https://github.com/dselivanov/text2vec
Fast vectorization, topic modeling, distances and GloVe word embeddings in R.
https://github.com/dselivanov/text2vec
glove latent-dirichlet-allocation natural-language-processing text-mining topic-modeling vectorization word-embeddings word2vec
Last synced: 13 days ago
JSON representation
Fast vectorization, topic modeling, distances and GloVe word embeddings in R.
- Host: GitHub
- URL: https://github.com/dselivanov/text2vec
- Owner: dselivanov
- License: other
- Created: 2015-08-05T06:09:14.000Z (over 9 years ago)
- Default Branch: master
- Last Pushed: 2024-08-16T02:54:30.000Z (8 months ago)
- Last Synced: 2025-04-06T08:34:23.581Z (15 days ago)
- Topics: glove, latent-dirichlet-allocation, natural-language-processing, text-mining, topic-modeling, vectorization, word-embeddings, word2vec
- Language: R
- Homepage: http://text2vec.org
- Size: 46.2 MB
- Stars: 860
- Watchers: 53
- Forks: 133
- Open Issues: 25
-
Metadata Files:
- Readme: README.md
- Changelog: NEWS.md
- License: LICENSE
Awesome Lists containing this project
- awesome-nlp - text2vec - R 中的快速矢量化,主題建模,距離和 GloVe 字嵌入。 (函式庫 / 書籍)
README
**text2vec** is an R package which provides an efficient framework with a concise API for text analysis and natural language processing (NLP).
Goals which we aimed to achieve as a result of development of `text2vec`:
* **Concise** - expose as few functions as possible
* **Consistent** - expose unified interfaces, no need to explore new interface for each task
* **Flexible** - allow to easily solve complex tasks
* **Fast** - maximize efficiency per single thread, transparently scale to multiple threads on multicore machines
* **Memory efficient** - use streams and iterators, not keep data in RAM if possibleSee [API](http://text2vec.org/api.html) section for details.
# Performance
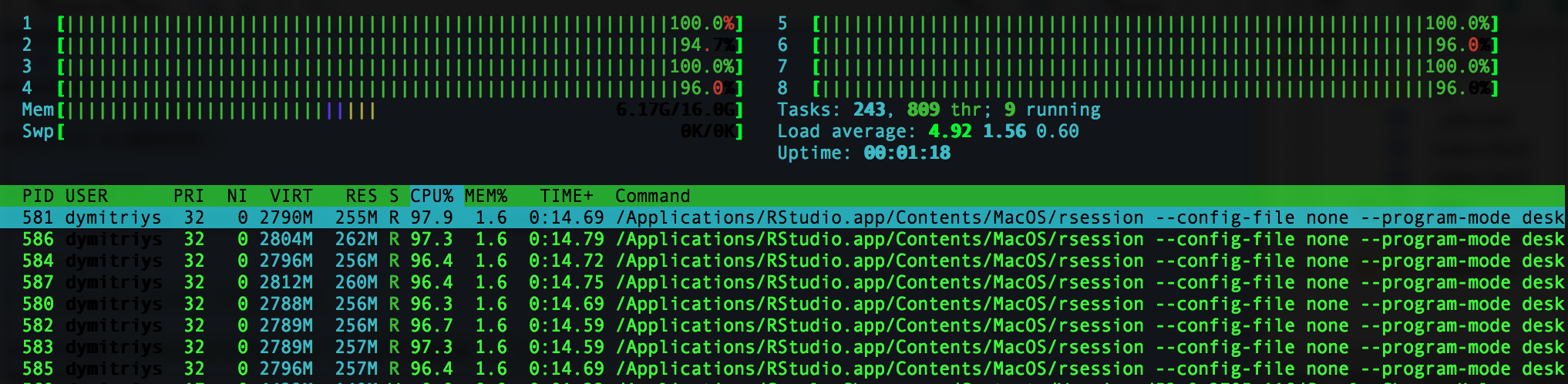
This package is efficient because it is carefully written in C++, which also means that text2vec is memory friendly. Some parts are fully parallelized using OpenMP.
Other emrassingly parallel tasks (such as vectorization) can use any fork-based parallel backend on UNIX-like machines. They can achieve near-linear scalability with the number of available cores.
Finally, a streaming API means that users do not have to load all the data into RAM.
# Contributing
The package has [issue tracker on GitHub](https://github.com/dselivanov/text2vec/issues) where I'm filing feature requests and notes for future work. Any ideas are appreciated.
Contributors are welcome. You can help by:
- testing and leaving feedback on the [GitHub issuer tracker](https://github.com/dselivanov/text2vec/issues) (preferably) or directly by e-mail
- forking and contributing (check [code our style guide](https://github.com/dselivanov/text2vec/wiki/Code-style-guide)). Vignettes, docs, tests, and use cases are very welcome
- by giving me a star on [project page](https://github.com/dselivanov/text2vec) :-)# License
GPL (>= 2)