|naive elementwise|float|298.46us|85.88%|
|oneflow elementwise|float|284us|89.42%|
|naive elementwise|half|237.28us|52.55%|
|oneflow elementwise|half|140.74us|87.31%|可以看到无论是性能还是带宽,使用 oneflow 的 elementwise 模板相比于原始实现都有较大提升。
### 4. FastAtomicAdd
实现的脚本是针对half数据类型做向量的内积,用到了atomicAdd,保证数据的长度以及gridsize和blocksize都是完全一致的。一共实现了3个脚本:
1. https://github.com/BBuf/how-to-optim-algorithm-in-cuda/blob/master/FastAtomicAdd/atomic_add_half.cu 纯half类型的atomicAdd。
2. https://github.com/BBuf/how-to-optim-algorithm-in-cuda/blob/master/FastAtomicAdd/atomic_add_half_pack2.cu half+pack,最终使用的是half2类型的atomicAdd。
3. https://github.com/BBuf/how-to-optim-algorithm-in-cuda/blob/master/FastAtomicAdd/fast_atomic_add_half.cu 快速原子加,虽然没有显示的pack,但本质上也是通过对单个half补0使用上了half2的原子加。性能和带宽的测试情况如下 (A100 PCIE 40G):
|原子加方式|性能(us)|
|--|--|
|纯half类型|422.36ms|
|pack half2类型|137.02ms|
|fastAtomicAdd|137.01ms|可以看到使用pack half的方式和直接使用half的fastAtomicAdd方式得到的性能结果一致,均比原始的half的原子加快3-4倍。
### 5. UpsampleNearest2D
upsample_nearest_2d.cu 展示了 oneflow 对 upsample_nearest2d 的前后向的优化 kernel 的用法,性能和带宽的测试情况如下 (A100 PCIE 40G):
|框架|数据类型|Op类型|带宽利用率|耗时|
|--|--|--|--|--|
| PyTorch | Float32 | UpsampleNearest2D forward | 28.30% | 111.42us |
| PyTorch | Float32 | UpsampleNearest2D backward | 60.16% | 65.12us |
| OneFlow | Float32 |UpsampleNearest2D forward | 52.18% | 61.44us |
| OneFlow | Float32 |UpsampleNearest2D backward | 77.66% | 50.56us |
| PyTorch | Float16 | UpsampleNearest2D forward | 16.99% | 100.38us |
| PyTorch | Float16 | UpsampleNearest2D backward | 31.56% | 57.38us |
| OneFlow | Float16 |UpsampleNearest2D forward | 43.26% | 35.36us |
| OneFlow | Float16 |UpsampleNearest2D backward | 44.82% | 40.26us |可以看到基于 oneflow upsample_nearest2d 的前后向的优化 kernel 可以获得更好的带宽利用率和性能。注意这里的 profile 使用的是 oneflow 脚本,而不是 upsample_nearest_2d.cu ,详情请看 [UpsampleNearest2D/README.md](UpsampleNearest2D/README.md) 。
### 6. indexing
在 PyTorch 中对 index_add 做了极致的优化,我这里将 [PyTorch 的 index_add 实现](indexing/index_add_cuda_pytorch_impl.cu) 进行了剥离,方便大家应用于其它框架。具体请看 indexing 文件夹的 README 。其中还有和 oneflow 的 index_add 实现的各个 case 的性能比较结果。整体来说 PyTorch 在 index Tensor元素很小,但Tensor很大的情况下有较大的性能提升,其它情况和 OneFlow 基本持平。详情请看 [indexing/README.md](indexing/README.md) 。
### 7. oneflow-cuda-optimize-skills
OneFlow 深度学习框架中基于 cuda 做的优化工作,动态更新中。
### 8. FastTransformer
总结 FastTransformer 相关的 cuda 优化技巧。[README_BERT.md](FastTransformer/README_BERT.md) 总结了 BERT 相关的优化技巧。
### 9. softmax
学习了oneflow的softmax kernel实现以及Faster Transformer softmax kernel的实现,并以个人的角度分别解析了原理和代码实现,最后对性能做一个对比方便大家直观的感受到oneflow softmax kernel相比于FasterTransformer的优越性。
### 10. linear-attention
学习一些 linear attention 的 cuda 优化技巧。
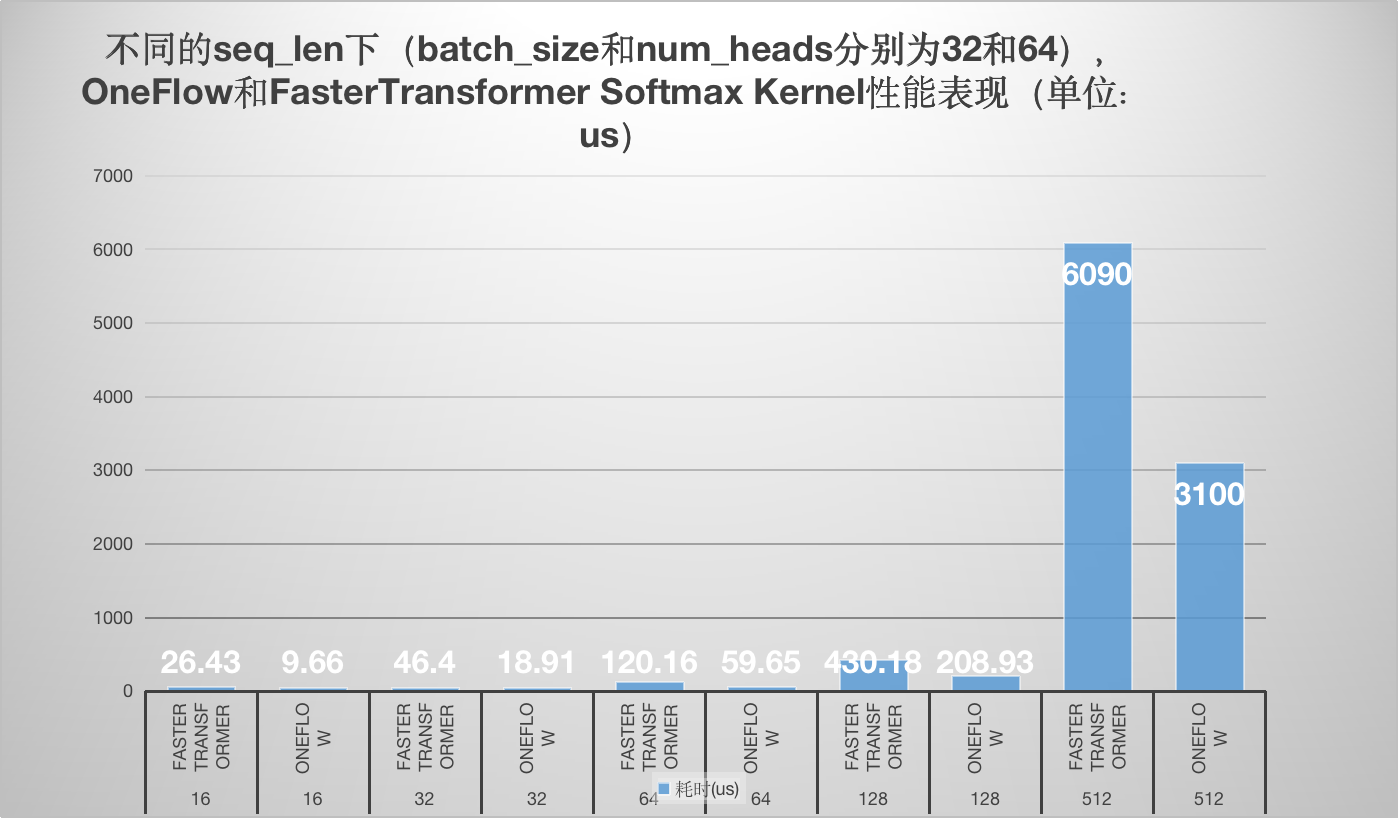
### 11. large-language-model-note
收集了和大语言模型原理,训练,推理,数据标注的相关文章。
### 12. mlsys-paper
前研的大模型训练相关 AI-Infra 论文收集以及阅读笔记。
### 13. triton
Triton 学习过程中的代码记录和学习笔记。
### 14. meagtron-lm
Meagtron-LM 学习笔记。
### 15. triton-meetup
Triton 中国举办的 Meetup 的slides汇总。点卡这个文件夹也可以找到对应的Meetup的视频回放。
### 16. ptx-isa
对 CUDA PTX ISA 文档的一个翻译和学习。
### 17. pytorch-blog-codes
对 PyTorch 团队发布的 cuda 技术的一些学习笔记。
### 18. cutlass
cutlass 相关的学习笔记。
### 19. cuda-paper
cuda 相关的 paper 的阅读。
### 20. 原创学习笔记
点击展开/收起 BBuf 的 CUDA 学习笔记列表
- [【BBuf的CUDA笔记】一,解析OneFlow Element-Wise 算子实现](https://zhuanlan.zhihu.com/p/591058808)
- [【BBuf的CUDA笔记】二,解析 OneFlow BatchNorm 相关算子实现](https://zhuanlan.zhihu.com/p/593483751)
- [【BBuf的CUDA笔记】三,reduce优化入门学习笔记](https://zhuanlan.zhihu.com/p/596012674)
- [【BBuf的CUDA笔记】四,介绍三个高效实用的CUDA算法实现(OneFlow ElementWise模板,FastAtomicAdd模板,OneFlow UpsampleNearest2d模板)](https://zhuanlan.zhihu.com/p/597435971)
- [【BBuf的CUDA笔记】五,解读 PyTorch index_add 操作涉及的优化技术](https://zhuanlan.zhihu.com/p/599085070)
- [【BBuf的CUDA笔记】六,总结 FasterTransformer Encoder(BERT) 的cuda相关优化技巧](https://zhuanlan.zhihu.com/p/601130731)
- [【BBuf的CUDA笔记】七,总结 FasterTransformer Decoder(GPT) 的cuda相关优化技巧](https://zhuanlan.zhihu.com/p/603611192)
- [【BBuf的CUDA笔记】八,对比学习OneFlow 和 FasterTransformer 的 Softmax Cuda实现](https://zhuanlan.zhihu.com/p/609198294)
- [【BBuf的CUDA笔记】九,使用newbing(chatgpt)解析oneflow softmax相关的fuse优化](https://zhuanlan.zhihu.com/p/615619524)
- [CodeGeeX百亿参数大模型的调优笔记:比FasterTransformer更快的解决方案](https://zhuanlan.zhihu.com/p/617027615)
- [【BBuf的cuda学习笔记十】Megatron-LM的gradient_accumulation_fusion优化](https://mp.weixin.qq.com/s/neP8faIXIvj-XlyFjXjWBg)
- [【BBuf的CUDA笔记】十,Linear Attention的cuda kernel实现解析](https://mp.weixin.qq.com/s/1EPeU5hsOhB7rNAmmXrZRw)
- [【BBuf的CUDA笔记】十一,Linear Attention的cuda kernel实现补档](https://mp.weixin.qq.com/s/qDVKclf_AvpZ5qb2Obf4aA)
- [【BBuf的CUDA笔记】十二,LayerNorm/RMSNorm的重计算实现](https://mp.weixin.qq.com/s/G_XvnB4CeEBWTLNefi0Riw)
- [【BBuf的CUDA笔记】十三,OpenAI Triton 入门笔记一](https://mp.weixin.qq.com/s/RMR_n1n6nBqpdMl6tdd7pQ)
- [【BBuf的CUDA笔记】十四,OpenAI Triton入门笔记二](https://mp.weixin.qq.com/s/ZjADeYg5LCyGaLx0chpSZw)
- [【BBuf的CUDA笔记】十五,OpenAI Triton入门笔记三 FusedAttention](https://mp.weixin.qq.com/s/NKShFDrfDGsb0G6PAkUCGw)
- [AI Infra论文阅读之通过打表得到训练大模型的最佳并行配置](https://mp.weixin.qq.com/s/D-14J482SFQf-zh-EFa-1w)
- [AI Infra论文阅读之将流水线并行气泡几乎降到零(附基于Meagtron-LM的ZB-H1开源代码实现解读)](https://mp.weixin.qq.com/s/PXjYm9dN8C9B8svMQ7nOvw)
- [AI Infra论文阅读之LIGHTSEQ(LLM长文本训练的Infra工作)](https://mp.weixin.qq.com/s/u4gG1WZ73mgH9mEKQQCRww)
- [AI Infra论文阅读之《在LLM训练中减少激活值内存》](https://mp.weixin.qq.com/s/WRUmZT5NIbiHSnNrK1vLOw)
- [系统调优助手,PyTorch Profiler TensorBoard 插件教程](https://mp.weixin.qq.com/s/dG-wlwi8oLg8YMQe_A87qQ)
- [在GPU上加速RWKV6模型的Linear Attention计算](https://mp.weixin.qq.com/s/YXtvafdxB1rVeoy0qJmjyA)
- [flash-linear-attention的fused_recurrent_rwkv6 Triton实现精读](https://mp.weixin.qq.com/s/H6wWBxwIJNCzkIlH_uIuiw)
- [flash-linear-attention中的Chunkwise并行算法的理解](https://mp.weixin.qq.com/s/7utRk157_TFxF8gNRCyIyA)
- [硬件高效的线性注意力机制Gated Linear Attention论文阅读](https://mp.weixin.qq.com/s/IVFeHK1ItPVzttmRRa7ycw)
- [GQA,MLA之外的另一种KV Cache压缩方式:动态内存压缩(DMC)](https://mp.weixin.qq.com/s/5pd4fF14ZUgYeM4UXA7ujQ)
- [vAttention:用于在没有Paged Attention的情况下Serving LLM](https://mp.weixin.qq.com/s/F87-Qoo3xYGbwTTYr68guw)
- [大模型KV Cache节省神器MLA学习笔记(包含推理时的矩阵吸收分析)](https://mp.weixin.qq.com/s/cBMrRUdM1IM0T1ji_ODxng)
- [CUDA-MODE 课程笔记 第一课: 如何在 PyTorch 中 profile CUDA kernels](https://mp.weixin.qq.com/s/owF7AFR61SLrOosUPdZPQQ)
- [CUDA-MODE 第一课课后实战(上)](https://mp.weixin.qq.com/s/9XeJPWUsKTaMU2OdPkL-OQ)
- [CUDA-MODE 第一课课后实战(下)](https://mp.weixin.qq.com/s/FCqnQESCQTtlqCG_BSLulA)
- [CUDA-MODE 课程笔记 第二课: PMPP 书的第1-3章速通](https://mp.weixin.qq.com/s/y0fYn8gUqHqEoRO41ftKnA)
- [CUDA-MODE 课程笔记 第四课: PMPP 书的第4-5章笔记](https://mp.weixin.qq.com/s/P87c8LRJ1CEOOyaQw8L-cA)
- [CUDA-MODE课程笔记 第6课: 如何优化PyTorch中的优化器](https://mp.weixin.qq.com/s/qxPYdGZ71DKVLnnYxmvUVA)
- [CUTLASS 2.x & CUTLASS 3.x Intro 学习笔记](https://mp.weixin.qq.com/s/r9b1dGyOr82ooMl4LD1n_Q)
- [CUDA-MODE课程笔记 第7课: Quantization Cuda vs Triton](https://mp.weixin.qq.com/s/1gCgpp49NF7sDw__EpO-nw)
- [TRT-LLM中的Quantization GEMM(Ampere Mixed GEMM)CUTLASS 2.x 课程学习笔记](https://mp.weixin.qq.com/s/NPytrkchX25YRBc_6Zy6nA)
- [CUDA-MODE课程笔记 第8课: CUDA性能检查清单](https://mp.weixin.qq.com/s/zJLDVF-yjuZ_lMjaCHoS5g)
- [TensorRT-LLM 中的 Hopper Mixed GEMM 的 CUTLASS 3.x 实现讲解](https://mp.weixin.qq.com/s/AntEnjuNqrAnU9pe2rGC6Q)
- [通过微基准测试和指令级分析(Instruction-level Analysis)揭秘英伟达Ampere架构](https://mp.weixin.qq.com/s/lmy6Drqh0LbomcaA19Nf8Q)
- [CUDA-MODE课程笔记 第9课: 归约(也对应PMPP的第10章)](https://mp.weixin.qq.com/s/jdZEPLIzgKm8hilXIUKUww)
- [【翻译】Accelerating Llama3 FP8 Inference with Triton Kernels](https://mp.weixin.qq.com/s/v6Ah4uFtI2zTgiAZ3-mKvw)
- [【PyTorch 奇淫技巧】Python Custom Operators翻译](https://mp.weixin.qq.com/s/1P5gXcDhQxavsgo2IYP6rQ)
- [【翻译】教程:在PyTorch中为CUDA库绑定Python接口](https://mp.weixin.qq.com/s/sgFP59OT-Ex2F9zguSr2Rg)
- [【翻译】教程:CUTLASS中的矩阵转置 (使用CuTe把矩阵转置优化到GPU内存带宽上下限)](https://mp.weixin.qq.com/s/IQaD4Cq0SEVjmus1wB4-cg)
- [CUDA-MODE课程笔记 第11课: Sparsity](https://mp.weixin.qq.com/s/28Ku4_EXm0H-ipJX9LKF6g)
- [【PyTorch 奇淫技巧】Async Checkpoint Save](https://mp.weixin.qq.com/s/DcNjBi_rJKvrU9Ssp8Mo0Q)
- [CUDA-MODE课程笔记 第12课,Flash Attention](https://mp.weixin.qq.com/s/IBeBHO5WlS5BfyL0nZaDHg)
- [【翻译】在 GPU 上如何加速 GPTQ Triton 反量化kernel](https://mp.weixin.qq.com/s/CX6lPJOVYRPlpFS_WbGbmg)
- [基于o1-preview解读 Optimized GPTQ INT4 Dequantization Triton Kernel](https://mp.weixin.qq.com/s/xhCNBjFr6m5hPDPGIhDP7w)
- [【翻译】深入探讨 Hopper TMA 单元在 FP8 GEMM 运算中的应用](https://mp.weixin.qq.com/s/cZRoRq_gzAdA2iaMpZ08VA)
- [【翻译】CUTLASS 教程:掌握 NVIDIA® 张量内存加速器 (TMA)](https://mp.weixin.qq.com/s/0J-JihHhfl77AS2uowA1RA)
- [【PyTorch 奇技淫巧】介绍 depyf:轻松掌握 torch.compile](https://mp.weixin.qq.com/s/Z4VG59ihp_r2H75HLGlMaQ)
- [CUDA-MODE 课程笔记 第13课:Ring Attention](https://mp.weixin.qq.com/s/hvqPhNo3l0tL_-lf978euw)
- [【翻译】torch.compile 的详细示例解析教程](https://mp.weixin.qq.com/s/8FwbaP5q4f_VGWE4vobaMw)
- [【翻译】【PyTorch 奇技淫巧】FlexAttetion 基于Triton打造灵活度拉满的Attention](https://mp.weixin.qq.com/s/KJUk-jmwGPrJvVuLQ44DyQ)
- [Flex Attention API 应用 Notebook 代码速览](https://mp.weixin.qq.com/s/ufOKYJn6z19MreiEk0YAEA)
- [【翻译】CUDA-Free Inference for LLMs](https://mp.weixin.qq.com/s/KlxBzBNxyRBnoEr8qXjgeg)
- [CUDA-MODE 课程笔记 第14课,Triton 实践指南](https://mp.weixin.qq.com/s/bWn4epnUAkHc-7nQGJjpyw)
- [【翻译】使用PyTorch FSDP最大化训练吞吐量](https://mp.weixin.qq.com/s/6wNX38rKcFjxLb4ooYQokw)
- [【翻译】使用PyTorch FSDP和Torch.compile最大化训练吞吐量](https://mp.weixin.qq.com/s/YVVau7boVUEnVB6o_qKORA)
