https://github.com/tyler-hilbert/cuda-linearregression
Linear Regression in CUDA
https://github.com/tyler-hilbert/cuda-linearregression
ai cublas cuda gpu linear-regression nsight
Last synced: 3 months ago
JSON representation
Linear Regression in CUDA
- Host: GitHub
- URL: https://github.com/tyler-hilbert/cuda-linearregression
- Owner: Tyler-Hilbert
- Created: 2024-08-02T01:14:41.000Z (11 months ago)
- Default Branch: main
- Last Pushed: 2025-01-03T03:36:23.000Z (6 months ago)
- Last Synced: 2025-03-30T03:31:48.244Z (3 months ago)
- Topics: ai, cublas, cuda, gpu, linear-regression, nsight
- Language: Cuda
- Homepage:
- Size: 390 KB
- Stars: 4
- Watchers: 1
- Forks: 0
- Open Issues: 0
-
Metadata Files:
- Readme: README.md
Awesome Lists containing this project
README
# Linear regression implemented from scratch in CUDA vs cuBLAS
`LinearRegression_cuBLAS.cu` is linear regression written from scratch but utilizing the cuBLAS library.
`LinearRegression_CUDA.cu` is linear regression with kernels written completely from scratch.
`LinearRegression_CUDA_NoSharedMemory.cu` is linear regression with kernels written completely from scratch but without the shared memory optimizations.# cuBLAS vs CUDA Performance
