Ecosyste.ms: Awesome
An open API service indexing awesome lists of open source software.
https://github.com/Mathepia/awesome-sciml
Awesome-spatial-temporal-scientific-machine-learning-data-mining-packages. Julia and Python resources on spatial and temporal data mining. Mathematical epidemiology as an application. Most about package information. Data Sources Links and Epidemic Repos are also included.
https://github.com/Mathepia/awesome-sciml
List: awesome-sciml
bayesian-deep-learning bayesian-inference data-science deep-learning julia machine-learning mathematical-biology mathematical-epidemiology neural-ode physics-informed-neural-networks pinns probabilistic-machine-learning probabilistic-programming scientific-computing scientific-machine-learning time-series universal-differential-equations
Last synced: 23 days ago
JSON representation
Awesome-spatial-temporal-scientific-machine-learning-data-mining-packages. Julia and Python resources on spatial and temporal data mining. Mathematical epidemiology as an application. Most about package information. Data Sources Links and Epidemic Repos are also included.
- Host: GitHub
- URL: https://github.com/Mathepia/awesome-sciml
- Owner: Mathepia
- License: mit
- Created: 2022-11-12T19:24:05.000Z (about 2 years ago)
- Default Branch: main
- Last Pushed: 2022-12-03T00:42:27.000Z (about 2 years ago)
- Last Synced: 2024-05-23T01:06:14.554Z (7 months ago)
- Topics: bayesian-deep-learning, bayesian-inference, data-science, deep-learning, julia, machine-learning, mathematical-biology, mathematical-epidemiology, neural-ode, physics-informed-neural-networks, pinns, probabilistic-machine-learning, probabilistic-programming, scientific-computing, scientific-machine-learning, time-series, universal-differential-equations
- Language: JavaScript
- Homepage: https://mathepia.github.io/awesome-sciml/
- Size: 1.56 MB
- Stars: 7
- Watchers: 2
- Forks: 1
- Open Issues: 0
-
Metadata Files:
- Readme: README.md
- License: LICENSE
Awesome Lists containing this project
- ultimate-awesome - awesome-sciml - Awesome-spatial-temporal-scientific-machine-learning-data-mining-packages. Julia and Python resources on spatial and temporal data mining. Mathematical epidemiology as an application. Most about package information. Data Sources Links and Epidemic Repos are also included. . (Programming Language Lists / Julia Lists)
README
# awesome-sciml
See [Website of awesome-sciml](https://Mathepia.github.io/awesome-sciml/)
Note that at this time packages are all listed in Readme. Now I am gradually classifying them and move them to docs (with the name Resources) in [Website of awesome-sciml](https://Mathepia.github.io/awesome-sciml/). See details in Resources/Tools for AI4Science.
Guides on contributions:
- Open issues to add source links
- Fork and pull requestsAlso see its twin repo [awesome-scimlTutorial: Tutorials on math epidemiology and epidemiology informed deep learning methods](https://github.com/Song921012/MathEpiDeepLearningTutorial).
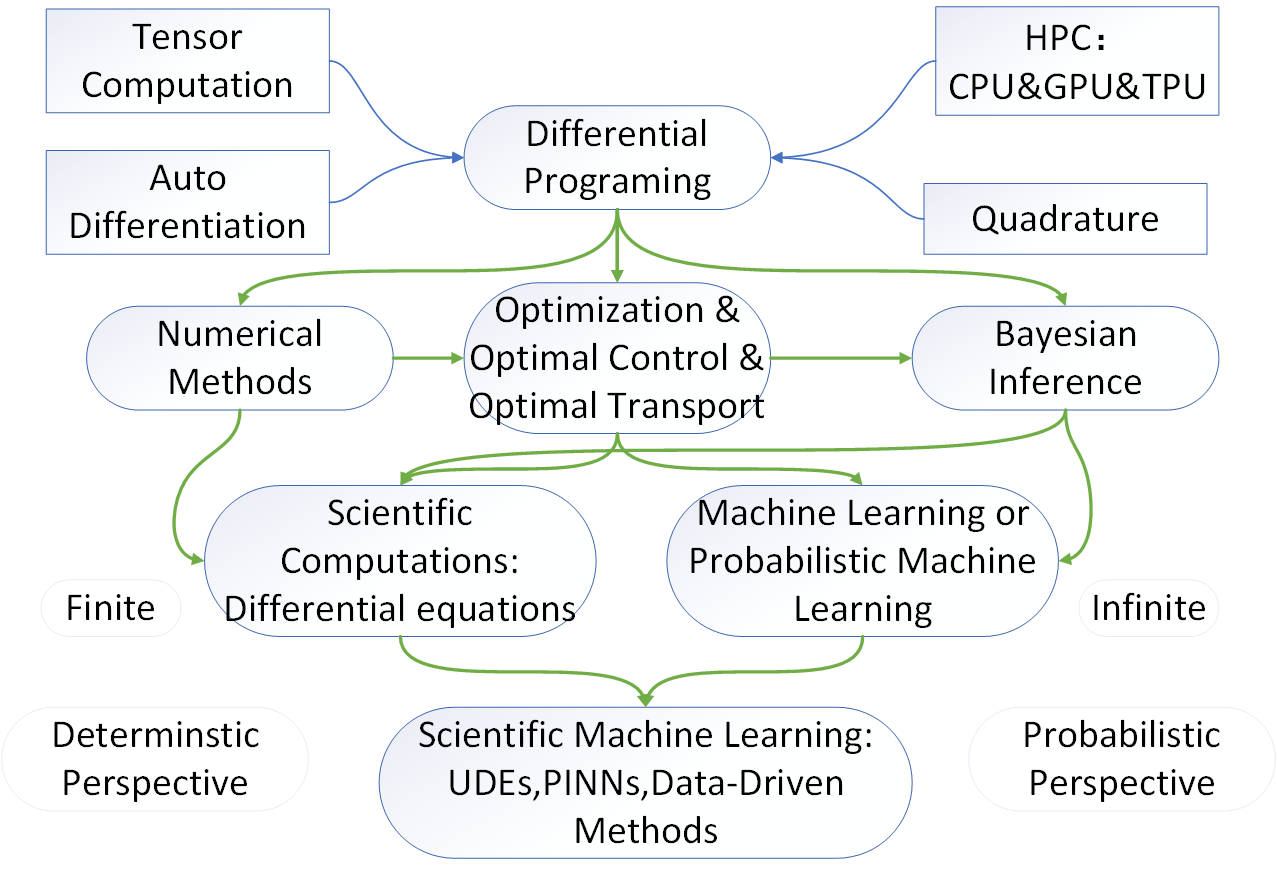
Contents:
- [Introduction](#head1)
- [0. Epidemic Model](#head79)
- [1. Data Preprocessing](#head2)
- [1.1. Data Science](#head3)
- [Smoothing](#head4)
- [Outlier Detection](#head75)
- [2. Basic Statistics and Data Visualization](#head5)
- [2.1. Statistics](#head6)
- [2.2. (Deep Learning based) Time Series Analysis](#head7)
- [2.3. Survival Analysis](#head8)