- [【ml-engineering 翻译系列】大模型推理](https://mp.weixin.qq.com/s/9417IxdvNMYThjmaSwPBTw)
- [【ml-engineering 翻译系列】AI系统中的网络概述](https://mp.weixin.qq.com/s/dhspQMOHerIpKESb4IWCgg)
- [【ml-engineering 翻译系列】AI系统中的网络 debug](https://mp.weixin.qq.com/s/sne7cjEnzzSW_5bsAn-P3A)
- [【ml-engineering 翻译系列】AI系统中的网络 benchmark](https://mp.weixin.qq.com/s/FlSkBykNIFXfc6TnqOX25A)
- [【翻译】在FSDP2中开启Float8 All-Gather](https://mp.weixin.qq.com/s/44zFNWr5aVtA3zPtegY9dg)
- [【ml-engineering 翻译系列】训练之模型并行](https://mp.weixin.qq.com/s/VTrTM121jEPGEuFaeIT4Cw)
- [梳理下Flash Attention的dispatch逻辑](https://mp.weixin.qq.com/s/Dcw0F4HpV33Uziy2lvNUeA)
- [【ml-engineering 翻译系列】计算加速器之cpu](https://mp.weixin.qq.com/s/IQd4lz8ebQTrkj_lwDXuSA)
- [CUDA-MODE课程笔记 Lecture 16 通过CUDA C++核心库把llm.c移植为llm.cpp](https://mp.weixin.qq.com/s/ynJwHLH9LFKNBYBBWgU25A)
- [GPU 矩阵乘实际可达最大FLOPS测量工具](https://mp.weixin.qq.com/s/kkIxIUaKtSECMNcvma_ayg)
- [CUDA-MODE 课程笔记 第28课 用在生产环境中的LinkedIn Liger kernel](https://mp.weixin.qq.com/s/Mcmii9XYR7zw2H_DA8IUUQ)
- [RMSNorm的精度陷阱:记一次LLM推理精度调查](https://mp.weixin.qq.com/s/Jag-WRH_2w5-GjTYbRnb-Q)
- [如何正确理解NVIDIA GPU利用率的概念 ](https://mp.weixin.qq.com/s/sYJvdqB9PGhEJphMkuSOzw)
- [CUDA-MODE 课程笔记 第29课 Triton内部机制](https://mp.weixin.qq.com/s/7tfTXaG7D208l_5DzN9hBw)
- [GTX 4090 的 cuda graph 诡异](https://mp.weixin.qq.com/s/SAfnlT4aTd67sRqOAoCxQg)
- [【ml-engineering 翻译系列】计算加速器之gpu](https://mp.weixin.qq.com/s/1B52ORme3s2gzpXPXGNNQw)
- [CUDA-MODE课程笔记 第17课 GPU集合通信(NCCL)](https://mp.weixin.qq.com/s/1QdEJKs4a4u3BepNQ716cQ)
- [Triton Kernel 编译阶段](https://mp.weixin.qq.com/s/dw9bP1ZI__0yrf2_wb6nag)
- [使用torchtune把LLaMa-3.1 8B蒸馏为1B](https://mp.weixin.qq.com/s/TfH9tqNjIdNiIi9iwSdY7w)
- [[分布式训练与TorchTitan] PyTorch中的Async Tensor Parallelism介绍](https://mp.weixin.qq.com/s/Jx4B-sF9dudg7OOT-FbsLg)
- [PyTorch 博客 CUTLASS Ping-Pong GEMM Kernel 简介](https://mp.weixin.qq.com/s/QWS9YEjsbM7hzy5tJm--1g)
- [PyTorch博客 《使用 Triton 加速 2D 动态块量化 Float8 GEMM 简介》](https://mp.weixin.qq.com/s/oK45nVPTctIHW-rXbJ128Q)
- [使用NCU和Cursor Claude-sonnet-3.5写出高效cuda算子的正确姿势](https://mp.weixin.qq.com/s/YEw8JZxn15CfLEnK32Jj-Q)
- [Fused AllGather_MatMul Triton工程实现](https://mp.weixin.qq.com/s/oMkyrelpXjc3-KUQBVx6Tg)
- [MoE之年的总结和MoE 推理优化的一些认识](https://mp.weixin.qq.com/s/RXFmnVI_JIlT0Yo6bN3ZHg)
- [SGLang DP MLA 特性解读](https://mp.weixin.qq.com/s/X2uA507VbQVCv3JIQ8EtPA)
- [Windsurf(可平替 Cursor) 的使用体验和技巧](https://mp.weixin.qq.com/s/3PNaEom76jQ8bdxNtYWkkA)
- [SGLang MLA 实现解析](https://mp.weixin.qq.com/s/wRIjy_HHAH_CeEhkZ_BvNg)
- [详解vLLM和SGLang awq dequantize kernel的魔法](https://mp.weixin.qq.com/s/X9AOH1HGXJ3t0jZ5_hd7Ew)
- [SGLang 支持Flash Attention V3 Backend](https://mp.weixin.qq.com/s/FjFi1ORhAyJITTJNA9G3wA)
- [分享一个DeepSeek V3和R1中 Shared Experts和普通Experts融合的一个小技巧](https://mp.weixin.qq.com/s/Bz3qdkldULZiZ8ypooOX-A)### 21. CUDA/大模型 学习资料收集
#### 专栏
- [CUDA编程入门及优化 专栏by jie.hang](https://www.zhihu.com/column/c_1522503697624346624)
- [深入浅出GPU优化 专栏by 有了琦琦的棍子](https://www.zhihu.com/column/c_1437330196193640448)
- [CUDA 编程入门](https://www.zhihu.com/column/c_1699097150611595264)
- [reed CUDA高性能编程](https://www.zhihu.com/column/c_1696937812497235968)#### CUDA 相关博客
点击展开/收起 CUDA优质博客列表
- [一文读懂nvidia-smi topo的输出](https://zhuanlan.zhihu.com/p/692947173)
- [如果你是一个C++面试官,你会问哪些问题?](https://www.zhihu.com/question/451327108/answer/3299498791)
- [推理部署工程师面试题库](https://zhuanlan.zhihu.com/p/673046520)
- [[C++特性]对std::move和std::forward的理解](https://zhuanlan.zhihu.com/p/469607144)
- [论文阅读:Mimalloc Free List Sharding in Action](https://zhuanlan.zhihu.com/p/665602526)
- [在 C++ 中,RAII 有哪些妙用?](https://zhuanlan.zhihu.com/p/687230917)
- [AI/HPC面试问题整理](https://zhuanlan.zhihu.com/p/663917237)
- [Roofline Model与深度学习模型的性能分析](https://zhuanlan.zhihu.com/p/34204282)
- [FlashAttention核心逻辑以及V1 V2差异总结](https://zhuanlan.zhihu.com/p/665170554)
- [flash attention 1和flash attention 2算法的python和triton实现](https://zhuanlan.zhihu.com/p/662759306)
- [Flash Attention 推公式](https://zhuanlan.zhihu.com/p/646697716)
- [图解大模型计算加速系列:FlashAttention V1,从硬件到计算逻辑](https://zhuanlan.zhihu.com/p/669926191)
- [flash attention完全解析和CUDA零基础实现](https://zhuanlan.zhihu.com/p/658947627)
- [FlashAttention图解(如何加速Attention)](https://zhuanlan.zhihu.com/p/626079753)
- [FlashAttention:加速计算,节省显存, IO感知的精确注意力](https://zhuanlan.zhihu.com/p/639228219)
- [FlashAttention 反向传播运算推导](https://zhuanlan.zhihu.com/p/631106302)
- [比标准Attention提速5-9倍,大模型都在用的FlashAttention v2来了](https://zhuanlan.zhihu.com/p/644324647)
- [FlashAttention 的速度优化原理是怎样的?](https://www.zhihu.com/question/611236756/answer/3134408839)
- [FlashAttention 的速度优化原理是怎样的?](https://www.zhihu.com/question/611236756/answer/3132304304)
- [FlashAttention2详解(性能比FlashAttention提升200%)](https://zhuanlan.zhihu.com/p/645376942)
- [FlashAttenion-V3: Flash Decoding详解](https://zhuanlan.zhihu.com/p/661478232)
- [速通PageAttention2](https://zhuanlan.zhihu.com/p/671293276)
- [PageAttention代码走读](https://zhuanlan.zhihu.com/p/668736097)
- [大模型推理加速之FlashDecoding++:野生Flash抵达战场](https://zhuanlan.zhihu.com/p/665361668)
- [学习Flash Attention和Flash Decoding的一些思考与疑惑](https://zhuanlan.zhihu.com/p/664704050)
- [大模型推理加速之Flash Decoding:更小子任务提升并行度](https://zhuanlan.zhihu.com/p/664264445)
- [FlashAttention与Multi Query Attention](https://zhuanlan.zhihu.com/p/640312259)
- [动手Attention优化1:Flash Attention 2优化点解析](https://zhuanlan.zhihu.com/p/634427617)
- [Flash Attention推理性能探究](https://zhuanlan.zhihu.com/p/652691133)
- [记录Flash Attention2-对1在GPU并行性和计算量上的一些小优化](https://zhuanlan.zhihu.com/p/650947918)
- [[LLM] FlashAttention 加速attention计算[理论证明|代码解读]](https://zhuanlan.zhihu.com/p/646084771)
- [FlashAttention核心逻辑以及V1 V2差异总结](https://zhuanlan.zhihu.com/p/665170554)
- [【手撕LLM-FlashAttention】从softmax说起,保姆级超长文!!](https://zhuanlan.zhihu.com/p/663932651)
- [动手Attention优化2:图解基于PTX的Tensor Core矩阵分块乘法实现](https://zhuanlan.zhihu.com/p/650374808)
- [flash attention 的几个要点](https://zhuanlan.zhihu.com/p/663381513)
- [GPU内存(显存)的理解与基本使用](https://zhuanlan.zhihu.com/p/462191421)
- [图文并茂,超详细解读nms cuda拓展源码](https://zhuanlan.zhihu.com/p/466169614)
- [大模型的好伙伴,浅析推理加速引擎FasterTransformer](https://zhuanlan.zhihu.com/p/626008090)
- [LLM Inference CookBook(持续更新)](https://zhuanlan.zhihu.com/p/619596323)
- [NVIDIA的custom allreduce](https://zhuanlan.zhihu.com/p/611229620)
- [[论文速读] FlashAttention: Fast and Memory-Efficient Exact Attention with IO-Awareness](https://zhuanlan.zhihu.com/p/548811565)
- [CUDA随笔之Stream的使用](https://zhuanlan.zhihu.com/p/51402722)
- [简单读读FasterTransformer](https://zhuanlan.zhihu.com/p/589442432)
- [cutlass FusedMultiheadAttention代码解读](https://zhuanlan.zhihu.com/p/600373700)
- [简单谈谈CUDA Reduce](https://zhuanlan.zhihu.com/p/559549740)
- [GridReduce - CUDA Reduce 部分结果归约](https://zhuanlan.zhihu.com/p/635456406)
- [CUTLASS: Fast Linear Algebra in CUDA C++](https://zhuanlan.zhihu.com/p/461060382)
- [cutlass源码导读(1)——API与设计理念](https://zhuanlan.zhihu.com/p/588953452)
- [cutlass源码导读(2)——Gemm的计算流程](https://zhuanlan.zhihu.com/p/592689326)
- [CUDA GroupNorm NHWC优化](https://zhuanlan.zhihu.com/p/596871310)
- [传统 CUDA GEMM 不完全指北](https://zhuanlan.zhihu.com/p/584236348)
- [怎么评估内存带宽的指标,并进行优化?](https://www.zhihu.com/question/424477202/answer/2322341112)
- [TensorRT Diffusion模型优化点](https://zhuanlan.zhihu.com/p/592713879)
- [NVIDIA GPU性能优化基础](https://zhuanlan.zhihu.com/p/577412348)
- [一文理解 PyTorch 中的 SyncBatchNorm](https://zhuanlan.zhihu.com/p/555881100)
- [如何开发机器学习系统:高性能GPU矩阵乘法](https://zhuanlan.zhihu.com/p/531498210)
- [CUDA SGEMM矩阵乘法优化笔记——从入门到cublas](https://zhuanlan.zhihu.com/p/518857175)
- [Dropout算子的bitmask优化](https://zhuanlan.zhihu.com/p/517766170)
- [面向 Tensor Core 的算子自动生成](https://zhuanlan.zhihu.com/p/502935328)
- [PICASSO论文学习](https://zhuanlan.zhihu.com/p/500026086)
- [CUDA翻译:How to Access Global Memory Efficiently in CUDA C/C++ Kernels](https://zhuanlan.zhihu.com/p/473133201)
- [CUDA Pro Tips翻译:Write Flexible Kernels with Grid-Stride Loops](https://zhuanlan.zhihu.com/p/472952257)
- [[施工中] CUDA GEMM 理论性能分析与 kernel 优化](https://zhuanlan.zhihu.com/p/441146275)
- [CUDA Ampere Tensor Core HGEMM 矩阵乘法优化笔记 —— Up To 131 TFLOPS!](https://zhuanlan.zhihu.com/p/555339335)
- [Nvidia Tensor Core-CUDA HGEMM优化进阶](https://zhuanlan.zhihu.com/p/639297098)
- [CUDA C++ Best Practices Guide Release 12.1笔记(一)](https://zhuanlan.zhihu.com/p/636103380)
- [CUDA 矩阵乘法终极优化指南](https://zhuanlan.zhihu.com/p/410278370)
- [如何用CUDA写有CuBLAS 90%性能的GEMM Kernel](https://zhuanlan.zhihu.com/p/631227862)
- [如何理解Nvidia英伟达的Multi-GPU多卡通信框架NCCL?](https://www.zhihu.com/question/63219175/answer/2768301153)
- [如何理解Nvidia英伟达的Multi-GPU多卡通信框架NCCL?](https://www.zhihu.com/question/63219175/answer/206697974)
- [如何理解Nvidia英伟达的Multi-GPU多卡通信框架NCCL?](https://www.zhihu.com/question/63219175/answer/3487108775)
- [使用FasterTransformer实现LLM分布式推理](https://zhuanlan.zhihu.com/p/644322962)
- [细粒度GPU知识点详细总结](https://zhuanlan.zhihu.com/p/349185459)
- [https://siboehm.com/articles/22/CUDA-MMM](https://siboehm.com/articles/22/CUDA-MMM)
- [【CUDA编程】OneFlow Softmax算子源码解读之BlockSoftmax](https://zhuanlan.zhihu.com/p/646998408)
- [【CUDA编程】OneFlow Softmax 算子源码解读之WarpSoftmax](https://zhuanlan.zhihu.com/p/646994689)
- [【CUDA编程】OneFlow Element-Wise 算子源码解读](https://zhuanlan.zhihu.com/p/646990764)