Tested on T4 with 800,000 training points and 200,000 testing points using CUDA 12.2 using Nsight.
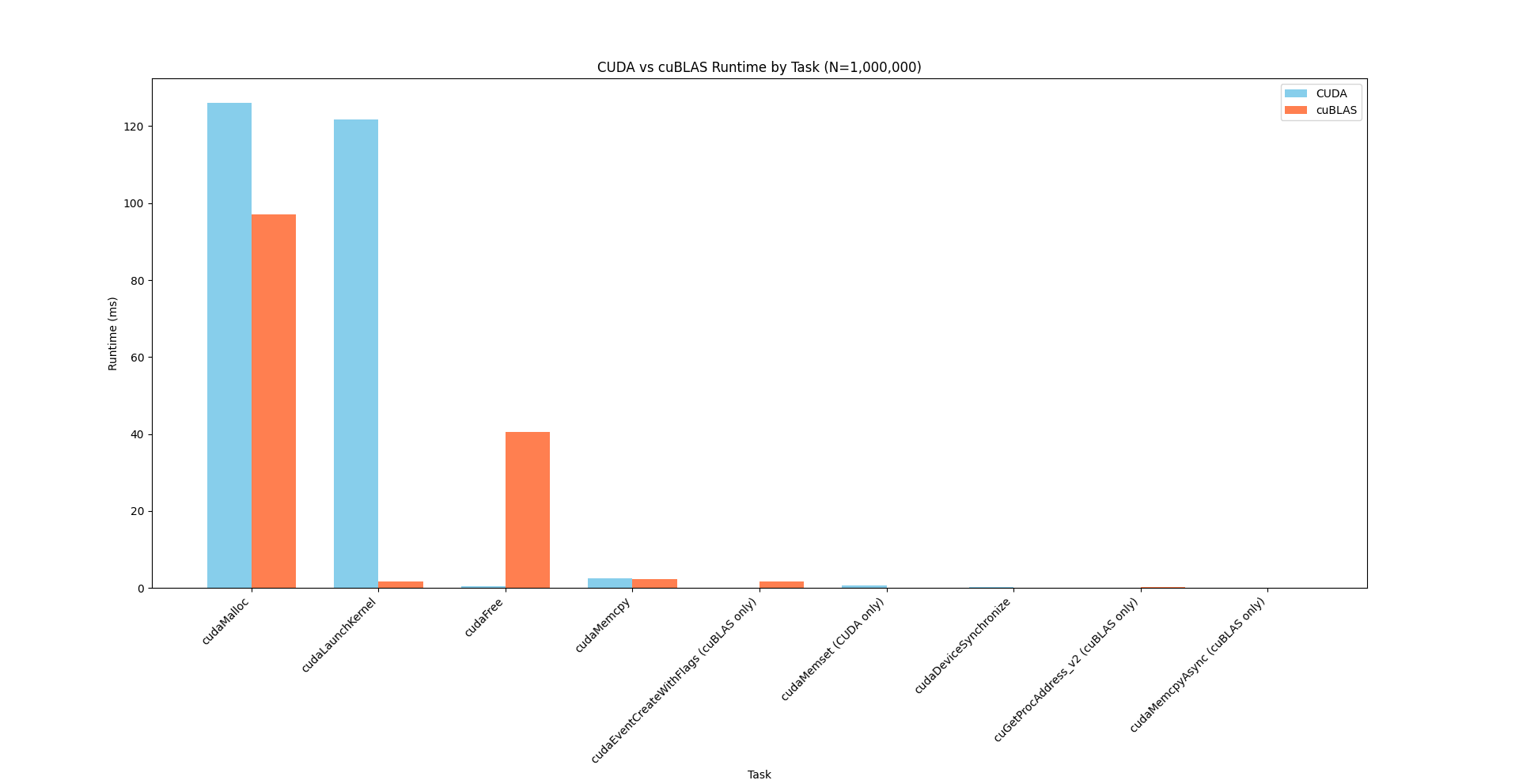# cuBLAS
## Usage
```C++
LinearRegression_cuBLAS model(N, TRAIN_SIZE, TEST_SIZE, x, y);
model.calculate_coefficients();
model.make_predictions();
model.calculate_mse();
```#### Table 1: cuBLAS 'cuda_api_sum'
| Task | Time (%) | Total Time (ns) | Num Calls | Avg (ns) | Med (ns) | Min (ns) | Max (ns) | StdDev (ns) |
|----------------------------|----------|-----------------|-----------|---------------|-------------|----------|--------------|---------------|
| cudaMalloc | 67.5 | 97,130,331 | 6 | 16,188,388.5 | 114,106.0 | 9,119 | 96,736,963 | 39,460,617.3 |
| cudaFree | 28.2 | 40,578,612 | 8 | 5,072,326.5 | 169,325.0 | 3,214 | 36,343,841 | 12,693,387.7 |
| cudaMemcpy | 1.6 | 2,257,018 | 4 | 564,254.5 | 565,201.0 | 170,728 | 955,888 | 434,917.8 |
| cudaLaunchKernel | 1.2 | 1,702,178 | 12 | 141,848.2 | 8,823.5 | 4,870 | 1,509,709 | 431,077.1 |
| cudaEventCreateWithFlags | 1.2 | 1,678,509 | 18 | 93,250.5 | 378.5 | 330 | 1,665,258 | 392,322.7 |
| cuGetProcAddress_v2 | 0.2 | 280,738 | 766 | 366.5 | 240.0 | 90 | 49,982 | 1,909.2 |
| cudaMemcpyAsync | 0.1 | 141,881 | 5 | 28,376.2 | 30,934.0 | 21,578 | 33,780 | 5,627.5 |