- [2.4. Data Visualization](#head9)
- [2.5. GLM](#head76)
- [3. Differential Programing and Data Mining](#head10)
- [3.1. Differentiation, Quadrature and Tensor computation](#head11)
- [3.1.1. Auto Differentiation](#head12)
- [Auto Difference](#head13)
- [3.1.2. Quadrature](#head14)
- [Bayesian Methods](#head15)
- [Expectations calculation](#head16)
- [3.1.3. Matrix and Tensor computation](#head17)
- [Special Matrix](#head18)
- [Eigenvalues](#head19)
- [Tensor computation](#head80)
- [Maps and Operators](#head20)
- [Matrix Equations](#head21)
- [Kronecker-based algebra](#head22)
- [3.1.4. CPU, GPU and TPU](#head23)
- [3.2. Optimization](#head24)
- [3.2.1. Metaheuristic](#head25)
- [3.2.2. Evolution Strategy](#head26)
- [3.2.3. Genetic Algorithms](#head27)
- [3.2.4. Nonconvex](#head28)
- [3.3. Optimal Control](#head29)
- [3.4. Bayesian Inference](#head30)
- [3.4.1. MCMC](#head31)
- [3.4.2. Approximate Bayesian Computation (ABC)](#head32)
- [3.4.3. Data Assimilation (SMC, particles filter)](#head33)
- [3.4.4. Variational Inference](#head34)
- [3.4.5. Gaussian, non-Gaussian and Kernel](#head35)
- [3.4.6. Bayesian Optimization](#head36)
- [3.4.7. Information theory](#head37)
- [3.4.8. Uncertainty](#head38)
- [3.4.9. Casual](#head39)
- [3.4.10. Sampling](#head40)
- [3.5. Machine Learning and Deep Learning](#head41)
- [3.5.1. Machine Learning](#head42)
- [3.5.2. Deep Learning](#head43)
- [3.5.3. Reinforce Learning](#head44)
- [3.5.4. GNN](#head45)
- [3.5.5. Transformer](#head46)
- [3.5.6. Transfer Learning](#head47)
- [3.5.7. Neural Tangent](#head48)
- [3.5.8. Visualization](#head49)
- [3.6. Probabilistic Machine Learning and Deep Learning](#head50)
- [3.6.1. GAN](#head51)
- [3.6.2. Normalization Flows](#head52)
- [3.6.3. VAE](#head53)
- [3.7. Differential Equations and Scientific Computation](#head54)
- [3.7.1. Partial differential equation](#head55)
- [3.8. Scientific Machine Learning (Differential Equation and ML)](#head56)
- [3.8.1. Universal Differential Equations. (Neural differential equations)](#head57)
- [3.8.2. Physical Informed Neural Networks](#head58)
- [3.8.3. Neural Operator](#head59)
- [3.9. Data Driven Methods (Equation Searching Methods)](#head60)
- [3.9.1. Symbolic Regression](#head61)
- [3.9.2. SINDy (Sparse Identification of Nonlinear Dynamics from Data)](#head62)
- [3.9.3. DMD (Dynamic Mode Decomposition)](#head63)
- [3.10. Model Evaluation](#head64)
- [3.10.1. Structure Identification](#head65)
- [3.10.2. Global Sensitivity Analysis](#head66)
- [3.10. Optimal Transportation](#head77)
- [3.11. Agents, Graph and Networks](#head81)
- [4. Theoretical Analysis](#head67)
- [4.0. Special Functions](#head68)
- [4.1. Symbolic Computation](#head69)
- [4.3. Roots, Interpolations](#head70)
- [4.3.1. Roots](#head71)
- [4.3.2. Interpolations](#head72)
- [4.2. Bifurcation](#head73)
- [5. Writings, Blog and Web](#head74)
# Introduction
Julia and Python resources on mathematical epidemiology and epidemiology informed deep learning methods. Most about package information. Main Topics include
- Data Preprocessing
- Basic Statistics and Data Visualization
- Differential Programing and Data Mining
such as bayesian inference, deep learning, scientific machine learning computation
- Theoretical Analysis
such as calculus, bifurcation analysis
- Writings, Blog and Web
[TOC]Julia:
[epirecipes/sir-julia: Various implementations of the classical SIR model in Julia](https://github.com/epirecipes/sir-julia)