- [【CUDA编程】Faster Transformer v1.0 源码详解](https://zhuanlan.zhihu.com/p/647012855)
- [【CUDA编程】Faster Transformer v2.0 源码详解](https://zhuanlan.zhihu.com/p/650462095)
- [FasterTransformer Decoding 源码分析(七)-FFNLayer MoE(上篇)](https://zhuanlan.zhihu.com/p/670916589)
- [FasterTransformer Decoding 源码分析(八)-FFNLayer MoE(下篇)](https://zhuanlan.zhihu.com/p/672189305)
- [从roofline模型看CPU矩阵乘法优化](https://zhuanlan.zhihu.com/p/655421318)
- [性能优化的终极手段之 Profile-Guided Optimization (PGO)](https://zhuanlan.zhihu.com/p/652814504)
- [有没有大模型推理加速引擎FasterTransformer入门级教程?](https://www.zhihu.com/question/602468960/answer/3203088852)
- [深入浅出GPU优化系列:gemv优化](https://zhuanlan.zhihu.com/p/494144694)
- [NVIDIA Hopper架构TensorCore分析(4)](https://zhuanlan.zhihu.com/p/654067822)
- [GPU host+device的编译流程](https://zhuanlan.zhihu.com/p/655850951)
- [Tensor Core 优化半精度矩阵乘揭秘](https://zhuanlan.zhihu.com/p/658306956)
- [无痛CUDA实践:μ-CUDA 自动计算图生成](https://zhuanlan.zhihu.com/p/658080362)
- [CUDA(三):通用矩阵乘法:从入门到熟练](https://zhuanlan.zhihu.com/p/657632577)
- [自己写的CUDA矩阵乘法能优化到多快?](https://www.zhihu.com/question/41060378/answer/2645323107)
- [高效CUDA Scan算法浅析](https://zhuanlan.zhihu.com/p/499963645)
- [一次 CUDA Graph 调试经历](https://zhuanlan.zhihu.com/p/661451140)
- [CUDA中的radix sort算法](https://zhuanlan.zhihu.com/p/488016994)
- [NVIDIA Tensor Core微架构解析](https://zhuanlan.zhihu.com/p/660531822)
- [cutlass cute 101](https://zhuanlan.zhihu.com/p/660379052)
- [在GPU避免分支的方法](https://zhuanlan.zhihu.com/p/143571980)
- [Pytorch-CUDA从入门到放弃(二)](https://zhuanlan.zhihu.com/p/48463543)
- [腾讯机智团队分享--AllReduce算法的前世今生](https://zhuanlan.zhihu.com/p/79030485)
- [cute 之 Layout](https://zhuanlan.zhihu.com/p/661182311)
- [cute Layout 的代数和几何解释](https://zhuanlan.zhihu.com/p/662089556)
- [cute 之 GEMM流水线](https://zhuanlan.zhihu.com/p/665082713)
- [Using CUDA Warp-Level Primitives](https://zhuanlan.zhihu.com/p/664395938)
- [CUDA Pro Tip: Increase Performance with Vectorized Memory Access](https://zhuanlan.zhihu.com/p/666480387)
- [cute 之 简单GEMM实现](https://zhuanlan.zhihu.com/p/667521327)
- [cute 之 MMA抽象](https://zhuanlan.zhihu.com/p/663092747)
- [cute 之 Tensor](https://zhuanlan.zhihu.com/p/663093816)
- [cute Swizzle细谈](https://zhuanlan.zhihu.com/p/684250988)
- [基于 CUTE 的 GEMM 优化【2】—— 高效 GEMM 实现,超越 Cublas 20%](https://zhuanlan.zhihu.com/p/696028389)
- [CUDA单精度矩阵乘法(sgemm)优化笔记](https://zhuanlan.zhihu.com/p/638820727)
- [HPC(高性能计算第一篇) :一文彻底搞懂并发编程与内存屏障(第一篇)](https://zhuanlan.zhihu.com/p/670350655)
- [GPU CUDA 编程的基本原理是什么? 怎么入门?](https://www.zhihu.com/question/613405221/answer/3129776636)
- [如何入门 OpenAI Triton 编程?](https://www.zhihu.com/question/622685131/answer/3217107882)
- [CUDA(二):GPU的内存体系及其优化指南](https://zhuanlan.zhihu.com/p/654027980)
- [nvitop: 史上最强GPU性能实时监测工具](https://zhuanlan.zhihu.com/p/614024375)
- [使用Triton在模型中构建自定义算子](https://zhuanlan.zhihu.com/p/670326958)
- [CUDA笔记 内存合并访问](https://zhuanlan.zhihu.com/p/641639133)
- [GPGPU架构,编译器和运行时](https://zhuanlan.zhihu.com/p/592975749)
- [GPGPU的memory 体系理解](https://zhuanlan.zhihu.com/p/658081469)
- [nvlink那些事……](https://zhuanlan.zhihu.com/p/639228770)
- [对NVidia Hopper GH100 的一些理解](https://zhuanlan.zhihu.com/p/486224812)
- [黑科技:用cutlass进行低成本、高性能卷积算子定制开发](https://zhuanlan.zhihu.com/p/258931422)
- [乱谈Triton Ampere WMMA (施工中)](https://zhuanlan.zhihu.com/p/675925978)
- [可能是讲的最清楚的WeightonlyGEMM博客](https://zhuanlan.zhihu.com/p/675427125)
- [GPU 底层机制分析:kernel launch 开销](https://zhuanlan.zhihu.com/p/544492099)
- [GPU内存(显存)的理解与基本使用](https://zhuanlan.zhihu.com/p/462191421)
- [超越AITemplate,打平TensorRT,SD全系列模型加速框架stable-fast隆重登场](https://zhuanlan.zhihu.com/p/669610362)
- [[手把手带你入门CUTLASS系列] 0x00 cutlass基本认知---为什么要用cutlass](https://zhuanlan.zhihu.com/p/677616101)
- [[手把手带你入门CUTLASS系列] 0x02 cutlass 源码分析(一) --- block swizzle 和 tile iterator (附tvm等价code)](https://zhuanlan.zhihu.com/p/679929705)
- [[手把手带你入门CUTLASS系列] 0x03 cutlass 源码分析(二) --- bank conflict free 的shared memory layout (附tvm等价pass)](https://zhuanlan.zhihu.com/p/681966685)
- [[深入分析CUTLASS系列] 0x04 cutlass 源码分析(三) --- 多级流水线(software pipeline)](https://zhuanlan.zhihu.com/p/687397095)
- [[深入分析CUTLASS系列] 0x03 cutlass 源码分析(二) --- bank conflict free 的shared memory layout (附tvm等价pass)](https://zhuanlan.zhihu.com/p/681966685)
- [GPU 内存概念浅析](https://zhuanlan.zhihu.com/p/651179378)
- [NV_GPU tensor core 算力/带宽/编程模型分析](https://zhuanlan.zhihu.com/p/638129792)
- [Nsight Compute - Scheduler Statistics](https://zhuanlan.zhihu.com/p/673770855)
- [NVidia GPU指令集架构-前言](https://zhuanlan.zhihu.com/p/686198447)
- [搞懂 CUDA Shared Memory 上的 bank conflicts 和向量化指令(LDS.128 / float4)的访存特点](https://zhuanlan.zhihu.com/p/690052715)
- [窥探Trition的lower(二)](https://zhuanlan.zhihu.com/p/695255185)
- [窥探Trition的lower(三)](https://zhuanlan.zhihu.com/p/696133729)
- [ops(2):SoftMax 算子的 CUDA 实现与优化](https://zhuanlan.zhihu.com/p/695307283)
- [cuda学习日记(6) nsight system / nsight compute](https://zhuanlan.zhihu.com/p/640344249)
- [ops(3):Cross Entropy 的 CUDA 实现](https://zhuanlan.zhihu.com/p/695594396)
- [cuda的ldmatrix指令的详细解释](https://zhuanlan.zhihu.com/p/697228676)
- [揭秘 Tensor Core 底层:如何让AI计算速度飞跃](https://mp.weixin.qq.com/s/UL7CLWp3cmdUgGILr4iVzA)
- [NCCL(NVIDIA Collective Communication Library)的来龙去脉](https://zhuanlan.zhihu.com/p/667221519)
- [ldmatrix与swizzle(笔记)](https://zhuanlan.zhihu.com/p/696231622)
- [GPU上GEMM的边界问题以及优化](https://zhuanlan.zhihu.com/p/699776368)
- [NV Tensor Core and Memory Accelerator 理论分析](https://zhuanlan.zhihu.com/p/601204275)
- [CUTLASS CuTe GEMM细节分析(一)——ldmatrix的选择](https://zhuanlan.zhihu.com/p/702818267)
- [Triton到PTX(1):Elementwise](https://zhuanlan.zhihu.com/p/699979345)
- [由矩阵乘法边界处理引起的CUDA wmma fragment与原始矩阵元素对应关系探究](https://zhuanlan.zhihu.com/p/703476975)
- [NVIDIA Hopper架构TensorCore分析(4)](https://zhuanlan.zhihu.com/p/654067822)
- [NVidia GPU指令集架构-Load和Cache](https://zhuanlan.zhihu.com/p/692445145)
- [NVidia GPU指令集架构-寄存器](https://zhuanlan.zhihu.com/p/688616037)
- [Async Copy 及 Memory Barrier 指令的功能与实现](https://zhuanlan.zhihu.com/p/685168850)
- [tensorcore中ldmatrix指令的优势是什么?](https://www.zhihu.com/question/600927104/answer/3029266372)
- [使用cutlass cute复现flash attention](https://zhuanlan.zhihu.com/p/696323042)
- [1. Cuda矩阵乘法GeMM性能优化](https://zhuanlan.zhihu.com/p/593462636)
- [一步步优化 GEMM by Tensorcore](https://zhuanlan.zhihu.com/p/638522893)
- [CUTLASS 3.x 异构编程随感](https://zhuanlan.zhihu.com/p/689829403)
- [Triton到PTX(1):Elementwise](https://zhuanlan.zhihu.com/p/699979345)
- [Triton到SASS(2):Reduction](https://zhuanlan.zhihu.com/p/703748336)
- [cuda的ldmatrix指令的详细解释](https://zhuanlan.zhihu.com/p/697228676)
- [基于 CuTe 理解 swizzle, LDSM, MMA](https://zhuanlan.zhihu.com/p/934430036)
- [一文读懂nsight system与cuda kernel的时间线分析与可视化](https://zhuanlan.zhihu.com/p/691307737)
- [TileLang: 80行Python kernel代码实现FlashMLA 95%的性能](https://zhuanlan.zhihu.com/p/27965825936)
- [简单CUDA Assembly介绍](https://zhuanlan.zhihu.com/p/27455487044)
- [Deep Gemm 代码浅析](https://zhuanlan.zhihu.com/p/26916462532)
- [如何看懂deepseek ai开源的FlashMLA中的核心cu代码?](https://www.zhihu.com/question/13188512132/answer/113811134716)
- [浅析GEMM优化multistage数怎么算](https://zhuanlan.zhihu.com/p/714353243)
- [DeepSeek: FlashMLA代码解析](https://zhuanlan.zhihu.com/p/26269071923)
- [triton(openai)如何实现splitk和streamk?](https://www.zhihu.com/question/13143162788/answer/108685833211)
- [FlashMLA性能简测](https://zhuanlan.zhihu.com/p/26113545571)
- [DeepSeek-V3/R1 的 Hosting 成本预估](https://zhuanlan.zhihu.com/p/23282743306)
- [实用 Swizzle 教程(一)](https://zhuanlan.zhihu.com/p/20579515046)
- [实用 Swizzle 教程(二)](https://zhuanlan.zhihu.com/p/21142007017)
- [CUDA编程入门之Cooperative Groups(1)](https://zhuanlan.zhihu.com/p/572820342)
- [Flash Attention 3 深度解析](https://zhuanlan.zhihu.com/p/17533058076)
- [flashattention中为什么Br的分块要取min,Bc除以4我理解是M要装下QKVO,Br呢?](https://www.zhihu.com/question/5742804352/answer/57630890590)
- [FlashAttention笔记](https://zhuanlan.zhihu.com/p/12107755947)
- [由GQA性能数据异常引发的对MHA,GQA,MQA 在GPU上的感性分析](https://zhuanlan.zhihu.com/p/708776013)
- [动手Attention优化3:理解Bank Conflict及Cutlass Swizzle](https://zhuanlan.zhihu.com/p/9840919069)
- [如何理解GPU Kernel Grid/Block与SM占用率的关系?什么是Tail Effect?](https://zhuanlan.zhihu.com/p/8627456110)
- [Triton入门笔记(二):flash attention的Triton/CUDA对比(前向传播部分)](https://zhuanlan.zhihu.com/p/849538419)
- [基于 CuTe 理解 swizzle, LDSM, MMA](https://zhuanlan.zhihu.com/p/934430036)
- [NCCL通信C++示例(四): AlltoAll_Split实现与分析](https://zhuanlan.zhihu.com/p/718765726)
- [如何用 Triton实现一个更高效的topk_gating kernel?——算子合并技术](https://zhuanlan.zhihu.com/p/730534981)
- [关于Nsight Compute中Compute Workload Analysis反映的Tensor Pipe Utilization的理解](https://zhuanlan.zhihu.com/p/720562971)
- [MARLIN: Mixed-Precision Auto-Regressive Parallel Inference on Large Language Models论文解读](https://zhuanlan.zhihu.com/p/716412368)
- [Shader中的条件分支能否节省shader的性能?](https://www.zhihu.com/question/329084698/answer/3609014411)
- [LLM Decode GQA & GEMV算子性能分析(一)](https://zhuanlan.zhihu.com/p/715091838)
- [LLM Decode GQA & GEMV算子性能分析(二)](https://zhuanlan.zhihu.com/p/715609504)
- [cute gemm 优化](https://zhuanlan.zhihu.com/p/707715989)
- [[Triton] Triton-Linalg](https://zhuanlan.zhihu.com/p/707274848)
- [cutlass swizzle机制解析(一)](https://zhuanlan.zhihu.com/p/710337546)