| cudaEventRecord | 0.0 | 19,337 | 5 | 3,867.4 | 2,467.0 | 1,029 | 10,421 | 3,822.9 |
| cudaStreamGetCaptureInfo_v2_v11030 | 0.0 | 17,083 | 25 | 683.3 | 436.0 | 234 | 3,133 | 681.9 |
| cudaDeviceSynchronize | 0.0 | 15,637 | 4 | 3,909.3 | 2,011.0 | 988 | 10,627 | 4,524.7 |
| cudaStreamSynchronize | 0.0 | 13,527 | 5 | 2,705.4 | 2,376.0 | 1,756 | 4,171 | 911.6 |
| cudaEventDestroy | 0.0 | 8,490 | 18 | 471.7 | 348.0 | 285 | 1,587 | 311.9 |
| cuInit | 0.0 | 6,545 | 2 | 3,272.5 | 3,272.5 | 2,481 | 4,064 | 1,119.4 |
| cudaEventQuery | 0.0 | 4,780 | 1 | 4,780.0 | 4,780.0 | 4,780 | 4,780 | 0.0 |
| cuModuleGetLoadingMode | 0.0 | 1,477 | 3 | 492.3 | 334.0 | 168 | 975 | 426.2 |#### Table 2: cuBLAS 'cuda_gpu_kern_sum'
| Kernel | Time (%) | Total Time (ns) | Instances | Avg (ns) | Med (ns) | Min (ns) | Max (ns) | StdDev (ns) |
|----------------------|----------|-----------------|-----------|------------|------------|----------|----------|-------------|
| dot_kernel | 37.7 | 53,727 | 3 | 17,909.0 | 18,239.0 | 9,216 | 26,272 | 8,532.8 |
| asum_kernel | 35.5 | 50,622 | 4 | 12,655.5 | 12,367.5 | 4,448 | 21,439 | 9,260.7 |
| axpy_kernel_val | 15.5 | 22,080 | 2 | 11,040.0 | 11,040.0 | 10,912 | 11,168 | 181.0 |