[jangevaare/Pathogen.jl: Simulation, visualization, and inference tools for modelling the spread of infectious diseases with Julia](https://github.com/jangevaare/Pathogen.jl)
Mobility[jtmatamalas/MMCAcovid19.jl: Microscopic Markov Chain Approach to model the spreading of COVID-19](https://github.com/jtmatamalas/MMCAcovid19.jl)
[jpfairbanks/SemanticModels.jl: A julia package for representing and manipulating model semantics](https://github.com/jpfairbanks/SemanticModels.jl)
[cambridge-mlg/Covid19](https://github.com/cambridge-mlg/Covid19)
[affans/covid19abm.jl: Agent Based Model for COVID 19 transmission dynamics](https://github.com/affans/covid19abm.jl)
Python:
[ryansmcgee/seirsplus: Models of SEIRS epidemic dynamics with extensions, including network-structured populations, testing, contact tracing, and social distancing.](https://github.com/ryansmcgee/seirsplus)
[pyro.contrib.epidemiology.models — Pyro documentation](https://docs.pyro.ai/en/stable/_modules/pyro/contrib/epidemiology/models.html#HeterogeneousSIRModel)
Modelling Human Mobility
[scikit-mobility/scikit-mobility: scikit-mobility: mobility analysis in Python](https://github.com/scikit-mobility/scikit-mobility)Matlab:
[JDureau/AllScripts](https://github.com/JDureau/AllScripts)
# 1. Data Preprocessing
## 1.1. Data Science
Julia:
[JuliaData](https://github.com/JuliaData)
[JuliaData/CSV.jl: Utility library for working with CSV and other delimited files in the Julia programming language](https://github.com/JuliaData/CSV.jl)
[JuliaData/DataFrames.jl: In-memory tabular data in Julia](https://github.com/JuliaData/DataFrames.jl)
[JuliaStats/TimeSeries.jl: Time series toolkit for Julia](https://github.com/JuliaStats/TimeSeries.jl)
[Queryverse](https://github.com/queryverse)
[JuliaDatabases](https://github.com/JuliaDatabases)
Python:
Numpy
Pandas
## Smoothing
[PumasAI/DataInterpolations.jl: A library of data interpolation and smoothing functions](https://github.com/PumasAI/DataInterpolations.jl)
[viraltux/Smoothers.jl: Collection of basic smoothers and smoothing related applications](https://github.com/viraltux/Smoothers.jl)
Expotential Smoothing:
[LAMPSPUC/StateSpaceModels.jl: StateSpaceModels.jl is a Julia package for time-series analysis using state-space models.](https://github.com/LAMPSPUC/StateSpaceModels.jl)
[miguelraz/StagedFilters.jl](https://github.com/miguelraz/StagedFilters.jl)
[JuliaDSP/DSP.jl: Filter design, periodograms, window functions, and other digital signal processing functionality](https://github.com/JuliaDSP/DSP.jl)
[konkam/FeynmanKacParticleFilters.jl: Particle filtering using the Feynman-Kac formalism](https://github.com/konkam/FeynmanKacParticleFilters.jl)
[mschauer/Kalman.jl: Flexible filtering and smoothing in Julia](https://github.com/mschauer/Kalman.jl)
[JuliaStats/Loess.jl: Local regression, so smooooth!](https://github.com/JuliaStats/Loess.jl)
[CliMA/EnsembleKalmanProcesses.jl: Implements Optimization and approximate uncertainty quantification algorithms, Ensemble Kalman Inversion, and Ensemble Kalman Processes.](https://github.com/CliMA/EnsembleKalmanProcesses.jl)
## Outlier Detection
Survey[rob-med/awesome-TS-anomaly-detection: List of tools & datasets for anomaly detection on time-series data.](https://github.com/rob-med/awesome-TS-anomaly-detection)
Julia:
[OutlierDetectionJL](https://github.com/OutlierDetectionJL)