- [vLLM源码之PageAttention](https://zhuanlan.zhihu.com/p/711304830)
- [CUTLASS CUTE MMA](https://zhuanlan.zhihu.com/p/688884665)
- [了解FlashAttentionV3的优化需要先了解Hopper的主要技术(Hopper White Paper概述)](https://zhuanlan.zhihu.com/p/708416319)
- [从Hopper架构到HGEMM](https://zhuanlan.zhihu.com/p/30427909948)
- [基于CUTLASS CuTe分析cp.async的Prefetch行为](https://zhuanlan.zhihu.com/p/32486160866)
- [为什么加pad可以解bank conflict?](https://zhuanlan.zhihu.com/p/603016056)
- [cute swizzle](https://zhuanlan.zhihu.com/p/706796240)
- [CUTLASS CuTe GEMM细节分析(三)——Swizzle模板参数的取值](https://zhuanlan.zhihu.com/p/713713957)
- [OpenAI Triton: Why layout is important](https://zhuanlan.zhihu.com/p/672720213)
- [Triton到SASS(5.5):TMA/Multicast/Warp Specialize踩坑记](https://zhuanlan.zhihu.com/p/15027115038)
- [Tile-lang 简介](https://zhuanlan.zhihu.com/p/31180917197)
- [CUTLASS:基于CUTE的矩阵乘法优化](https://zhuanlan.zhihu.com/p/31273798568)
- [Marlin W4A16&W4A8代码走读](https://zhuanlan.zhihu.com/p/707470647)
- [CUTLASS 3: CuTe Layout Algebra](https://zhuanlan.zhihu.com/p/22300321859)#### 大模型Infra相关博客(DeepSeek,VERL, Megatron-LM, SGLang,vLLM,xDiT等)
点击展开/收起 大模型Infra优质博客列表
- [Megatron-LM 分布式执行调研](https://strint.notion.site/Megatron-LM-86381cfe51184b9c888be10ee82f3812)
- [BLOOM 训练背后的技术](https://www.cnblogs.com/Matrix_Yao/p/17238627.html)
- [聊聊 PyTorch2.0 中新的Distributed API](https://mp.weixin.qq.com/s/hOOFE_eFD6a8GKTdnRcJXg)
- [聊聊 PyTorch 中新的Distributed API (二)](https://mp.weixin.qq.com/s/zDSuToVMo4iK3sxF662kvg)
- [【LLM】从零开始训练大模型](https://zhuanlan.zhihu.com/p/636270877)
- [在一张 24 GB 的消费级显卡上用 RLHF 微调 20B LLMs](https://www.cnblogs.com/huggingface/p/17245966.html)
- [人手一个ChatGPT!微软DeepSpeed Chat震撼发布,一键RLHF训练千亿级大模型](https://zhuanlan.zhihu.com/p/621379646)
- [大型语言模型(LLM)训练指南🚀](https://zhuanlan.zhihu.com/p/611325149)
- [“StackLLaMA”: 用 RLHF 训练 LLaMA 的手把手教程](https://zhuanlan.zhihu.com/p/626896135)
- [图解大模型训练之:流水线并行(Pipeline Parallelism),以Gpipe为例](https://zhuanlan.zhihu.com/p/613196255)
- [图解大模型训练之:数据并行上篇(DP, DDP与ZeRO)](https://zhuanlan.zhihu.com/p/617133971)
- [图解大模型训练之:数据并行下篇( DeepSpeed ZeRO,零冗余优化)](https://zhuanlan.zhihu.com/p/618865052)
- [图解大模型训练之:张量模型并行(TP),Megatron-LM](https://zhuanlan.zhihu.com/p/622212228)
- [Megatron-LM 中的 pipeline 并行](https://zhuanlan.zhihu.com/p/432969288)
- [图解大模型系列之:Megatron源码解读1,分布式环境初始化](https://zhuanlan.zhihu.com/p/629121480)
- [图解大模型训练之:Megatron源码解读2,模型并行](https://zhuanlan.zhihu.com/p/634377071)
- [聊聊序列并行Sequence parallelism](https://mp.weixin.qq.com/s/ylScQOpJ1-ufyPK7X6VUjw)
- [深入理解 Megatron-LM(1)基础知识](https://zhuanlan.zhihu.com/p/650234985)
- [深入理解 Megatron-LM(2)原理介绍](https://zhuanlan.zhihu.com/p/650383289)
- [深入理解 Megatron-LM(3)代码结构](https://zhuanlan.zhihu.com/p/650237820)
- [深入理解 Megatron-LM(4)并行设置](https://zhuanlan.zhihu.com/p/650500590)
- [深入理解 Megatron-LM(5)张量并行](https://zhuanlan.zhihu.com/p/650237833)
- [聊聊字节 AML 万卡工作 MegaScale: Scaling Large Language Model Training](https://mp.weixin.qq.com/s/aXsURbHZKzoBw-ChaBnjEQ)
- [深度学习里,模型并行中怎么将模型拆分?](https://www.zhihu.com/question/319355346/answer/2985459442)
- [Transformers DeepSpeed官方文档](https://zhuanlan.zhihu.com/p/621572871)
- [DeepSeek-V3 MTP 工程实现思考](https://zhuanlan.zhihu.com/p/29082207943)
- [DeepSeek V3/R1 推理效率分析(1):关于DeepSeek V3/R1 Decoding吞吐极限的一些不负责任估计](https://zhuanlan.zhihu.com/p/27292649125)
- [DeepSeek V3/R1 推理效率分析(2): DeepSeek 满血版逆向工程分析](https://zhuanlan.zhihu.com/p/29841050824)
- [DeepSeek V3/R1 推理效率分析(3):Decode 配置泛化讨论](https://zhuanlan.zhihu.com/p/29540042383)
- [如何估算不同规格的芯片 EP 部署 Deepseek 的单卡吞吐 V1.0](https://zhuanlan.zhihu.com/p/30471846931)
- [深度解析FlashMLA: 一文读懂大模型加速新利器](https://zhuanlan.zhihu.com/p/27976368445)
- [Flash MLA 笔记](https://zhuanlan.zhihu.com/p/30423929220)
- [MoE Inference On AnyScale](https://zhuanlan.zhihu.com/p/28680264165)
- [大模型分布式通信技术博客汇总](https://zhuanlan.zhihu.com/p/30451575581)
- [sglang 源码学习笔记(一)- Cache、Req与Scheduler](https://zhuanlan.zhihu.com/p/17186885141)
- [DualPipe 深入浅出:没有分布式训练基础也能看懂的 DualPipe 全方位讲解](https://zhuanlan.zhihu.com/p/27045651854)
- [DeepSeek MLA引发的一些记忆碎片](https://zhuanlan.zhihu.com/p/25210365944)
- [DeepSeek MLA的序列并行和张量并行](https://zhuanlan.zhihu.com/p/25573883266)
- [SGLang: Triton算子extend_attention/Prefix优化](https://zhuanlan.zhihu.com/p/22996351654)
- [DeepSeek-V3 (671B) 模型参数量分解计算](https://zhuanlan.zhihu.com/p/21455638257)
- [DeepSeek关键技术再总结](https://zhuanlan.zhihu.com/p/30971034460)
- [PP->VPP->ZeroBubblePP->deepseekv3 dualPipe,对PP bubble的极致压缩](https://zhuanlan.zhihu.com/p/26559590326)
- [双流并行(DualPipe) 没有双流会更好](https://zhuanlan.zhihu.com/p/26915547331)
- [deepseek 训练 profile data 基础分析](https://zhuanlan.zhihu.com/p/26717172494)
- [Deepseek FlashMLA解析](https://zhuanlan.zhihu.com/p/26262350225)
- [理解DeepGEMM源码和实现逻辑](https://zhuanlan.zhihu.com/p/32383172703)
- [DeepEP Dispatch/Combine 图示](https://zhuanlan.zhihu.com/p/29273768638)
- [MoE并行负载均衡:EPLB的深度解析与可视化](https://zhuanlan.zhihu.com/p/29963005584)
- [给 Megatron 的长文本训练抓了一个 Bug](https://zhuanlan.zhihu.com/p/26109356836)
- [对DualPipe的一些想法](https://zhuanlan.zhihu.com/p/21525151726)
- [SGLang: Triton算子prefill_attention](https://zhuanlan.zhihu.com/p/19989050229)
- [[CUDA基础]📚CUDA-Learn-Notes: v3.0 大升级-面试刷题不迷路](https://zhuanlan.zhihu.com/p/19862356369)
- [[大模型推理系统] SGlang的异步调度:Overlap CPU和GPU流水](https://zhuanlan.zhihu.com/p/17744625577)
- [计算DeepSeekV3训练的MFU](https://zhuanlan.zhihu.com/p/16445683081)
- [如何评价 DeepSeek 的 DeepSeek-V3 模型?](https://www.zhihu.com/question/7837132971/answer/65842498313)
- [SGLang _fwd_kernel_stage2 计算公式推导](https://zhuanlan.zhihu.com/p/12749158715)
- [SGLang代码快速上手(with openRLHF)](https://zhuanlan.zhihu.com/p/11536619756)
- [DiT并行推理引擎-xDiT的设计哲学](https://zhuanlan.zhihu.com/p/713199948)
- [记一次对 SGLang weight update latency 的优化](https://zhuanlan.zhihu.com/p/9908228168)
- [vllm代码快速上手](https://zhuanlan.zhihu.com/p/6462326972)
- [由Ring-Attention性能问题引发的计算通信overlap分析](https://zhuanlan.zhihu.com/p/706805407)
- [TensorRT-LLM的allreduce插件](https://zhuanlan.zhihu.com/p/4805166171)
- [DeepSeek-V2 MLA KV Cache 真的省了吗?](https://zhuanlan.zhihu.com/p/714761319)
- [PyTorch FSDP 设计解读](https://zhuanlan.zhihu.com/p/694288870)
- [大模型推理-5-大模型推理优化之缓存及调度](https://zhuanlan.zhihu.com/p/676652273)
- [【22token/s|又提升20%】榨干ktransformers的每一滴性能](https://zhuanlan.zhihu.com/p/30079534043)
- [从零开始设计SGLang的KV Cache](https://zhuanlan.zhihu.com/p/31160183506)
- [LLM(33):MoE 的算法理论与 EP 的工程化问题](https://zhuanlan.zhihu.com/p/28558622452)
- [Megatron中的MoE TokenDispatcher机制](https://zhuanlan.zhihu.com/p/30092100811)
- [KTransformers v0.2.4: 多并发支持(上万行代码的诚意更新),Xeon6+MRDIMM 加持下单机单卡环境下四并发超过 40 tokens/s](https://zhuanlan.zhihu.com/p/1890755315215095344)
- [从零开始的verl框架解析](https://zhuanlan.zhihu.com/p/30876678559)
- [[AI Infra] VeRL 框架入门&代码带读](https://zhuanlan.zhihu.com/p/27676081245)
- [【AI Infra】【RLHF框架】一、VeRL中基于Ray的执行流程源码解析](https://zhuanlan.zhihu.com/p/29997527557)
- [【AI Infra】【RLHF框架】二、VeRL中colocate实现源码解析](https://zhuanlan.zhihu.com/p/31595392436)
- [【AI Infra】【RLHF框架】三、VeRL中的Rollout实现源码解析](https://zhuanlan.zhihu.com/p/1888310042580743730)
- [SGLang-veRL Server:从 Engine 到 Server,我们需要更灵活的 RLHF rollout 接口](https://zhuanlan.zhihu.com/p/1890631652486665464)
- [vLLM V1 源码阅读](https://zhuanlan.zhihu.com/p/32045324831)
- [veRL框架初探](https://im9jhce8va.feishu.cn/docx/HQ1Hd8OcKoekhFxgkgJcnW66n8f?from=from_copylink)#### 大模型和AIGC的演进记录
点击展开/收起 大模型和AIGC的演进
##### Linear Attention
- [github仓库](https://github.com/BlinkDL/RWKV-LM)
- [rwkv论文原理解读](https://www.zhihu.com/question/602564718)
- [RWKV的微调教学,以及RWKV World:支持世界所有语言的生成+对话+任务+代码](https://zhuanlan.zhihu.com/p/638326262)
- [RWKV:用RNN达到Transformer性能,且支持并行模式和长程记忆,既快又省显存,已在14B参数规模检验](https://zhuanlan.zhihu.com/p/599150009)
- [谈谈 RWKV 系列的 prompt 设计,模型选择,解码参数设置](https://zhuanlan.zhihu.com/p/639629050)
- [RWKV进展:一键生成论文,纯CPU高速INT4,纯CUDA脱离pytorch,ctx8192不耗显存不变慢](https://zhuanlan.zhihu.com/p/626083366)
- [开源1.5/3/7B中文小说模型:显存3G就能跑7B模型,几行代码即可调用](https://zhuanlan.zhihu.com/p/609154637)
- [发布几个RWKV的Chat模型(包括英文和中文)7B/14B欢迎大家玩](https://zhuanlan.zhihu.com/p/618011122)
- [实例:手写 CUDA 算子,让 Pytorch 提速 20 倍(某特殊算子)](https://zhuanlan.zhihu.com/p/476297195)
- [BlinkDL/RWKV-World-7B gradio demo](https://huggingface.co/spaces/BlinkDL/RWKV-World-7B/tree/main)
- [ChatRWKV(有可用猫娘模型!)微调/部署/使用/训练资源合集](https://zhuanlan.zhihu.com/p/616351661)
- [pengbo的专栏](https://www.zhihu.com/people/bopengbopeng/posts)
- [RWKV 模型解析](https://zhuanlan.zhihu.com/p/640050680)
- [[线性RNN系列] Mamba: S4史诗级升级](https://zhuanlan.zhihu.com/p/661237120)
- [状态空间模型: RWKV & Mamba](https://zhuanlan.zhihu.com/p/701121020)
- [Transformer,SSM,Linear Attention的联系与理解](https://zhuanlan.zhihu.com/p/705837508)##### MOE
- [mixture-of-experts-with-expert-choice](https://blog.research.google/2022/11/mixture-of-experts-with-expert-choice.html)
- [MoE训练论文解读之Megablocks:打破动态路由限制](https://zhuanlan.zhihu.com/p/653270049)
- [MoE训练论文解读之Tutel: 动态切换并行策略实现动态路由](https://zhuanlan.zhihu.com/p/653518289)
- [ACM SIGCOMM 2023有哪些亮点?](https://www.zhihu.com/question/600051474/answer/3202735839)
- [LLM终身学习的可能性——Mixture of Experts](https://zhuanlan.zhihu.com/p/656015139)
- [MoE 入门介绍 核心工作回顾 模型篇](https://zhuanlan.zhihu.com/p/671434414)
- [大语言模型结构之:浅谈MOE结构](https://zhuanlan.zhihu.com/p/670007189)