| reduce_1Block_kernel | 11.3 | 16,096 | 3 | 5,365.3 | 5,280.0 | 5,056 | 5,760 | 359.7 |#### Table 3: cuBLAS 'cuda_gpu_mem_time_sum'
| Operation | Time (%) | Total Time (ns) | Count | Avg (ns) | Med (ns) | Min (ns) | Max (ns) | StdDev (ns) |
|-----------------------------|----------|-----------------|-------|------------|------------|----------|----------|-------------|
| [CUDA memcpy Host-to-Device] | 95.6 | 1,533,312 | 3 | 511,104.0 | 701,362.0 | 68,031 | 763,919 | 384,985.2 |
| [CUDA memcpy Device-to-Host] | 4.4 | 70,143 | 6 | 11,690.5 | 2,080.0 | 1,600 | 60,639 | 23,980.9 |### calculate_coefficients
uses 2 x cublasSasum + 2 x cublasSdot
### make_predictions
uses cublasSaxpy
### calculate_mse
uses cublasSaxpy + cublasSdot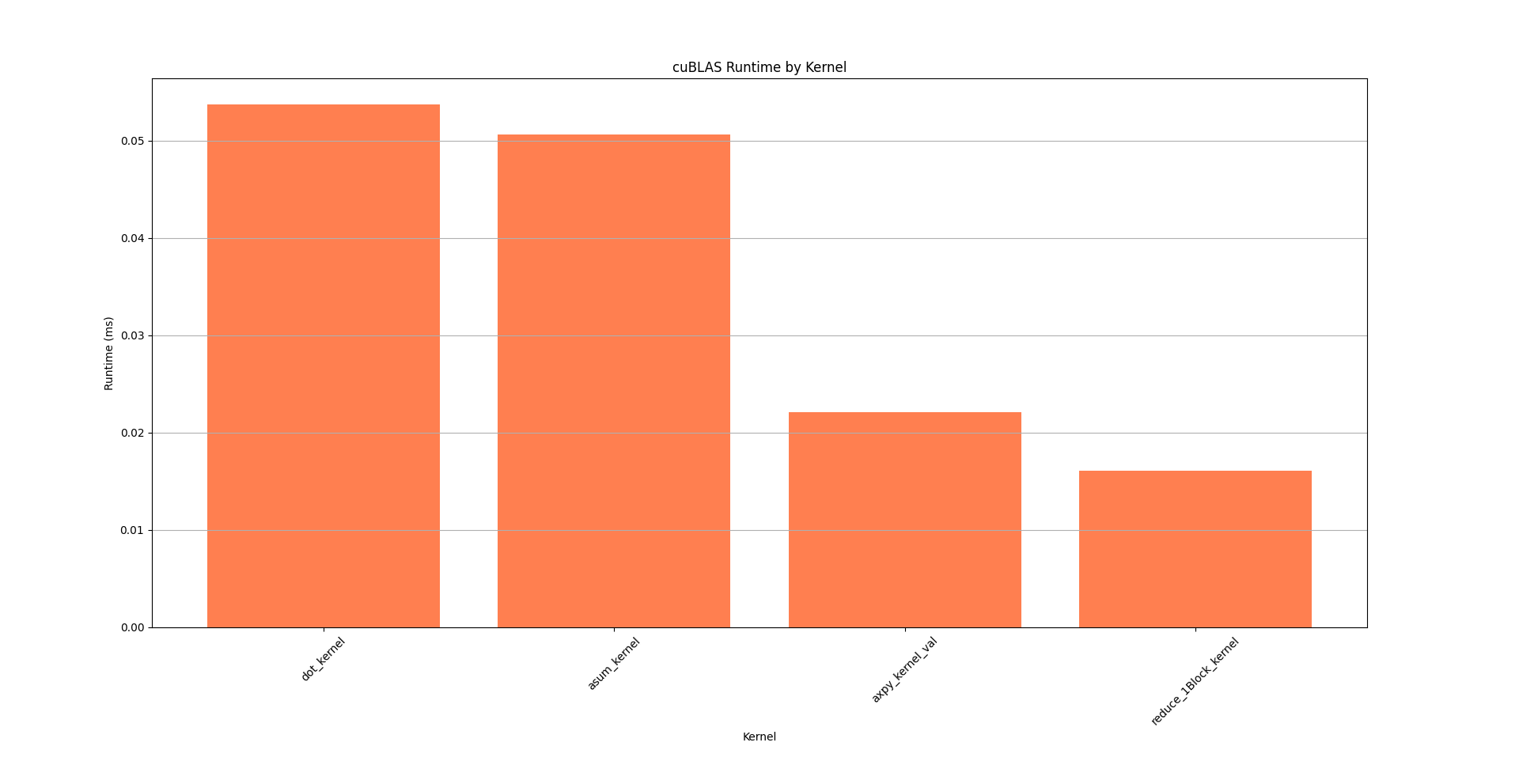
### Example Output
(n=1024)
Slope: 24.999807
Intercept: 49.886719
Predictions
2741: 68574.359375
2715: 67924.367188
913: 22874.710938
...
Mean Squared Error: 27.672958# CUDA (Kernel Written from Scratch)
## Usage
```C++
LinearRegression_CUDA model(N, TRAIN_SIZE, TEST_SIZE, x, y);
model.calculate_coefficients();
model.make_predictions();
model.calculate_mse();
```#### Table 4: CUDA 'cuda_api_sum'
| Task | Time (%) | Total Time (ns) | Num Calls | Avg (ns) | Med (ns) | Min (ns) | Max (ns) | StdDev (ns) |