[baggepinnen/MatrixProfile.jl: Time-series analysis using the Matrix profile in Julia](https://github.com/baggepinnen/MatrixProfile.jl)
[jbytecode/LinRegOutliers: Direct and robust methods for outlier detection in linear regression](https://github.com/jbytecode/LinRegOutliers)
Python:
[yzhao062/pyod: A Python Toolbox for Scalable Outlier Detection (Anomaly Detection)](https://github.com/yzhao062/pyod)
[cerlymarco/tsmoothie: A python library for time-series smoothing and outlier detection in a vectorized way.](https://github.com/cerlymarco/tsmoothie)
[DHI/tsod: Anomaly Detection for time series data](https://github.com/DHI/tsod)
# 2. Basic Statistics and Data Visualization
## 2.1. Statistics
[Julia Statistics](https://github.com/JuliaStats)
[gragusa (Giuseppe Ragusa)](https://github.com/gragusa)
[cscherrer/MeasureTheory.jl: "Distributions" that might not add to one.](https://github.com/cscherrer/MeasureTheory.jl)
## 2.2. (Deep Learning based) Time Series Analysis
Julia: (few)
[JuliaStats/TimeSeries.jl: Time series toolkit for Julia](file:///F:/Zotero/Zotero/storage/GJAUE3T5/TimeSeries.html)
[JuliaDynamics/ARFIMA.jl: Simulate stochastic timeseries that follow ARFIMA, ARMA, ARIMA, AR, etc. processes](https://github.com/JuliaDynamics/ARFIMA.jl)
Python:
Survey[MaxBenChrist/awesome_time_series_in_python: This curated list contains python packages for time series analysis](https://github.com/MaxBenChrist/awesome_time_series_in_python)
[Introduction — statsmodels](file:///F:/Zotero/Zotero/storage/JRHBIF8V/index.html)
[unit8co/darts: A python library for easy manipulation and forecasting of time series.](https://github.com/unit8co/darts)
[jdb78/pytorch-forecasting: Time series forecasting with PyTorch](https://github.com/jdb78/pytorch-forecasting)
[AIStream-Peelout/flow-forecast: Deep learning PyTorch library for time series forecasting, classification, and anomaly detection (originally for flood forecasting).](https://github.com/AIStream-Peelout/flow-forecast)
[timeseriesAI/tsai: Time series Timeseries Deep Learning Machine Learning Pytorch fastai | State-of-the-art Deep Learning library for Time Series and Sequences in Pytorch / fastai](https://github.com/timeseriesAI/tsai)
[tslearn-team/tslearn: A machine learning toolkit dedicated to time-series data](https://github.com/tslearn-team/tslearn)
[salesforce/Merlion: Merlion: A Machine Learning Framework for Time Series Intelligence](https://github.com/salesforce/Merlion)
[ourownstory/neural_prophet: NeuralProphet: A simple forecasting package](https://github.com/ourownstory/neural_prophet)
[alan-turing-institute/sktime: A unified framework for machine learning with time series](https://github.com/alan-turing-institute/sktime)
[sktime/sktime-dl: sktime companion package for deep learning based on TensorFlow](https://github.com/sktime/sktime-dl)
[IBM/TSML.jl: A package for time series data processing, classification, clustering, and prediction.](https://github.com/IBM/TSML.jl)
[alkaline-ml/pmdarima: A statistical library designed to fill the void in Python's time series analysis capabilities, including the equivalent of R's auto.arima function.](https://github.com/alkaline-ml/pmdarima)
[zhouhaoyi/Informer2020: The GitHub repository for the paper "Informer" accepted by AAAI 2021.](https://github.com/zhouhaoyi/Informer2020)
[blue-yonder/tsfresh: Automatic extraction of relevant features from time series:](https://github.com/blue-yonder/tsfresh)