- [训不动Mixtral,要不试试LLaMA-MoE?](https://zhuanlan.zhihu.com/p/674085893)
- [Mixtral-8x7B MoE大模型微调实践,超越Llama2-65B](https://zhuanlan.zhihu.com/p/674028456)
- [Mixtral-8x7B 模型挖坑](https://zhuanlan.zhihu.com/p/674751021)
- [Mixture of Experts(MoE)学习笔记](https://zhuanlan.zhihu.com/p/675216281)
- [群魔乱舞:MoE大模型详解](https://zhuanlan.zhihu.com/p/677638939)
- [Mixtral 8x7B论文终于来了:架构细节、参数量首次曝光](https://zhuanlan.zhihu.com/p/677108093)
- [MoE(Mixture-of-Experts)大模型架构的优势是什么?为什么?](https://www.zhihu.com/question/634844209/answer/3364787819)
- [图解大模型训练系列之:DeepSpeed-Megatron MoE并行训练(原理篇](https://zhuanlan.zhihu.com/p/681154742)
- [图解大模型训练系列之:DeepSpeed-Megatron MoE并行训练(源码解读篇)](https://mp.weixin.qq.com/s/AiqmTG8j6lyoHrUV056p5Q)
- [LLM 学习笔记-Deepspeed-MoE 论文](https://zhuanlan.zhihu.com/p/670968683)
- [图解Mixtral 8 * 7b推理优化原理与源码实现](https://mp.weixin.qq.com/s/WUx73P_LN6TA-6DW6nNvKQ)##### 大模型知识介绍
- [压缩下一个 token 通向超过人类的智能](https://zhuanlan.zhihu.com/p/619511222)
- [LLM 入门笔记-Tokenizer](https://zhuanlan.zhihu.com/p/669901093)
- [【Transformer 基础系列】手推显存占用](https://zhuanlan.zhihu.com/p/648924115)
- [《A Survey of Large Language Models》笔记](https://zhuanlan.zhihu.com/p/631065995)
- [分析transformer模型的参数量、计算量、中间激活、KV cache](https://zhuanlan.zhihu.com/p/624740065)
- [Transformer模型的基础演算](https://mp.weixin.qq.com/s/0Er0UOk6Wdky-0gzeQxK0g)
- [Transformer 估算 101](https://zhuanlan.zhihu.com/p/630582034)
- [通向AGI之路:大型语言模型(LLM)技术精要](https://zhuanlan.zhihu.com/p/597586623)
- [Transformer学习笔记二:Self-Attention(自注意力机制)](https://zhuanlan.zhihu.com/p/455399791)
- [Transformer学习笔记三:为什么Transformer要用LayerNorm/Batch Normalization & Layer Normalization (批量&层标准化)](https://zhuanlan.zhihu.com/p/456863215)
- [Transformer学习笔记五:Subword Tokenization(子词分词器)](https://zhuanlan.zhihu.com/p/460678461)
- [ChatGPT技术解析系列之:GPT1、GPT2与GPT3](https://zhuanlan.zhihu.com/p/609367098)
- [ChatGPT技术解析系列之:训练框架InstructGPT](https://zhuanlan.zhihu.com/p/605516116)
- [ChatGPT技术解析系列之:赋予GPT写代码能力的Codex](https://zhuanlan.zhihu.com/p/611313567)
- [大模型推理性能优化之KV Cache解读](https://zhuanlan.zhihu.com/p/630832593)
- [拆解追溯 ChatGPT各项能力的起源](https://zhuanlan.zhihu.com/p/607469120)
- [ChatGPT 的突现能力,我们是否真的面临范式转变?](https://zhuanlan.zhihu.com/p/622052864)
- [复杂推理:大型语言模型的"北极星"能力](https://zhuanlan.zhihu.com/p/628855304)
- [深入理解NLP Subword算法:BPE、WordPiece、ULM](https://zhuanlan.zhihu.com/p/86965595)
- [ChatGPT 背后的“功臣”——RLHF 技术详解](https://www.cnblogs.com/huggingface/p/17040315.html)
- [深入浅出,解析ChatGPT背后的工作原理](https://zhuanlan.zhihu.com/p/597100830)
- [这是Meta版ChatGPT雏形?开源、一块GPU就能跑,1/10参数量打败GPT-3](https://zhuanlan.zhihu.com/p/609544219)
- [LLaMA模型惨遭泄漏,Meta版ChatGPT被迫「开源」!GitHub斩获8k星,评测大量出炉](https://zhuanlan.zhihu.com/p/612009979)
- [LeCun狂赞:600刀GPT-3.5平替! 斯坦福70亿参数「羊驼」爆火,LLaMA杀疯了](https://zhuanlan.zhihu.com/p/613880958)
- [LeCun转赞:在苹果M1/M2芯片上跑LLaMA!130亿参数模型仅需4GB内存](https://zhuanlan.zhihu.com/p/613602977)
- [Stanford Alpaca (羊驼):ChatGPT 学术版开源实现](https://zhuanlan.zhihu.com/p/614354549)
- [Alpaca-Lora (羊驼-Lora): 轻量级 ChatGPT 的开源实现(对标 Standford Alpaca)](https://zhuanlan.zhihu.com/p/615646636)
- [Alpaca-cpp(羊驼-cpp): 可以本地运行的 Alpaca 大语言模型](https://zhuanlan.zhihu.com/p/616267309)
- [NLP(九):LLaMA, Alpaca, ColossalChat 系列模型研究](https://zhuanlan.zhihu.com/p/618695885)
- [全球最大ChatGPT开源平替来了!支持35种语言,写代码、讲笑话全拿捏](https://zhuanlan.zhihu.com/p/616917667)
- [国产ChatGPT又开源了!效果大幅升级,在手机上也可以跑](https://zhuanlan.zhihu.com/p/617679244)
- [世界首款真开源类ChatGPT大模型Dolly 2.0,可随意修改商用](https://zhuanlan.zhihu.com/p/621655147)
- [用ChatGPT训练羊驼:「白泽」开源,轻松构建专属模型,可在线试玩](https://zhuanlan.zhihu.com/p/619453625)
- [3090单卡5小时,每个人都能训练专属ChatGPT,港科大开源LMFlow](https://zhuanlan.zhihu.com/p/618919940)
- [300美元复刻ChatGPT九成功力,GPT-4亲自监考,130亿参数开源模型「小羊驼」来了](https://zhuanlan.zhihu.com/p/618699807)
- [学术专用版ChatGPT火了,一键完成论文润色、代码解释、报告生成](https://zhuanlan.zhihu.com/p/618310974)
- [笔记本就能运行的ChatGPT平替来了,附完整版技术报告](https://zhuanlan.zhihu.com/p/618310404)
- [训练个中文版ChatGPT没那么难:不用A100,开源Alpaca-LoRA+RTX 4090就能搞定](https://zhuanlan.zhihu.com/p/617221484)
- [弥补斯坦福70亿参数「羊驼」短板,精通中文的大模型来了,已开源](https://zhuanlan.zhihu.com/p/616079388)
- [还在为玩不了ChatGPT苦恼?这十几个开源平替也能体验智能对话](https://zhuanlan.zhihu.com/p/615257807)
- [斯坦福70亿参数开源模型媲美GPT-3.5,100美元即可复现](https://zhuanlan.zhihu.com/p/614212219)
- [真·ChatGPT平替:无需显卡,MacBook、树莓派就能运行LLaMA](https://zhuanlan.zhihu.com/p/613923687)
- [ChatGPT开源替代来了!参数量200亿,在4300万条指令上微调而成](https://zhuanlan.zhihu.com/p/613609788)
- [B站UP主硬核自制智能音箱:有ChatGPT加持,才是真・智能](https://zhuanlan.zhihu.com/p/599602043)
- [熔岩羊驼LLaVA来了:像GPT-4一样可以看图聊天,无需邀请码,在线可玩](https://zhuanlan.zhihu.com/p/624442883)
- [3天近一万Star,无差体验GPT-4识图能力,MiniGPT-4看图聊天、还能草图建网站](https://zhuanlan.zhihu.com/p/623731818)
- [ChatGPT 中文调教指南。各种场景使用指南。学习怎么让它听你的话](https://github.com/PlexPt/awesome-chatgpt-prompts-zh)
- [ChatGPT提示工程师|AI大神吴恩达教你写提示词](https://www.bilibili.com/video/BV1No4y1t7Zn/?vd_source=4dffb0fbabed4311f4318e8c6d253a10)
- [[分析] 浅谈ChatGPT的Tokenizer](https://zhuanlan.zhihu.com/p/626621158)
- [OPT-175B是如何炼成的](https://zhuanlan.zhihu.com/p/622061951)
- [Meta复刻GPT-3“背刺”OpenAI,完整模型权重及训练代码全公开](https://zhuanlan.zhihu.com/p/509100358)
- [Limitations of LLaMA](https://zhuanlan.zhihu.com/p/618776565)
- [Hugging News #0506: StarCoder, DeepFloyd/IF 好多新的重量级模型](https://zhuanlan.zhihu.com/p/627319332)
- [StarCoder: 最先进的代码大模型](https://zhuanlan.zhihu.com/p/627840388)
- [VideoChat🦜: 基于视频指令数据微调的聊天机器人](https://zhuanlan.zhihu.com/p/628712512)
- [MiniGPT-4 本地部署 RTX 3090](https://zhuanlan.zhihu.com/p/624417097)
- [更擅长推理的LLaMA大模型,支持中文!](https://zhuanlan.zhihu.com/p/628688680)
- [点击鼠标,让ChatGPT更懂视觉任务!](https://zhuanlan.zhihu.com/p/628266214)
- [[分析] ROPE的不同实现:llama&palm](https://zhuanlan.zhihu.com/p/627536105)
- [羊驼系列大模型和ChatGPT差多少?详细测评后,我沉默了](https://zhuanlan.zhihu.com/p/629085937)
- [【开源骆驼】更好的翻译prompt,中英文token比例,比alpaca更强的中文数据集WizardLM](https://zhuanlan.zhihu.com/p/629379775)
- [ImageBind: 表征大一统?也许还有一段距离](https://zhuanlan.zhihu.com/p/629389992)
- [训练开销骤减,10%成本定制专属类GPT-4多模态大模型](https://mp.weixin.qq.com/s/UqBEGLpF6H7NU9jyqbvRLg)
- [国内首个可复现的RLHF基准,北大团队开源 PKU-Beaver](https://mp.weixin.qq.com/s/O1RDHrmEg99zCil8ycqOGQ)
- [北大紧跟步伐开源PKU-Beaver (河狸)——不仅支持RLHF训练, 还开源RLHF训练数据](https://zhuanlan.zhihu.com/p/630326764)
- [大模型迎来「开源季」,盘点过去一个月那些开源的LLM和数据集](https://mp.weixin.qq.com/s/VleZkQT6Vga7vqZP8pvgQQ)
- [超越GPT-4!华人团队爆火InstructBLIP抢跑看图聊天,开源项目横扫多项SOTA](https://mp.weixin.qq.com/s/jI1cf7FDYJscHDZKiNvoug)
- [基于 ChatGLM-6B 搭建个人专属知识库](https://zhuanlan.zhihu.com/p/629558941)
- [大模型-LLM分布式训练框架总结](https://zhuanlan.zhihu.com/p/623746805)
- [没有RLHF,一样媲美GPT-4、Bard,Meta发布650亿参数语言模型LIMA](https://mp.weixin.qq.com/s/Oze93Brun-AQUBI5Tt1b6w)
- [在Transformer时代重塑RNN,RWKV将非Transformer架构扩展到数百亿参数](https://mp.weixin.qq.com/s/cg8F4cE6JGij7JJJivUqxg)
- [马腾宇团队新出大模型预训练优化器,比Adam快2倍,成本减半](https://mp.weixin.qq.com/s/L_66ZWTeLE43gQtSi1reEw)
- [跑分达ChatGPT的99%,人类难以分辨!开源「原驼」爆火,iPhone都能微调大模型了](https://mp.weixin.qq.com/s/1ZrPtBmgkklFk2_TvOhK_w)
- [大模型词表扩充必备工具SentencePiece](https://zhuanlan.zhihu.com/p/630696264)
- [RWKV – transformer 与 RNN 的强强联合](https://zhuanlan.zhihu.com/p/633735524)
- [Falcon 登陆 Hugging Face 生态](https://zhuanlan.zhihu.com/p/637676443)
- [详解大模型RLHF过程(配代码解读)](https://zhuanlan.zhihu.com/p/624589622)
- [详解Transformer-XL](https://zhuanlan.zhihu.com/p/271984518)
- [教科书级数据is all you need:1.3B小模型逆袭大模型的秘密](https://zhuanlan.zhihu.com/p/608004441)
- [清华第二代60亿参数ChatGLM2开源!中文榜居首,碾压GPT-4,推理提速42%](https://zhuanlan.zhihu.com/p/639888131)
- [NLP(十七):从 FlashAttention 到 PagedAttention, 如何进一步优化 Attention 性能](https://zhuanlan.zhihu.com/p/638468472)
- [AGI最前沿:GPT-4之后大模型学术进展速览](https://zhuanlan.zhihu.com/p/639165892)
- [LLM学习记录(一)--关于大模型的一些知识](https://zhuanlan.zhihu.com/p/624918286)
- [UC伯克利LLM排行榜首次重磅更新!GPT-4稳居榜首,全新330亿参数「小羊驼」位列开源第一](https://zhuanlan.zhihu.com/p/607403006)
- [【Falcon Paper】我们是靠洗数据洗败 LLaMA 的!](https://zhuanlan.zhihu.com/p/637996787)
- [[中文开源震撼首发]33B QLoRA大语言模型Anima真的太强大了!QLoRA技术可能是AI转折点!](https://zhuanlan.zhihu.com/p/638058537)
- [详解大模型RLHF过程(配代码解读)](https://zhuanlan.zhihu.com/p/624589622)
- [羊驼家族大模型集体进化!32k上下文追平GPT-4,成本忽略不计](https://zhuanlan.zhihu.com/p/640156580)
- [大模型LLM知识整理](https://zhuanlan.zhihu.com/p/641109766)
- [Relative position embedding](https://zhuanlan.zhihu.com/p/364828960)
- [ICLR 2023 Spotlight | ViT-Adapter:针对原始ViT结构设计密集预测任务适配器](https://zhuanlan.zhihu.com/p/608272954)
- [DevChat:将 GPT-4 无缝融入 VS Code,极致提升你的编程体验](https://zhuanlan.zhihu.com/p/640807148)
- [OpenAI早就不卷大模型,开始卷AI Agents了?这是一篇来自OpenAI应用研究主管关于Agent的万字长文](https://zhuanlan.zhihu.com/p/640634046)
- [为什么说大模型训练很难?](https://www.zhihu.com/question/498271491/answer/3052744672)
- [LLM学习记录(五)--超简单的RoPE理解方式](https://zhuanlan.zhihu.com/p/642289220)
- [langchain源码剖析-模块整体介绍【1】](https://zhuanlan.zhihu.com/p/640848809)
- [如何为GPT/LLM模型添加额外知识?](https://www.zhihu.com/question/591935281/answer/2995472929)
- [LLaMA Plus版来了,谷歌推出LongLLaMA,不仅让你的大模型更集中注意力,还能处理超长上线文](https://zhuanlan.zhihu.com/p/642551367)
- [Transformer升级之路:10、RoPE是一种β进制编码](https://zhuanlan.zhihu.com/p/643630735)
- [大模型的幻觉问题调研: LLM Hallucination Survey](https://zhuanlan.zhihu.com/p/642648601)
- [[Transformer 101系列] 初探LLM基座模型](https://zhuanlan.zhihu.com/p/640784855)
- [LLaMA2 RLHF 技术细节](https://zhuanlan.zhihu.com/p/644680366)
- [万字长文谈多模态预训练(UNITER、ViLBERT、CLIP、ALBEF、BLIP、METER)](https://zhuanlan.zhihu.com/p/539906825)