|------------------------|----------|-----------------|-----------|---------------|-------------|----------|--------------|---------------|
| cudaMalloc | 50.1 | 126,094,891 | 8 | 15,761,861.4 | 13,477.5 | 2,781 | 125,828,040 | 44,473,478.8 |
| cudaLaunchKernel | 48.3 | 121,654,725 | 4 | 30,413,681.3 | 34,383.0 | 19,211 | 121,566,748 | 60,768,712.3 |
| cudaMemcpy | 1.0 | 2,608,023 | 8 | 326,002.9 | 22,941.0 | 10,456 | 916,812 | 430,826.4 |
| cudaMemset | 0.3 | 640,070 | 4 | 160,017.5 | 4,877.0 | 2,505 | 627,811 | 311,870.1 |
| cudaFree | 0.2 | 414,971 | 5 | 82,994.2 | 7,205.0 | 4,713 | 213,711 | 106,401.8 |
| cudaDeviceSynchronize | 0.1 | 286,250 | 4 | 71,562.5 | 85,105.5 | 23,420 | 92,619 | 32,290.6 |
| cuModuleGetLoadingMode | 0.0 | 988 | 1 | 988.0 | 988.0 | 988 | 988 | 0.0 |#### Table 5: CUDA 'cuda_gpu_kern_sum'
| Kernel | Time (%) | Total Time (ns) | Instances | Avg (ns) | Med (ns) | Min (ns) | Max (ns) | StdDev (ns) |
|--------------------------------|----------|-----------------|-----------|------------|------------|----------|----------|-------------|
| calculatePartialCoefficients | 31.8 | 88,766 | 1 | 88,766.0 | 88,766.0 | 88,766 | 88,766 | 0.0 |
