https://github.com/si-ddhartha/cat-vs-non-cat-nn
Implemented an L-layer neural network to classify images as being of a cat or not from scratch without using any deep learning libraries like TensorFlow or PyTorch
https://github.com/si-ddhartha/cat-vs-non-cat-nn
classifier deep-learning l-layer-neural-network neural-network
Last synced: 13 days ago
JSON representation
Implemented an L-layer neural network to classify images as being of a cat or not from scratch without using any deep learning libraries like TensorFlow or PyTorch
- Host: GitHub
- URL: https://github.com/si-ddhartha/cat-vs-non-cat-nn
- Owner: Si-ddhartha
- Created: 2023-05-02T11:31:06.000Z (about 2 years ago)
- Default Branch: main
- Last Pushed: 2023-05-02T11:44:18.000Z (about 2 years ago)
- Last Synced: 2025-03-14T23:24:37.034Z (3 months ago)
- Topics: classifier, deep-learning, l-layer-neural-network, neural-network
- Language: Jupyter Notebook
- Homepage:
- Size: 23.4 KB
- Stars: 0
- Watchers: 1
- Forks: 0
- Open Issues: 0
-
Metadata Files:
- Readme: README.md
Awesome Lists containing this project
README
# Cat-vs-Non-cat-nn
Designed a deep neural network to classify images as either containing a cat or not containing a cat.
The network consists of an input layer, l hidden layers, and an output layer. The input layer of the neural network consists of a flattened vector representation of the input image. Each pixel in the image is treated as a feature, and all the features are combined into a single long vector.
The l hidden layers of the network each contain a certain number of neurons.Each neuron in the hidden layer applies an activation function to the weighted sum of its inputs. I have chosen to use the Rectified Linear Unit (ReLU) activation function for all the hidden layers.
The output layer of the network consists of a single neuron with a sigmoid activation function. The sigmoid function takes the weighted sum of the inputs from the previous layer and produces a value between 0 and 1, which represents the probability that the input image contains a cat. If the output value is greater than 0.5, the neural network classifies the input image as a cat. Otherwise, it classifies the image as non-cat.
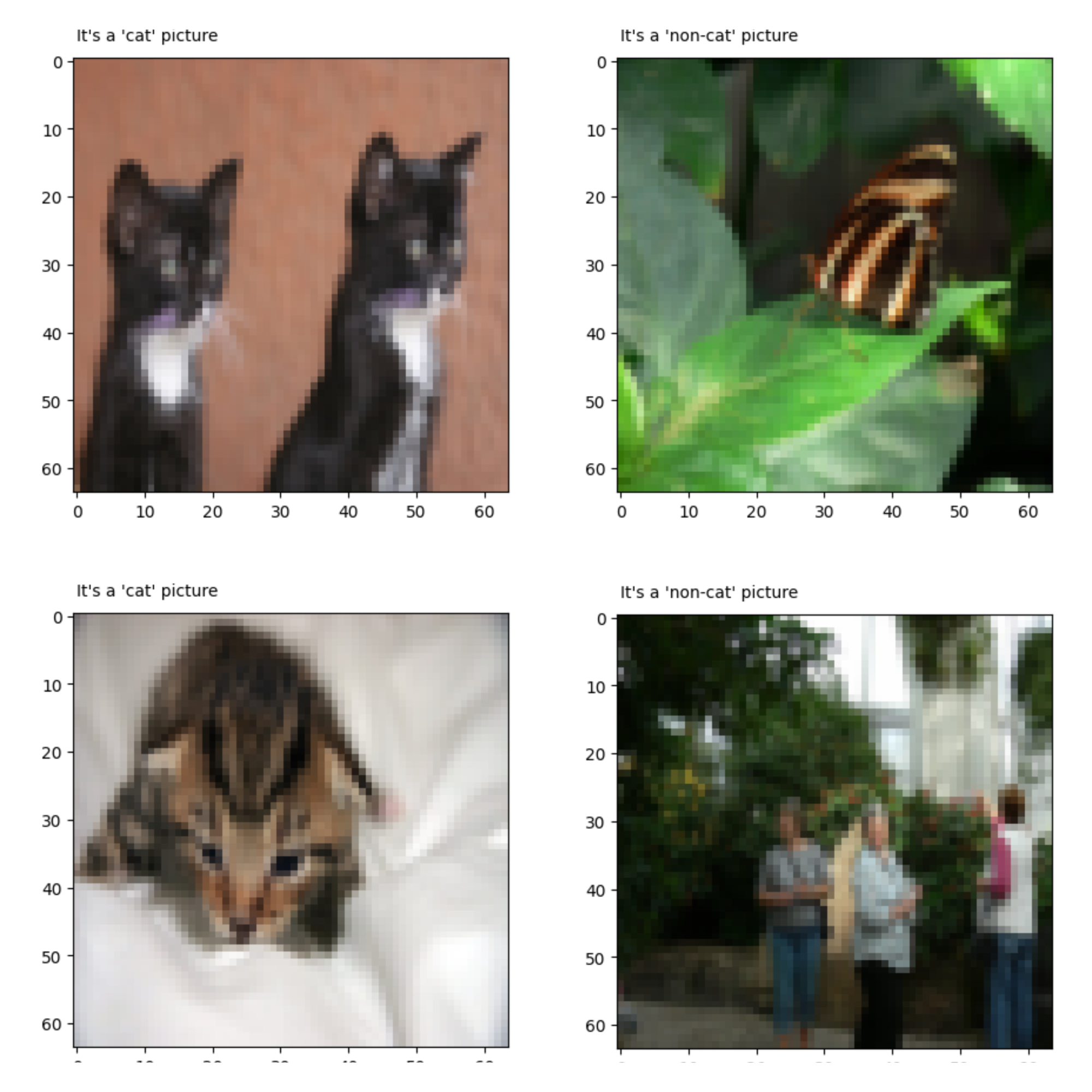