[microsoft/forecasting: Time Series Forecasting Best Practices & Examples](https://github.com/microsoft/forecasting)
[TDAmeritrade/stumpy: STUMPY is a powerful and scalable Python library for modern time series analysis](https://github.com/TDAmeritrade/stumpy)
[databrickslabs/tempo: API for manipulating time series on top of Apache Spark: lagged time values, rolling statistics (mean, avg, sum, count, etc), AS OF joins, downsampling, and interpolation](https://github.com/databrickslabs/tempo)
## 2.3. Survival Analysis
Julia:
Python:
[Deep Learning for Survival Analysis](https://humboldt-wi.github.io/blog/research/information_systems_1920/group2_survivalanalysis/)
[sebp/scikit-survival: Survival analysis built on top of scikit-learn](https://github.com/sebp/scikit-survival)
[havakv/pycox: Survival analysis with PyTorch](https://github.com/havakv/pycox)
[CamDavidsonPilon/lifelines: Survival analysis in Python](https://github.com/camDavidsonPilon/lifelines)
[chl8856/DeepHit: DeepHit: A Deep Learning Approach to Survival Analysis with Competing Risks](https://github.com/chl8856/DeepHit)
[jaredleekatzman/DeepSurv: DeepSurv is a deep learning approach to survival analysis.](https://github.com/jaredleekatzman/DeepSurv)
[square/pysurvival: Open source package for Survival Analysis modeling](https://github.com/square/pysurvival/)
## 2.4. Data Visualization
Julia:
[JuliaPlots](https://github.com/JuliaPlots)
[GiovineItalia/Gadfly.jl: Crafty statistical graphics for Julia.](https://github.com/GiovineItalia/Gadfly.jl)
[queryverse/VegaLite.jl: Julia bindings to Vega-Lite](https://github.com/queryverse/VegaLite.jl)
[JuliaPlots/UnicodePlots.jl: Unicode-based scientific plotting for working in the terminal](https://github.com/JuliaPlots/UnicodePlots.jl)
Colors and Color schemes
[JuliaGraphics/Colors.jl: Color manipulation utilities for Julia](https://github.com/JuliaGraphics/Colors.jl)
[JuliaGraphics/ColorSchemes.jl: colorschemes, colormaps, gradients, and palettes](https://github.com/JuliaGraphics/ColorSchemes.jl)
Interactive
[GenieFramework/Stipple.jl: The reactive UI library for interactive data applications with pure Julia.](https://github.com/GenieFramework/Stipple.jl)
[theogf/Turkie.jl: Turing + Makie = Turkie](https://github.com/theogf/Turkie.jl)
Python:
Matplotlib
[rougier/scientific-visualization-book: An open access book on scientific visualization using python and matplotlib](https://github.com/rougier/scientific-visualization-book)
R:
Color themes:
[discrete.knit](https://emilhvitfeldt.github.io/r-color-palettes/discrete.html)
### Venn Diagrams
R:
[yanlinlin82/ggvenn: Venn Diagram by ggplot2, with really easy-to-use API.](https://github.com/yanlinlin82/ggvenn)
[gaospecial/ggVennDiagram: A 'ggplot2' implement of Venn Diagram.](https://github.com/gaospecial/ggVennDiagram)
Python:
[konstantint/matplotlib-venn: Area-weighted venn-diagrams for Python/matplotlib](https://github.com/konstantint/matplotlib-venn)
Julia:
[JuliaPlots/VennEuler.jl: Venn/Euler Diagrams for Julia](https://github.com/JuliaPlots/VennEuler.jl)
## 2.5. GLM
[bambinos/bambi: BAyesian Model-Building Interface (Bambi) in Python.](https://github.com/bambinos/bambi)
# 3. Differential Programing and Data Mining
[The Algorithms](https://github.com/TheAlgorithms)