- [大模型中的分词器tokenizer:BPE、WordPiece、Unigram LM、SentencePiece](https://zhuanlan.zhihu.com/p/620508648)
- [【LLM系列】开源模型和闭源模型之争--写在LLaMA2 开源之后](https://zhuanlan.zhihu.com/p/644892671)
- [0718 - LLaMA2讨论 - Memo](https://d7mv45xi4m.feishu.cn/docx/OOhedFKGao2jlmxgsKGcCTnEnUc)
- [0723 - LLaMA 2 第二次讨论 - Memo](https://d7mv45xi4m.feishu.cn/docx/DOHIdmpbCoXhRwx62cCc3RcEnCh)
- [Bert/Transformer 被忽视的细节(或许可以用来做面试题)](https://zhuanlan.zhihu.com/p/559495068)
- [大模型面试八股](https://zhuanlan.zhihu.com/p/643560888)
- [降龙十八掌:这套优化transformer内存占用的组合技值得收藏](https://mp.weixin.qq.com/s/yNi1ehpHT8v2VnmNlZTBaw)
- [十分钟读懂旋转编码(RoPE)](https://zhuanlan.zhihu.com/p/647109286)
- [[LLM] multi query attention加速推理解码](https://zhuanlan.zhihu.com/p/647109286)
- [大模型(LLM) + 上下文检索增强](https://zhuanlan.zhihu.com/p/647112059)
- [语言模型的训练时间:从估算到 FLOPs 推导](https://zhuanlan.zhihu.com/p/646905171)
- [大模型基础|位置编码|RoPE|ALiBi](https://zhuanlan.zhihu.com/p/650469278)
- [RoPE外推的缩放法则 —— 尝试外推RoPE至1M上下文](https://zhuanlan.zhihu.com/p/660073229)
- [NTK-ALiBi:通过插值实现大模型ALiBi位置编码的长文本外推](https://zhuanlan.zhihu.com/p/647628295)
- [miniGPT-4的同期工作: 微软LLaVa模型论文笔记](https://zhuanlan.zhihu.com/p/625723805)
- [Function Call: Chat 应用的插件基石与交互技术的变革黎明](https://zhuanlan.zhihu.com/p/649766613)
- [关于 Llama 2 的一切资源,我们都帮你整理好了](https://zhuanlan.zhihu.com/p/650614370)
- [大模型升级与设计之道:ChatGLM、LLAMA、Baichuan及LLM结构解析](https://zhuanlan.zhihu.com/p/651747035)
- [如何评价超越Llama的Falcon模型?](https://www.zhihu.com/question/605021170/answer/3202176558)
- [From LLaMA2 to GPT4](https://zhuanlan.zhihu.com/p/645387165)
- [大杀器,多模态大模型MiniGPT-4入坑指南](https://zhuanlan.zhihu.com/p/627671257)
- [视觉Transformer如何优雅地避开位置编码?](https://www.zhihu.com/question/453193028/answer/3196023627)
- [动动嘴就可以创建专属的AI智能体小队,LinkSoul.AI、北大、港科大等发布AutoAgents技术](https://zhuanlan.zhihu.com/p/654238433)
- [MiniGPT-4模型原理及复现](https://zhuanlan.zhihu.com/p/637819943)
- [手把手教学!部署MiniGPT4模型](https://zhuanlan.zhihu.com/p/625152404)
- [LLM投机采样(Speculative Sampling)为何能加速模型推理](https://zhuanlan.zhihu.com/p/653734659)
- [LangChain之Memory](https://zhuanlan.zhihu.com/p/628734321)
- [LLM/阿里:通义千问Qwen-VL与Qwen-VL-Chat多模态大模型【对标VisualGLM】](https://zhuanlan.zhihu.com/p/652545086)
- [不用4个H100!340亿参数Code Llama在Mac可跑,每秒20个token,代码生成最拿手|Karpathy转赞](https://zhuanlan.zhihu.com/p/653729679)
- [超长上下文 LLM 推理简要分析](https://zhuanlan.zhihu.com/p/653375672)
- [LongMem: 大模型的长期记忆](https://zhuanlan.zhihu.com/p/642279963)
- [【LLM】Meta LLaMA 2中RLHF技术细节](https://zhuanlan.zhihu.com/p/644697081)
- [LLM大模型训练Trick系列(一)之拒绝采样](https://zhuanlan.zhihu.com/p/649731916)
- [想让大模型在prompt中学习更多示例,这种方法能让你输入更多字符](https://zhuanlan.zhihu.com/p/655965488)
- [主流大语言模型从预训练到微调的技术原理](https://zhuanlan.zhihu.com/p/651564985)
- [AI Agents大爆发:OpenAI的下一步](https://zhuanlan.zhihu.com/p/655560864)
- [小写一下llama2,破除迷信](https://zhuanlan.zhihu.com/p/655654221)
- [LLM评估指标困惑度的理解](https://zhuanlan.zhihu.com/p/651410752)
- [Anima新模型发布,100K窗口长度,突破极限,真的巨巨巨强大!长才是王道! ](https://mp.weixin.qq.com/s/e4qX3lIOp0-1_p4_2F53zA)
- [Mixture-of-Experts (MoE) 经典论文一览](https://zhuanlan.zhihu.com/p/542465517)
- [[LLM] 从实践到理论,Byte Pair Encoding(BPE) 深度调研](https://zhuanlan.zhihu.com/p/657938053)
- [理解NLP最重要的编码方式 — Byte Pair Encoding (BPE),这一篇就够了](https://zhuanlan.zhihu.com/p/424631681)
- [NLP三大Subword模型详解:BPE、WordPiece、ULM](https://zhuanlan.zhihu.com/p/191648421)
- [再读VIT,还有多少细节是你不知道的](https://zhuanlan.zhihu.com/p/657666107)
- [Transformer位置编码(基础)](https://zhuanlan.zhihu.com/p/631363482)
- [Llama 2 中使用 RLHF 的一些细节:margin r、reject sampling 和 PPO](https://zhuanlan.zhihu.com/p/660058778)
- [创造性vs确定性:大语言模型(LLM)中的温度(Temperature)和Top_P怎么调?](https://zhuanlan.zhihu.com/p/666315413)
- [如何混合大模型SFT阶段的各能力项数据?](https://zhuanlan.zhihu.com/p/662657529)
- [【llm大语言模型】一文看懂llama2(原理,模型,训练)](https://zhuanlan.zhihu.com/p/651248009)
- [如何更好地继续预训练(Continue PreTraining)](https://zhuanlan.zhihu.com/p/654463331)
- [[大模型推理][WINT8/4](00)🔥通俗易懂讲解-快速反量化算法](https://zhuanlan.zhihu.com/p/657072856)
- [Llama 2详解](https://zhuanlan.zhihu.com/p/649756898)
- [垂直领域大模型的思考](https://zhuanlan.zhihu.com/p/652645925)
- [解读 Effective Long Context Scaling of Foundation Models(强烈推荐)](https://zhuanlan.zhihu.com/p/666566126)
- [解析大模型中的Scaling Law](https://zhuanlan.zhihu.com/p/667489780)
- [NLP(廿三):LLM 中的长文本问题](https://zhuanlan.zhihu.com/p/640641794)
- [十分钟读懂Beam Search 1:基础](https://zhuanlan.zhihu.com/p/114669778)
- [颠覆Transformer霸权!CMU普林斯顿推Mamba新架构,解决致命bug推理速度暴增5倍](https://zhuanlan.zhihu.com/p/670490102)
- [矩阵模拟!Transformer大模型3D可视化,GPT-3、Nano-GPT每一层清晰可见](https://zhuanlan.zhihu.com/p/670287271)
- [旋转式位置编码 (RoPE) 知识总结](https://zhuanlan.zhihu.com/p/662790439)
- [大模型生成去重技术总结](https://zhuanlan.zhihu.com/p/659961396)
- [如何优雅地编码文本中的位置信息?三种positional encoding方法简述](https://zhuanlan.zhihu.com/p/121126531)
- [adam在大模型预训练中的不稳定性分析及解决办法](https://zhuanlan.zhihu.com/p/675421518)
- [饮鸩止渴?LLM训练要不要过采样/训多个epoch](https://zhuanlan.zhihu.com/p/671634621)
- [多个大语言微调模型并行推断的潜力](https://zhuanlan.zhihu.com/p/656344166)
- [剖析GPT推断中的批处理效应](https://zhuanlan.zhihu.com/p/630324993)
- [RoPE旋转位置编码深度解析:理论推导、代码实现、长度外推](https://zhuanlan.zhihu.com/p/645263524)
- [再论大模型位置编码及其外推性(万字长文)](https://zhuanlan.zhihu.com/p/675243992)
- [RoPE外推优化——支持192K上下文长度](https://zhuanlan.zhihu.com/p/678755776)
- [想研究大模型Alignment,你只需要看懂这几篇paper](https://zhuanlan.zhihu.com/p/681642685)
- [MiniCPM:揭示端侧大语言模型的无限潜力](https://shengdinghu.notion.site/MiniCPM-c805a17c5c8046398914e47f0542095a)
- [GPT-4内幕大泄露!1.8万亿巨量参数,13万亿token训练,斥资6300万美元](https://zhuanlan.zhihu.com/p/642902819)
- [一览大模型长文本能力](https://mp.weixin.qq.com/s/H0VwXlDz4SwA3D7hTgBPhw)
- [LLM(廿六):从信息论的角度解释 scaling law](https://zhuanlan.zhihu.com/p/687278237)
- [Mamba技术背景详解:从RNN到Mamba一文搞定!](https://zhuanlan.zhihu.com/p/689215356)
- [[大模型 08] 水多加面面多加水——参数量和数据的缩放定律](https://zhuanlan.zhihu.com/p/697473051)
- [GPT-4o解耦之旅](https://zhuanlan.zhihu.com/p/700092179)
- [CLA:降低Transformer模型内存需求的新方法](https://zhuanlan.zhihu.com/p/699863802)
- [为什么需要RLHF?SFT不够吗?](https://www.zhihu.com/question/651021172/answer/3513159005)
- [从Nemotron-4 看 Reward Model 发展趋势](https://zhuanlan.zhihu.com/p/703657164)
- [Cosmopedia: 如何为预训练构建大规模合成数据集](https://zhuanlan.zhihu.com/p/706832032)##### Agent
- [一个不是很长的综述:AI-Agent,Language Agent(语言代理,智能体)下一代语言大模型的发展](https://zhuanlan.zhihu.com/p/665355126)
- [NLP(廿二):LLM 时代的 multi-agent 系统](https://zhuanlan.zhihu.com/p/665644399)
- [关于 Agent 开发的一些思考](https://zhuanlan.zhihu.com/p/666401588)
- [AI Agent万字长文总结](https://zhuanlan.zhihu.com/p/662460753)##### 多模态
- [多模态大模型 CLIP, BLIP, BLIP2, LLaVA, miniGPT4, InstructBLIP 系列解读](https://zhuanlan.zhihu.com/p/653902791)
- [多模态大模型超详细解读 (目录)](https://zhuanlan.zhihu.com/p/625926419)
- [我们与 GPT-4V 的距离](https://zhuanlan.zhihu.com/p/686257072)
- [LLaVA(二)LLaVA-1.5 论文解读](https://zhuanlan.zhihu.com/p/696402890)##### 大模型训练和微调技术
- [Megatron-LM 分布式执行调研](https://strint.notion.site/Megatron-LM-86381cfe51184b9c888be10ee82f3812)
- [BLOOM 训练背后的技术](https://www.cnblogs.com/Matrix_Yao/p/17238627.html)
- [聊聊 PyTorch2.0 中新的Distributed API](https://mp.weixin.qq.com/s/hOOFE_eFD6a8GKTdnRcJXg)
- [聊聊 PyTorch 中新的Distributed API (二)](https://mp.weixin.qq.com/s/zDSuToVMo4iK3sxF662kvg)
- [【LLM】从零开始训练大模型](https://zhuanlan.zhihu.com/p/636270877)
- [在一张 24 GB 的消费级显卡上用 RLHF 微调 20B LLMs](https://www.cnblogs.com/huggingface/p/17245966.html)
- [人手一个ChatGPT!微软DeepSpeed Chat震撼发布,一键RLHF训练千亿级大模型](https://zhuanlan.zhihu.com/p/621379646)
- [大型语言模型(LLM)训练指南🚀](https://zhuanlan.zhihu.com/p/611325149)
- [“StackLLaMA”: 用 RLHF 训练 LLaMA 的手把手教程](https://zhuanlan.zhihu.com/p/626896135)
- [图解大模型训练之:流水线并行(Pipeline Parallelism),以Gpipe为例](https://zhuanlan.zhihu.com/p/613196255)
- [图解大模型训练之:数据并行上篇(DP, DDP与ZeRO)](https://zhuanlan.zhihu.com/p/617133971)
- [图解大模型训练之:数据并行下篇( DeepSpeed ZeRO,零冗余优化)](https://zhuanlan.zhihu.com/p/618865052)
- [图解大模型训练之:张量模型并行(TP),Megatron-LM](https://zhuanlan.zhihu.com/p/622212228)
- [Megatron-LM 中的 pipeline 并行](https://zhuanlan.zhihu.com/p/432969288)
- [图解大模型系列之:Megatron源码解读1,分布式环境初始化](https://zhuanlan.zhihu.com/p/629121480)
- [图解大模型训练之:Megatron源码解读2,模型并行](https://zhuanlan.zhihu.com/p/634377071)
- [聊聊序列并行Sequence parallelism](https://mp.weixin.qq.com/s/ylScQOpJ1-ufyPK7X6VUjw)
- [Megatron-LM 近期的改动](https://zhuanlan.zhihu.com/p/651192295)
- [深入理解 Megatron-LM(1)基础知识](https://zhuanlan.zhihu.com/p/650234985)
- [深入理解 Megatron-LM(2)原理介绍](https://zhuanlan.zhihu.com/p/650383289)
- [深入理解 Megatron-LM(3)代码结构](https://zhuanlan.zhihu.com/p/650237820)
- [深入理解 Megatron-LM(4)并行设置](https://zhuanlan.zhihu.com/p/650500590)
- [深入理解 Megatron-LM(5)张量并行](https://zhuanlan.zhihu.com/p/650237833)
- [聊聊字节 AML 万卡工作 MegaScale: Scaling Large Language Model Training](https://mp.weixin.qq.com/s/aXsURbHZKzoBw-ChaBnjEQ)
- [深度学习里,模型并行中怎么将模型拆分?](https://www.zhihu.com/question/319355346/answer/2985459442)
- [Transformers DeepSpeed官方文档](https://zhuanlan.zhihu.com/p/621572871)
- [当红炸子鸡 LoRA,是当代微调 LLMs 的正确姿势?](https://zhuanlan.zhihu.com/p/618894919)
- [GLM、LLAMA用Accelerate+deepspeed做RLHF时可能遇到的问题](https://zhuanlan.zhihu.com/p/629614251)
- [GPT fine-tune实战: 训练我自己的 ChatGPT🚀🚀🚀](https://zhuanlan.zhihu.com/p/616504594)
- [DeepSpeed之ZeRO系列:将显存优化进行到底](https://zhuanlan.zhihu.com/p/513571706)
- [大模型也内卷,Vicuna训练及推理指南,效果碾压斯坦福羊驼](https://zhuanlan.zhihu.com/p/624012908)