| calculatePartialSums | 31.2 | 87,326 | 1 | 87,326.0 | 87,326.0 | 87,326 | 87,326 | 0.0 |
| calculatePartialMSE | 29.8 | 83,262 | 1 | 83,262.0 | 83,262.0 | 83,262 | 83,262 | 0.0 |
| makePredictions | 7.2 | 20,128 | 1 | 20,128.0 | 20,128.0 | 20,128 | 20,128 | 0.0 |#### Table 6: CUDA 'cuda_gpu_mem_time_sum'
| Operation | Time (%) | Total Time (ns) | Count | Avg (ns) | Med (ns) | Min (ns) | Max (ns) | StdDev (ns) |
|-----------------------------|----------|-----------------|-------|------------|------------|----------|----------|-------------|
| [CUDA memcpy Host-to-Device] | 94.9 | 1,382,915 | 2 | 691,457.5 | 691,457.5 | 680,370 | 702,545 | 15,680.1 |
| [CUDA memcpy Device-to-Host] | 4.9 | 71,134 | 6 | 11,855.7 | 1,840.0 | 1,600 | 62,174 | 24,651.7 |
| [CUDA memset] | 0.3 | 3,840 | 4 | 960.0 | 928.0 | 672 | 1,312 | 336.6 |## Kernels (Plain CUDA)
### calculatePartialCoefficients
Calculates the numerator and denominator to be used for slope and bias.
``slope = numerator / denominator; bias = y_mean - slope * x_mean;``### calculatePartialSums
Calculates the sums of X and Y, which are used to calculate the mean of X and Y by dividing by N.### calculatePartialMSE
Calculates the squared error, which is used to calculate the MSE by dividing by N.### makePredictions