## 3.1. Differentiation, Quadrature and Tensor computation
### 3.1.1. Auto Differentiation
[SciML/DiffEqSensitivity.jl: A component of the DiffEq ecosystem for enabling sensitivity analysis for scientific machine learning (SciML). Optimize-then-discretize, discretize-then-optimize, and more for ODEs, SDEs, DDEs, DAEs, etc.](https://github.com/SciML/DiffEqSensitivity.jl)
Julia:
[FluxML/Zygote.jl: Intimate Affection Auditor](https://github.com/FluxML/Zygote.jl)
JuliaDiffEqFlux organization
[JuliaDiff](https://github.com/JuliaDiff)
[JuliaDiff/ForwardDiff.jl: Forward Mode Automatic Differentiation for Julia](https://github.com/JuliaDiff/ForwardDiff.jl)
[JuliaDiff/ReverseDiff.jl: Reverse Mode Automatic Differentiation for Julia](https://github.com/JuliaDiff/ReverseDiff.jl)
[JuliaDiff/AbstractDifferentiation.jl: An abstract interface for automatic differentiation.](https://github.com/JuliaDiff/AbstractDifferentiation.jl)
[JuliaDiff/TaylorSeries.jl: A julia package for Taylor polynomial expansions in one and several independent variables.](https://github.com/JuliaDiff/TaylorSeries.jl)
[kailaix/ADCME.jl: Automatic Differentiation Library for Computational and Mathematical Engineering](https://github.com/kailaix/ADCME.jl)
[chakravala/Leibniz.jl: Tensor algebra utility library](https://github.com/chakravala/Leibniz.jl)
[briochemc/F1Method.jl: F-1 method](https://github.com/briochemc/F1Method.jl)
Python:
[google/jax: Composable transformations of Python+NumPy programs: differentiate, vectorize, JIT to GPU/TPU, and more](https://github.com/google/jax)
[pytorch/pytorch: Tensors and Dynamic neural networks in Python with strong GPU acceleration](https://github.com/pytorch/pytorch)
[tensorflow/tensorflow: An Open Source Machine Learning Framework for Everyone](https://github.com/tensorflow/tensorflow)
[AMICI-dev/AMICI: Advanced Multilanguage Interface to CVODES and IDAS](https://github.com/AMICI-dev/AMICI)
#### Auto Difference
Julia:
[SciML/DiffEqOperators.jl: Linear operators for discretizations of differential equations and scientific machine learning (SciML)](https://github.com/SciML/DiffEqOperators.jl)
[QuantEcon/SimpleDifferentialOperators.jl: Library for simple upwind finite differences](https://github.com/QuantEcon/SimpleDifferentialOperators.jl)
Python:
[maroba/findiff: Python package for numerical derivatives and partial differential equations in any number of dimensions.](https://github.com/maroba/findiff)
### 3.1.2. Quadrature
Learn One equals learn many
[SciML/Quadrature.jl: A common interface for quadrature and numerical integration for the SciML scientific machine learning organization](https://github.com/SciML/Quadrature.jl)
[SciML/QuasiMonteCarlo.jl: Lightweight and easy generation of quasi-Monte Carlo sequences with a ton of different methods on one API for easy parameter exploration in scientific machine learning (SciML)](https://github.com/SciML/QuasiMonteCarlo.jl)
[SciML/SymbolicNumericIntegration.jl](https://github.com/SciML/SymbolicNumericIntegration.jl)
Julia:
[JuliaMath/QuadGK.jl: adaptive 1d numerical Gauss–Kronrod integration in Julia](https://github.com/JuliaMath/QuadGK.jl)
[JuliaMath/HCubature.jl: pure-Julia multidimensional h-adaptive integration](https://github.com/JuliaMath/HCubature.jl)
[JuliaMath/Cubature.jl: One- and multi-dimensional adaptive integration routines for the Julia language](https://github.com/JuliaMath/Cubature.jl)
[giordano/Cuba.jl: Library for multidimensional numerical integration with four independent algorithms: Vegas, Suave, Divonne, and Cuhre.](https://github.com/giordano/Cuba.jl)