- [一键式 RLHF 训练 DeepSpeed Chat(一):理论篇](https://zhuanlan.zhihu.com/p/626159553)
- [使用DeepSpeed/P-Tuning v2对ChatGLM-6B进行微调](https://zhuanlan.zhihu.com/p/622351059)
- [从0到1基于ChatGLM-6B使用LoRA进行参数高效微调](https://zhuanlan.zhihu.com/p/621793987)
- [足够惊艳,使用Alpaca-Lora基于LLaMA(7B)二十分钟完成微调,效果比肩斯坦福羊驼](https://zhuanlan.zhihu.com/p/619426866)
- [基于LLaMA-7B/Bloomz-7B1-mt复现开源中文对话大模型BELLE及GPTQ量化](https://zhuanlan.zhihu.com/p/618876472)
- [从0到1复现斯坦福羊驼(Stanford Alpaca 7B)](https://zhuanlan.zhihu.com/p/618321077)
- [如何使用 Megatron-LM 训练语言模型](https://zhuanlan.zhihu.com/p/633160974)
- [[源码解析] 模型并行分布式训练Megatron (1) --- 论文&基础 ](https://juejin.cn/post/7057837676430360584)
- [[源码解析] 模型并行分布式训练Megatron (2) --- 整体架构 ](https://juejin.cn/post/7061942798957674504)
- [[源码解析] 模型并行分布式训练 Megatron (3) ---模型并行实现 ](https://juejin.cn/post/7062256365636419592)
- [[源码解析] 模型并行分布式训练 Megatron (4) --- 如何设置各种并行 ](https://juejin.cn/post/7063030243224879140)
- [[源码解析] 模型并行分布式训练Megatron (5) --Pipedream Flush ](https://juejin.cn/post/7064496967828635655)
- [模型并行训练:Megatron-LM pipeline并行源码解读](https://zhuanlan.zhihu.com/p/678724323)
- [Pytorch Distributed Data Parallal](https://fazzie-key.cool/2022/01/23/ddp/)
- [【分布式训练技术分享五】聊聊 Zero Bubble Pipeline Parallelism](https://zhuanlan.zhihu.com/p/670301574)
- [大模型参数高效微调技术原理综述(七)-最佳实践、总结](https://zhuanlan.zhihu.com/p/636999010)
- [【万字长文】LLaMA, ChatGLM, BLOOM的参数高效微调实践](https://zhuanlan.zhihu.com/p/635710004)
- [CPT:兼顾理解和生成的中文预训练模型](https://zhuanlan.zhihu.com/p/421402341)
- [大模型流水线并行(Pipeline)实战](https://zhuanlan.zhihu.com/p/636488690)
- [QLoRA:4-bit级别的量化+LoRA方法,用3090在DB-GPT上打造基于33B LLM的个人知识库](https://zhuanlan.zhihu.com/p/634516004)
- [大模型高效微调综述上:Adapter Tuning、AdaMix、PET、Prefix-Tuning、Prompt Tuning、P-tuning、P-tuning v2](https://zhuanlan.zhihu.com/p/638809556)
- [大模型高效微调综述下: DiffPruning、BitFit、LoRa、AdaLoRA、MAM Adapters、UniPELT](https://zhuanlan.zhihu.com/p/639068809)
- [RLHF实践中的框架使用与一些坑 (TRL, LMFlow)](https://zhuanlan.zhihu.com/p/636358058)
- [QLoRA: 4bit量化+LoRA训练=瞬间起飞](https://zhuanlan.zhihu.com/p/634256206)
- [baichuan-7B 模型使用/训练/Lora/测评](https://zhuanlan.zhihu.com/p/637343740)
- [LLM - finetuning - 踩坑经验之谈](https://zhuanlan.zhihu.com/p/639462205)
- [使用 RLHF 训练 LLaMA 的实践指南:StackLLaMA](https://zhuanlan.zhihu.com/p/631832914)
- [预训练模型时代:告别finetune, 拥抱adapter](https://zhuanlan.zhihu.com/p/451440421)
- [ChatGLM2微调保姆级教程~](https://zhuanlan.zhihu.com/p/641047705)
- [LLM训练指南:Token及模型参数准备](https://zhuanlan.zhihu.com/p/636812912)
- [单样本微调给ChatGLM2注入知识~](https://zhuanlan.zhihu.com/p/642357133)
- [想要微调清华chatglm6b模型,数据集给多少条比较合适?](https://www.zhihu.com/question/596950521/answer/3109759716)
- [如何看待chatglm2?真实效果怎么样?](https://www.zhihu.com/question/608702606/answer/3118275498)
- [百川13B-chat开箱及LORA进行PT/SFT微调](https://zhuanlan.zhihu.com/p/643021523)
- [打造 LLM 界的 Web UI:24GB 显卡训练百亿大模型](https://zhuanlan.zhihu.com/p/645010851)
- [大模型训练 Pipeline Parallel 流水并行性能分析](https://zhuanlan.zhihu.com/p/618590870)
- [【LLM系列】中文LLaMA2的一些工作](https://zhuanlan.zhihu.com/p/647388816)
- [LLaMA2中文微调](https://zhuanlan.zhihu.com/p/646811859)
- [图解大模型微调系列之:大模型低秩适配器LoRA(原理篇)](https://zhuanlan.zhihu.com/p/646831196)
- [大模型参数高效微调技术实战(二)-Prompt Tuning](https://zhuanlan.zhihu.com/p/646748939)
- [[调研]Megatron-LM 的分布式执行](https://strint.notion.site/Megatron-LM-86381cfe51184b9c888be10ee82f3812#720aad004d8241d9ae500ba39b545517)
- [深入理解 Megatron-LM(5)模型并行](https://zhuanlan.zhihu.com/p/650237833)
- [GPT-3模型为何难以复现?这也许是分布式AI框架的最优设计](https://cloud.tencent.com/developer/article/1832354)
- [北大硕士RLHF实践,基于DeepSpeed-Chat成功训练上自己的模型](https://mp.weixin.qq.com/s/OKaWJcbBH0Fjmu-fiB_Z9w)
- [Megatron-LM 第三篇Paper总结——Sequence Parallelism & Selective Checkpointing](https://zhuanlan.zhihu.com/p/522198082)
- [【llm大语言模型】code llama详解与应用](https://zhuanlan.zhihu.com/p/652855450)
- [DeepSpeed-Chat更新: Llama/Llama-2系统支持,效率提升和训练稳定性改进](https://zhuanlan.zhihu.com/p/653631374)
- [RLHF实践](https://zhuanlan.zhihu.com/p/635569455)
- [LLM - finetuning - 踩坑经验之谈](https://zhuanlan.zhihu.com/p/639462205)
- [从头训练一个迷你中文版Llama2--一个小项目踏上LLM之旅](https://zhuanlan.zhihu.com/p/652664029)
- [用 Decision Transformer/Offline RL 做 LLM Alignment](https://zhuanlan.zhihu.com/p/652335046)
- [代码生成模型评价指标 pass@k 的计算](https://zhuanlan.zhihu.com/p/653063532)
- [BaiChuan2技术报告细节分享&个人想法](https://zhuanlan.zhihu.com/p/656570703)
- [大模型参数高效微调技术实战(六)-IA3](https://zhuanlan.zhihu.com/p/649707359)
- [图解大模型微调系列之:AdaLoRA,能做“财务”预算的低秩适配器](https://zhuanlan.zhihu.com/p/657130029)
- [【2023Q4】再谈Long-Context LLM](https://zhuanlan.zhihu.com/p/660660723)
- [【大语言模型】LongLoRA:大语言模型长文本的高效微调方法](https://zhuanlan.zhihu.com/p/658067243)
- [RLHF 训练中,如何挑选最好的 checkpoint?](https://zhuanlan.zhihu.com/p/664575712)
- [deepspeed入门教程](https://zhuanlan.zhihu.com/p/630734624)
- [S-LORA:单卡服务两千个LLM模型,vLLM团队指出行业大模型新范式](https://zhuanlan.zhihu.com/p/667213961)
- [大模型微调技巧 | 高质量指令数据筛选方法-MoDS](https://zhuanlan.zhihu.com/p/671183709)
- [2023年神秘而难以理解的大模型强化学习技术:RLHF PPO,DPO,以及InstructGPT,DeepSpeed-Chat, LLama2,Baichuan2的RLHF](https://zhuanlan.zhihu.com/p/662753985)
- [影响PPO算法性能的10个关键技巧(附PPO算法简洁Pytorch实现)](https://zhuanlan.zhihu.com/p/512327050)
- [Transformer的浮点数计算](https://zhuanlan.zhihu.com/p/670583522)
- [ChatGLM3保姆级P-Tuning v2微调教程](https://zhuanlan.zhihu.com/p/670248457)
- [使用 PyTorch 完全分片数据并行技术加速大模型训练](https://zhuanlan.zhihu.com/p/670374745)
- [显存优化之加速通信算子内存释放](https://zhuanlan.zhihu.com/p/671834539)
- [Transformer第四章:vllm之PagedAttention代码分析(2)](https://zhuanlan.zhihu.com/p/663719053)
- [探索大模型SFT过程中的不稳定的原因](https://zhuanlan.zhihu.com/p/669976120)
- [【手撕RLHF-Rejection Sampling】如何优雅的从SFT过渡到PPO](https://zhuanlan.zhihu.com/p/669397860)
- [数据并行Deep-dive: 从DP 到 Fully Sharded Data Parallel (FSDP)完全分片数据并行](https://zhuanlan.zhihu.com/p/485208899)
- [ChatGLM2-6B多轮对话训练方式](https://zhuanlan.zhihu.com/p/651293366)
- [显存优化之重计算在长文场景的思考](https://zhuanlan.zhihu.com/p/675677945)
- [一文读懂分布式训练启动方式](https://zhuanlan.zhihu.com/p/675464874)
- [DeepSpeed ZeRO理论与VLM大模型训练实践](https://zhuanlan.zhihu.com/p/675360966)
- [LLM中的RLHF——ppo、dpo算法实践(基于qwen、chatglm3)](https://zhuanlan.zhihu.com/p/675215827)
- [使用Firefly在单卡V100上对Qwen1.5进行SFT和DPO,大幅超越Qwen1.5和Gemma](https://zhuanlan.zhihu.com/p/692871243)
- [DeepSpeed-Ulysses (SequenceParallel)](https://zhuanlan.zhihu.com/p/659198439)
- [NLP(九十六)使用LLaMA-Factory实现function calling](https://zhuanlan.zhihu.com/p/694577892)
- [不那么显然的 RLHF](https://zhuanlan.zhihu.com/p/642385494)
- [分布式训练与DeepSpeed浅谈](https://zhuanlan.zhihu.com/p/699572987)
- [序列并行做大模型训练,你需要知道的六件事](https://zhuanlan.zhihu.com/p/698031151)
- [我爱DeepSpeed-Ulysses:重新审视大模型序列并行技术](https://zhuanlan.zhihu.com/p/703669087)
- [由Ring-Attention性能问题引发的计算通信overlap分析](https://zhuanlan.zhihu.com/p/706805407)
- [为Token-level流水并行找PMF:从TeraPipe,Seq1F1B,HPipe到PipeFusion](https://zhuanlan.zhihu.com/p/706475158)
- [SFT Packing详解](https://zhuanlan.zhihu.com/p/707329908)##### 大模型推理技术
- [聊聊大模型推理服务中的优化问题](https://zhuanlan.zhihu.com/p/677650022)
- [聊聊大模型推理中的分离式推理](https://zhuanlan.zhihu.com/p/706469785)
- [大幅优化推理过程,字节高性能Transformer推理库获IPDPS 2023最佳论文奖 ](https://mp.weixin.qq.com/s/5TM4PXTUBZuOfZlltFfrsQ)
- [CodeGeeX百亿参数大模型的调优笔记:比FasterTransformer更快的解决方案](https://zhuanlan.zhihu.com/p/617027615)
- [优化故事: BLOOM 模型推理](https://mp.weixin.qq.com/s/yzVqh4d6ynNROJxHycDUXg)
- [大型语言模型的推理演算](https://mp.weixin.qq.com/s/2wfUQNsH4IRuJEF39mebUQ)
- [简单读读WeightOnly](https://zhuanlan.zhihu.com/p/622334595)
- [[大模型技术祛魅]关于FlexGen的一点理解](https://zhuanlan.zhihu.com/p/610853654)
- [LLM Inference CookBook(持续更新)](https://zhuanlan.zhihu.com/p/619596323)
- [优化故事: BLOOM 模型推理](https://mp.weixin.qq.com/s/yzVqh4d6ynNROJxHycDUXg)
- [GPTQ-for-LLaMa 量化分析和优化](https://zhuanlan.zhihu.com/p/625701227)
- [Web-LLM:机器学习编译纯浏览器运行大模型](https://zhuanlan.zhihu.com/p/622271247)
- [陈天奇等人新作引爆AI界:手机原生跑大模型,算力不是问题了](https://mp.weixin.qq.com/s/uQGAu1v-6ApgZHVkZJsUdQ)
- [NLP(十一):大语言模型的模型量化(INT8)技术](https://zhuanlan.zhihu.com/p/627436535)
- [大(语言)模型推理原理及加速](https://zhuanlan.zhihu.com/p/628511161)
- [AI算力碎片化:矩阵乘法的启示](https://zhuanlan.zhihu.com/p/624425308)
- [大大大模型部署方案抛砖引玉](https://mp.weixin.qq.com/s/e6ymQZs5MY1pdodC7eg8iQ)
- [BELLE(LLaMA-7B/Bloomz-7B1-mt)大模型使用GPTQ量化后推理性能测试](https://zhuanlan.zhihu.com/p/621128368)
- [大模型的好伙伴,浅析推理加速引擎FasterTransformer](https://zhuanlan.zhihu.com/p/626008090)
- [模型推理服务化框架Triton保姆式教程(一):快速入门](https://zhuanlan.zhihu.com/p/629336492)
- [模型推理服务化框架Triton保姆式教程(二):架构解析](https://zhuanlan.zhihu.com/p/634143650)
- [模型推理服务化框架Triton保姆式教程(三):开发实践](https://zhuanlan.zhihu.com/p/634444666)
- [【自然语言处理】【大模型】大语言模型BLOOM推理工具测试](https://zhuanlan.zhihu.com/p/608004441)
- [使用bitsandbytes、4 位量化和 QLoRA 使 LLM 更易于访问](https://zhuanlan.zhihu.com/p/632287465)
- [NLP(十七):从 FlashAttention 到 PagedAttention, 如何进一步优化 Attention 性能](https://zhuanlan.zhihu.com/p/638468472)
- [【LLM 加速技巧】Muti Query Attention 和 Attention with Linear Bias(附源码)](https://zhuanlan.zhihu.com/p/634236135)
- [如何优化transformer的attention?](https://www.zhihu.com/question/602057035/answer/3046820082)
- [Huggingface Accelerate文档:超大模型推理方法](https://zhuanlan.zhihu.com/p/606061177)
- [vLLM框架top down概览](https://zhuanlan.zhihu.com/p/645251151)
- [LLaMa 量化部署](https://zhuanlan.zhihu.com/p/641641929)
