Ecosyste.ms: Awesome
An open API service indexing awesome lists of open source software.
https://github.com/dipanjans/low_code_machine_learning_pycaret_workshop_2022
This workshop was done as a part of the 1729 conference organized by Fractal Analytics and Analytics Vidhya. Key content covered was hands-on notebooks leveraging PyCaret to compare, build, tune, evaluate and interpret machine learning models
https://github.com/dipanjans/low_code_machine_learning_pycaret_workshop_2022
Last synced: 4 months ago
JSON representation
This workshop was done as a part of the 1729 conference organized by Fractal Analytics and Analytics Vidhya. Key content covered was hands-on notebooks leveraging PyCaret to compare, build, tune, evaluate and interpret machine learning models
- Host: GitHub
- URL: https://github.com/dipanjans/low_code_machine_learning_pycaret_workshop_2022
- Owner: dipanjanS
- License: gpl-3.0
- Created: 2022-07-07T12:57:48.000Z (over 2 years ago)
- Default Branch: main
- Last Pushed: 2022-07-07T13:33:36.000Z (over 2 years ago)
- Last Synced: 2024-10-04T13:39:40.970Z (5 months ago)
- Language: Jupyter Notebook
- Size: 3.86 MB
- Stars: 21
- Watchers: 2
- Forks: 2
- Open Issues: 0
-
Metadata Files:
- Readme: README.md
- License: LICENSE
Awesome Lists containing this project
README