Calculates predictions for the array of values x based off slope and bias.
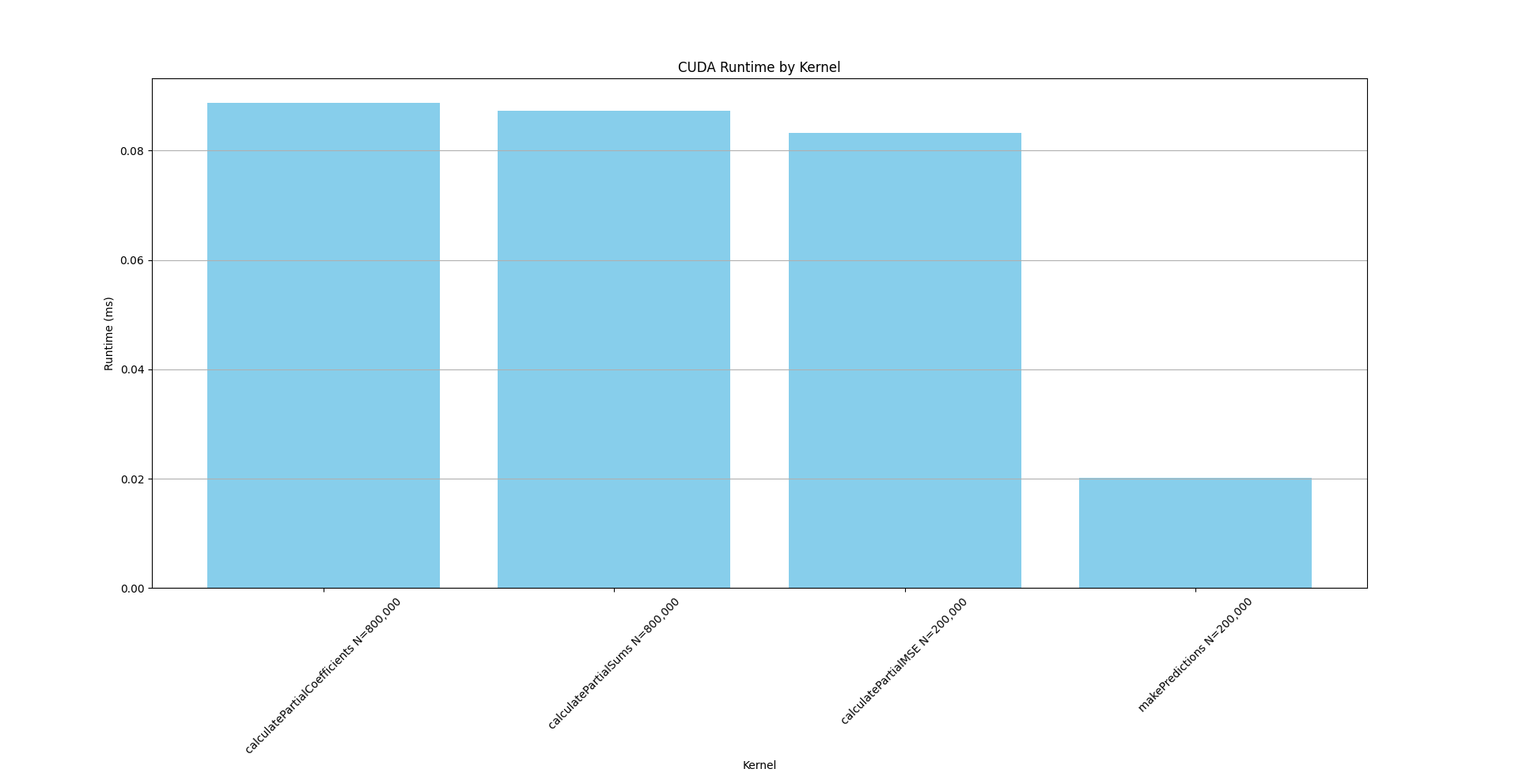### Example Output
(n=1024)
slope 24.999805 intercept 49.890625Predictions
2741 : 68574.359375
2715 : 67924.359375
913 : 22874.712891
...MSE: 27.675436
## (Both CUDA): Shared Memory Kernels vs No Shared Memory Kernels
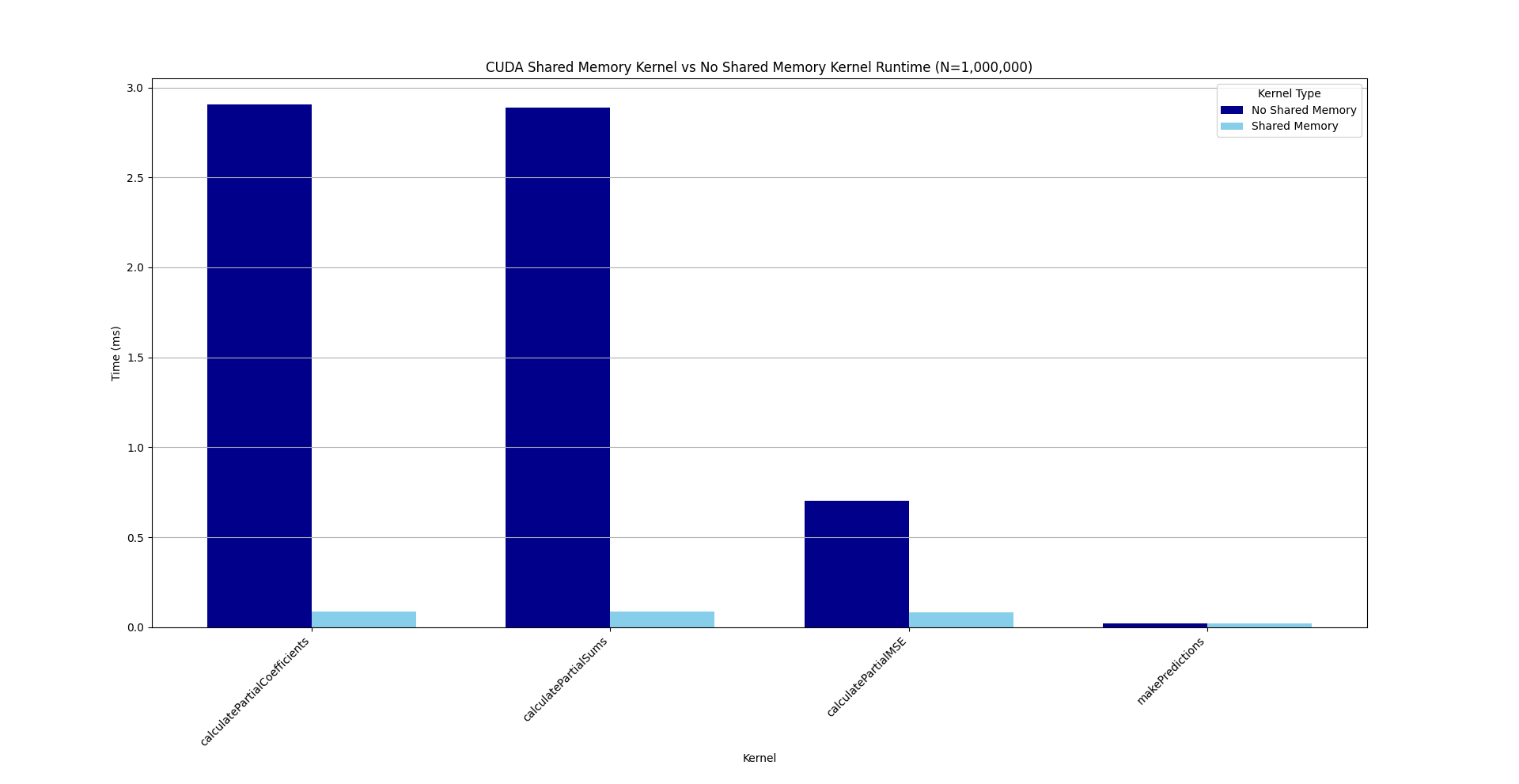
Table 7: CUDA Shared Memory vs No Shared Memory
| Kernel Name | Test | Time (%) | Total Time (ns) |
|----------------------------------------------|-------------------|----------|------------------|
| calculatePartialCoefficients (No Shared) | No Shared Memory | 44.6% | 2,905,087 |
| calculatePartialCoefficients (Shared) | Shared Memory | 31.8% | 88,766 |
| calculatePartialSums (No Shared) | No Shared Memory | 44.3% | 2,887,392 |
| calculatePartialSums (Shared) | Shared Memory | 31.2% | 87,326 |
| calculatePartialMSE (No Shared) | No Shared Memory | 10.8% | 704,785 |
| calculatePartialMSE (Shared) | Shared Memory | 29.8% | 83,262 |
| makePredictions (No Shared) | No Shared Memory | 0.3% | 20,256 |
| makePredictions (Doesn't Use Shared) | Shared Memory | 7.2% | 20,128 |Note: makePredictions doesn't use shared memory in either test.