- [为什么现在大家都在用 MQA 和 GQA?](https://zhuanlan.zhihu.com/p/647130255)
- [小记:主流推理框架在Llama 2 的上性能比较](https://zhuanlan.zhihu.com/p/646772063)
- [vllm vs TGI 部署 llama v2 7B 踩坑笔记](https://zhuanlan.zhihu.com/p/645732302)
- [TGI + exllama - llama 量化部署方案](https://zhuanlan.zhihu.com/p/646716367)
- [BELLE(LLaMA-7B/Bloomz-7B1-mt)大模型使用GPTQ量化后推理性能测试](https://zhuanlan.zhihu.com/p/621128368)
- [QLoRA、GPTQ:模型量化概述](https://zhuanlan.zhihu.com/p/646210009)
- [LLM推理性能优化探索](https://zhuanlan.zhihu.com/p/653735572)
- [CNN量化 vs. LLM量化](https://zhuanlan.zhihu.com/p/645362500)
- [LLM大语言模型之Generate/Inference(生成/推理)中参数与解码策略原理及其代码实现](https://zhuanlan.zhihu.com/p/653926703)
- [NLP(十八):LLM 的推理优化技术纵览](https://zhuanlan.zhihu.com/p/642412124)
- [LLM推理部署(一):LLM七种推理服务框架总结](https://zhuanlan.zhihu.com/p/653352979)
- [LLM系列笔记:LLM Inference量化分析与加速](https://zhuanlan.zhihu.com/p/642272677)
- [在大模型推理方面,有哪些研究热点?](https://www.zhihu.com/question/588122011/answer/3207992049)
- [LLM推理加速-Medusa](https://zhuanlan.zhihu.com/p/655809033)
- [PagedAttention--大模型推理服务框架vLLM要点简析 (中)](https://zhuanlan.zhihu.com/p/655561941)
- [[LLM]KV cache详解 图示,显存,计算量分析,代码](https://zhuanlan.zhihu.com/p/646577898)
- [LLM推理优化技术综述:KVCache、PageAttention、FlashAttention、MQA、GQA](https://zhuanlan.zhihu.com/p/655325832)
- [大规模 Transformer 模型 8 比特矩阵乘简介 - 基于 Hugging Face Transformers、Accelerate 以及 bitsandbytes](https://zhuanlan.zhihu.com/p/624929178)
- [使用bitsandbytes、4 位量化和 QLoRA 使 LLM 更易于访问](https://zhuanlan.zhihu.com/p/632287465)
- [ByteTransformer源码解析](https://zhuanlan.zhihu.com/p/656342974)
- [LLM推理加速的文艺复兴:Noam Shazeer和Blockwise Parallel Decoding](https://zhuanlan.zhihu.com/p/658298728)
- [LLM大模型之不同精度下显存占用与相互转换实践](https://zhuanlan.zhihu.com/p/658343628)
- [CUDA PagedAttention kernel源码解析--大模型推理服务框架vLLM要点简析(下)](https://zhuanlan.zhihu.com/p/658233994)
- [[vllm]kernels分析](https://zhuanlan.zhihu.com/p/657114963)
- [LLM大模型之精度问题(FP16,FP32,BF16)详解与实践](https://zhuanlan.zhihu.com/p/657886517)
- [PAI BladeLLM推理引擎: 超长上下文、更高性能](https://zhuanlan.zhihu.com/p/657187638)
- [大语言模型推理性能优化综述](https://zhuanlan.zhihu.com/p/656485997)
- [大模型推理优化--Prefill阶段seq_q切分](https://zhuanlan.zhihu.com/p/658443665)
- [LLM大语言模型之Generate/Inference(生成/推理)中参数与解码策略原理及其代码实现](https://zhuanlan.zhihu.com/p/653926703)
- [NLP(二十):漫谈 KV Cache 优化方法,深度理解 StreamingLLM](https://zhuanlan.zhihu.com/p/659770503)
- [【小白学习笔记】FP8 量化基础 - 英伟达](https://zhuanlan.zhihu.com/p/619431625)
- [大语言模型量化相关技术](https://zhuanlan.zhihu.com/p/664054739)
- [LLM Decoding Attention-KV Cache Int8量化](https://zhuanlan.zhihu.com/p/665474143)
- [大模型推理-TensorRT-LLM初探(一)运行llama,以及triton tensorrt llm backend](https://zhuanlan.zhihu.com/p/665209786)
- [llama.cpp源码解析--CUDA流程版本](https://zhuanlan.zhihu.com/p/665027154)
- [多个大语言微调模型并行推断的潜力](https://zhuanlan.zhihu.com/p/656344166)
- [DeepSpeed-FastGen:通过 MII 和 DeepSpeed-Inference 实现 LLM 高吞吐量文本生成](https://zhuanlan.zhihu.com/p/665494115)
- [关于大模型推理的量化算法总结](https://zhuanlan.zhihu.com/p/645308698)
- [Triton部署TensorRT-LLM](https://zhuanlan.zhihu.com/p/663378231)
- [Nvidia CUDA Core-LLM Decoding Attention推理优化](https://zhuanlan.zhihu.com/p/664348092)
- [【模型推理】谈谈为什么卷积加速更喜欢 NHWC Layout](https://zhuanlan.zhihu.com/p/395810743)
- [ChatGLM3 的工具调用(FunctionCalling)实现原理](https://zhuanlan.zhihu.com/p/664233831)
- [DeepSpeed Inference中的kernel优化](https://zhuanlan.zhihu.com/p/667329815)
- [【手撕LLM-投机解码】大模型迈向"并行"解码时代](https://zhuanlan.zhihu.com/p/671432448)
- [一行代码加速28倍大模型推理速度](https://zhuanlan.zhihu.com/p/670891343)
- [Continuous Batching:一种提升 LLM 部署吞吐量的利器](https://zhuanlan.zhihu.com/p/657586838)
- [大语言模型推理加速技术:计算加速篇](https://zhuanlan.zhihu.com/p/666452391)
- [不到1000行代码,PyTorch团队让Llama 7B提速10倍](https://zhuanlan.zhihu.com/p/670506844)
- [笔记:DeepSpeed inference 代码理解](https://zhuanlan.zhihu.com/p/668181423)
- [大模型推理核心技术之Continuous Batching和我的WXG往事](https://zhuanlan.zhihu.com/p/676109470)
- [论文笔记:DejaVu、LLM in Flash、PowerInfer](https://zhuanlan.zhihu.com/p/675585887)
- [TensorRT-LLM 如何加速推理之 -- Batching](https://zhuanlan.zhihu.com/p/675726439)
- [[ICML'23] DejaVu:LLM中的动态剪枝](https://zhuanlan.zhihu.com/p/673848224)
- [笔记:Llama.cpp 代码浅析(一):并行机制与KVCache](https://zhuanlan.zhihu.com/p/670515231)
- [LLM推理百倍加速之稀疏篇](https://zhuanlan.zhihu.com/p/677948929)
- [vLLM-prefix浅析(System Prompt,大模型推理加速)](https://zhuanlan.zhihu.com/p/678256296)
- [Text Generation Inference源码解读(一):架构设计与业务逻辑](https://zhuanlan.zhihu.com/p/672925155)
- [Text Generation Inference源码解读(二):模型加载与推理](https://zhuanlan.zhihu.com/p/675292919)
- [Weight Only Quantization 的性能优化](https://zhuanlan.zhihu.com/p/687844000)
- [LLM推理加速(三):AWQ量化](https://zhuanlan.zhihu.com/p/685867596)
- [OmniQuant-目前最优的LLM PTQ量化算法](https://zhuanlan.zhihu.com/p/687653912)
- [W4A16模型量化大法 AWQ](https://zhuanlan.zhihu.com/p/682041025)
- [大模型推理框架 vLLM 源码解析(二):Block 模块分配和管理](https://zhuanlan.zhihu.com/p/688660090)
- [FP8量化解读--8bit下最优方案?(一)](https://zhuanlan.zhihu.com/p/565021881)
- [LLM PTQ量化经典研究解析](https://zhuanlan.zhihu.com/p/695267503)
- [GPTQ & SmoothQuant & AWQ 代码解析](https://zhuanlan.zhihu.com/p/697860995)
- [深入理解AWQ量化技术](https://zhuanlan.zhihu.com/p/697761176)
- [FP8 量化-原理、实现与误差分析](https://zhuanlan.zhihu.com/p/574825662)
- [从continuous batching到vLLM中的batching](https://zhuanlan.zhihu.com/p/688551989)##### 扩散模型
- [都2023年了,我不允许你还不懂DDPM!](https://zhuanlan.zhihu.com/p/663880249)
- [Kandinsky-3:最大的开源文生图模型](https://zhuanlan.zhihu.com/p/668853830)
- [视频生成迎来SD时代:Stable Video Diffusion开源了!](https://zhuanlan.zhihu.com/p/668100036)
- [一文带你看懂DDPM和DDIM(含原理简易推导,pytorch代码)](https://zhuanlan.zhihu.com/p/666552214)
- [AIGC优质模型导读:数据为王DALL-E 3](https://zhuanlan.zhihu.com/p/669578590)
- [SDXL Turbo来了:一步生成高质量图像](https://zhuanlan.zhihu.com/p/669353808)
- [十分钟读懂Diffusion:图解Diffusion扩散模型](https://zhuanlan.zhihu.com/p/599887666)
- [Stable Diffusion生图越来越快,TensorRT扩展实现SD秒速生图](https://zhuanlan.zhihu.com/p/668632473)
- [stable diffusion中Lora的原理和实践](https://zhuanlan.zhihu.com/p/662253917)
- [深入浅出完整解析Stable Diffusion XL(SDXL)核心基础知识](https://zhuanlan.zhihu.com/p/643420260)
- [大模型推理加速-Decoding Attention优化](https://zhuanlan.zhihu.com/p/672290443)
- [新一代文生图模型Stable Cascade来了](https://zhuanlan.zhihu.com/p/682257045)
- [基于扩散的生成模型架构理论综述](https://zhuanlan.zhihu.com/p/683813264)
- [深入浅出完整解析Stable Diffusion(SD)核心基础知识](https://zhuanlan.zhihu.com/p/632809634)
- [DALL-E 3技术报告阅读笔记](https://zhuanlan.zhihu.com/p/662745543)
- [Scalable Diffusion Models with Transformers(DiTs)论文阅读 -- 文生视频Sora模型基础结构DiT](https://zhuanlan.zhihu.com/p/597695487)
- [一文读懂DDIM凭什么可以加速DDPM的采样效率](https://zhuanlan.zhihu.com/p/627616358)
- [Stable Diffusion 中的自注意力替换技术与 Diffusers 实现](https://mp.weixin.qq.com/s/dF0ykeSYSM7kzUHe1kMxAg)
- [从continuous batching到vLLM中的batching](https://zhuanlan.zhihu.com/p/688551989)
- [图解大模型计算加速系列:分离式推理架构1,从DistServe谈起](https://zhuanlan.zhihu.com/p/706761664)
- [[LLM性能优化]聊聊长文本推理性能优化方向](https://zhuanlan.zhihu.com/p/698308542)##### 文生视频
- [Datawhale AI视频生成学习](https://datawhaler.feishu.cn/docx/G4LkdaffWopVbwxT1oHceiv9n0c)
- [OpenAI Sora背后的技术架构](https://zhuanlan.zhihu.com/p/683002680)
- [从零实现CLIP模型](https://zhuanlan.zhihu.com/p/676480190)
- [CLIP 模型解读](https://zhuanlan.zhihu.com/p/646790176)
- [Sora 技术解读(附带 DiT 模型详解)](https://zhuanlan.zhihu.com/p/683184325)
- [OpenAI 的视频生成大模型Sora的核心技术详解(一):Diffusion模型原理和代码详解](https://zhuanlan.zhihu.com/p/683418039)
- [DiT详解](https://zhuanlan.zhihu.com/p/683612528)
- [Diffusion Transformer Family:关于Sora和Stable Diffusion 3你需要知道的一切](https://zhuanlan.zhihu.com/p/684448966)
- [聊聊 DiT 和 GenTron](https://mp.weixin.qq.com/s/GcUqBlt47ntc-ttsfbgh4A)
- [OpenAI 视频模型 Sora 科研贡献速览](https://mp.weixin.qq.com/s/t9ZqzwMGePrmkpmw4XBJQA)
- [技术神秘化的去魅:Sora关键技术逆向工程图解](https://zhuanlan.zhihu.com/p/687928845)
- [Stable Video 3D震撼登场:单图生成无死角3D视频、模型权重开放](https://zhuanlan.zhihu.com/p/688112512)
- [PipeFusion:如何用PCIe互联GPU 低成本并行推理扩散模型](https://zhuanlan.zhihu.com/p/699612077)##### 强化学习
- [聊聊GRPO算法——从Open R1来看如何训练DeepSeek R1模型](https://www.cnblogs.com/zhiyong-ITNote/p/18702470)
##### 大模型服务
- [Gradio:轻松实现AI算法可视化部署](https://zhuanlan.zhihu.com/p/374238080)
- [vllm vs TGI 部署 llama v2 7B 踩坑笔记](https://zhuanlan.zhihu.com/p/645732302)##### Agent
- [Agent is all you need | AI智能体前沿进展总结](https://zhuanlan.zhihu.com/p/655425020)
- [Qwen 7B大模型ReAct Prompt详解以及LLM 智能体Agent实战](https://zhuanlan.zhihu.com/p/664477178)
- [开源大语言模型作为 LangChain 智能体](https://zhuanlan.zhihu.com/p/683464443)##### 大模型数据处理
- [详谈大模型训练中的数据收集、处理与模型影响:A Survey of Large Language Models工作中的数据总结](https://mp.weixin.qq.com/s/bHsb631KA5AaulBHNT5m9w)
- [过去三个月,LLaMA系模型发展如何?指令微调的核心问题又是什么? ](https://mp.weixin.qq.com/s/cXPNyOeK9vFjJcgxc_LqZQ)
- [cc_cleaner │ 一种丝滑高效且易扩展的数据清洗流程](https://mp.weixin.qq.com/s/D48Z8x_8jD4Dfd2tYdFa7g)
- [BigCode 背后的大规模数据去重](https://zhuanlan.zhihu.com/p/644900514)
- [LLM数据为王: Textbooks Are All You Need](https://zhuanlan.zhihu.com/p/642684154)##### 大模型评测
- [“评测即科学”:首篇大语言模型评测的综述,一文带你全面了解大模型评测的现状、方法和挑战](https://zhuanlan.zhihu.com/p/642689101)
- [开源模型离GPT-4有多远,OpenCompass LLM评测 8月榜单新鲜出炉](https://zhuanlan.zhihu.com/p/653577364)
- [关于openCompass与大模型评测现状的分析](https://zhuanlan.zhihu.com/p/652688939)##### 李沐论文精度文字版专栏
- [李沐论文精度文字版专栏](https://www.zhihu.com/column/c_1656053216138719233)
##### cursor 充值教程
https://chatgpi.cn/how-subscribe-pay-cursor-pro/
## Star History