# Low-code and Auto-ML with PyCaret
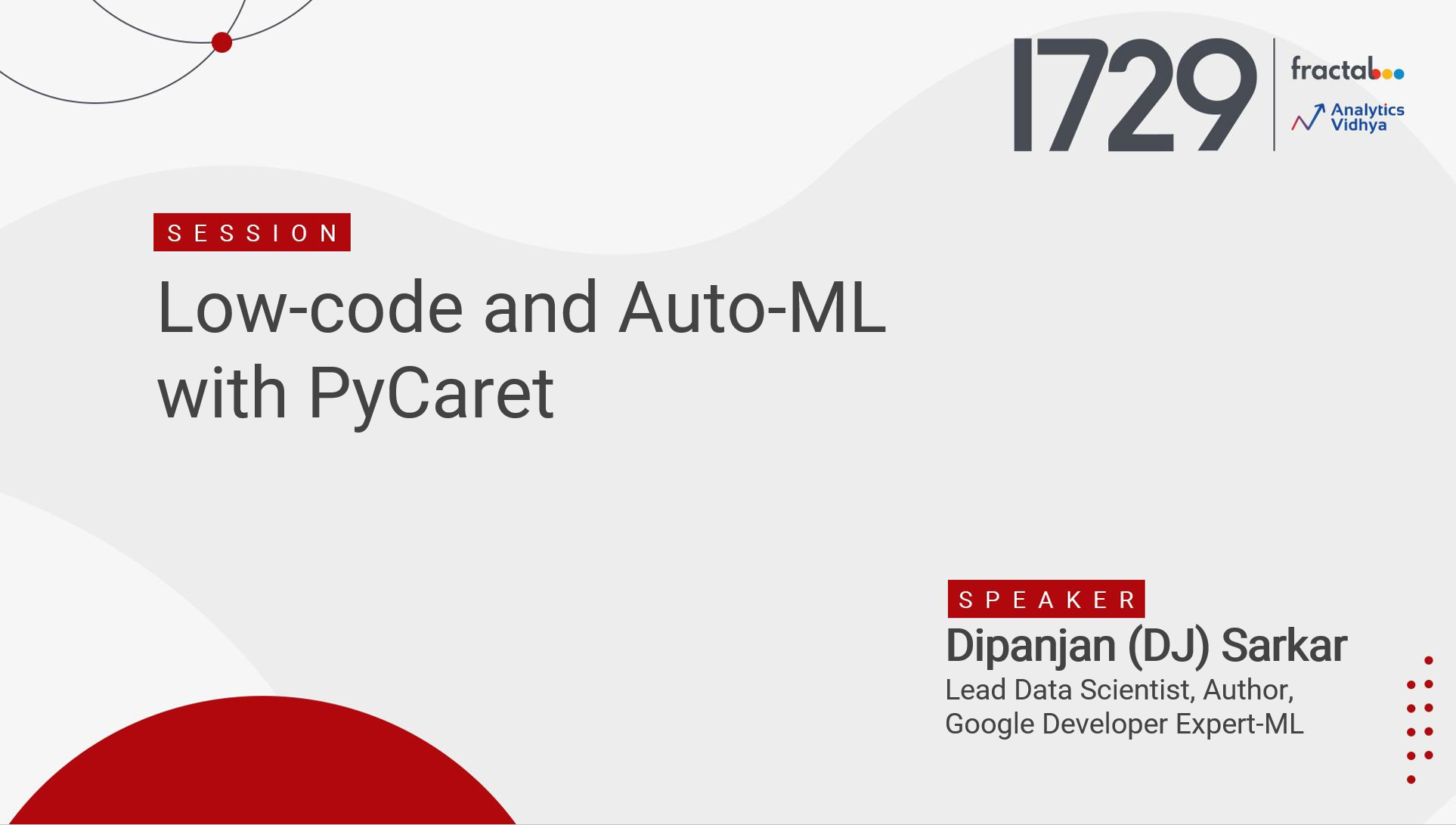This workshop was done as a part of the 1729 conference organized by Fractal Analytics and Analytics Vidhya. Key content covered was hands-on notebooks leveraging PyCaret to compare, build, tune, evaluate and interpret machine learning models.
## Session Outline
Low-code and Auto-ML are essential tools in a data scientist’s toolbox to improve productivity and iterate faster in data science projects. This hands-on workshop will focus on two major notebooks:
- __A Kickstarter notebook__ leveraging the popular open-source library PyCaret and go through learning the essentials:
- Setup experiments
- Train ML models
- Compare ML models
- Tune ML models
- Evaluate models
- Predictions on new data.- __An advanced notebook__ where we learn advanced capabilities of PyCaret including the following:
- Data transformations
- Imputation
- Multicollinearity
- Handling imbalanced data
- Advanced tuning methods
- Ensembling (Bagging, Boosting, Stacking, Blending)
- Explainable AI to interpret ML Models.__Recommendation:__ Please open the notebooks in Google Colab
## Author
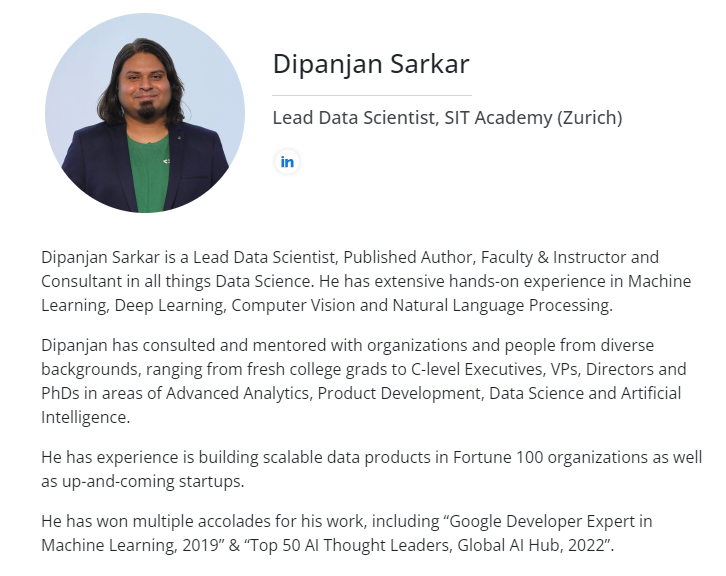
## Credits
Thanks to [PyCaret](https://pycaret.org), [Moez](https://www.linkedin.com/in/profile-moez) and the awesome documentation.