[JuliaApproximation/FastGaussQuadrature.jl: Julia package for Gaussian quadrature](https://github.com/JuliaApproximation/FastGaussQuadrature.jl)
[JuliaApproximation/ApproxFun.jl: Julia package for function approximation](https://github.com/JuliaApproximation/ApproxFun.jl)
[machakann/DoubleExponentialFormulas.jl: One-dimensional numerical integration using the double exponential formula](https://github.com/machakann/DoubleExponentialFormulas.jl)
[JuliaApproximation/SingularIntegralEquations.jl: Julia package for solving singular integral equations](https://github.com/JuliaApproximation/SingularIntegralEquations.jl)
[JuliaGNI/GeometricIntegrators.jl: Geometric Numerical Integration in Julia](https://github.com/JuliaGNI/GeometricIntegrators.jl)
#### Bayesian Methods
Julia:
[ranjanan/MonteCarloIntegration.jl: A package for multi-dimensional integration using monte carlo methods](https://github.com/ranjanan/MonteCarloIntegration.jl)
[theogf/BayesianQuadrature.jl: Is there anything we can't make Bayesian?](https://github.com/theogf/BayesianQuadrature.jl)
[s-baumann/BayesianIntegral.jl: Bayesian Integration of functions](https://github.com/s-baumann/BayesianIntegral.jl)
[theogf/BayesianQuadrature.jl: Is there anything we can't make Bayesian?](https://github.com/theogf/BayesianQuadrature.jl)
#### Expectations calculation
[QuantEcon/Expectations.jl: Expectation operators for Distributions.jl objects](https://github.com/QuantEcon/Expectations.jl)
### 3.1.3. Matrix and Tensor computation
Matrix organization
[JuliaArrays](https://github.com/JuliaArrays)
- [JuliaArrays/StaticArrays.jl: Statically sized arrays for Julia](https://github.com/JuliaArrays/StaticArrays.jl)
- [JuliaArrays/ArrayInterface.jl: Designs for new Base array interface primitives, used widely through scientific machine learning (SciML) and other organizations](https://github.com/JuliaArrays/ArrayInterface.jl)
- [JuliaArrays/StructArrays.jl: Efficient implementation of struct arrays in Julia](https://github.com/JuliaArrays/StructArrays.jl)
- [JuliaArrays/LazyArrays.jl: Lazy arrays and linear algebra in Julia](https://github.com/JuliaArrays/LazyArrays.jl)
- [JuliaArrays/AxisArrays.jl: Performant arrays where each dimension can have a named axis with values](https://github.com/JuliaArrays/AxisArrays.jl)
- [JuliaArrays/OffsetArrays.jl: Fortran-like arrays with arbitrary, zero or negative starting indices.](https://github.com/JuliaArrays/OffsetArrays.jl)
- [JuliaArrays/BlockArrays.jl: BlockArrays for Julia](https://github.com/JuliaArrays/BlockArrays.jl)
- [JuliaArrays/ArraysOfArrays.jl: Efficient storage and handling of nested arrays in Julia](https://github.com/JuliaArrays/ArraysOfArrays.jl)
- [JuliaArrays/InfiniteArrays.jl: A Julia package for representing infinite-dimensional arrays](https://github.com/JuliaArrays/InfiniteArrays.jl)
- [JuliaArrays/FillArrays.jl: Julia package for lazily representing matrices filled with a single entry](https://github.com/JuliaArrays/FillArrays.jl)[JuliaMatrices](https://github.com/JuliaMatrices)
- [JuliaMatrices/BandedMatrices.jl: A Julia package for representing banded matrices](https://github.com/JuliaMatrices/BandedMatrices.jl)
- [JuliaMatrices/BlockBandedMatrices.jl: A Julia package for representing block-banded matrices and banded-block-banded matrices](https://github.com/JuliaMatrices/BlockBandedMatrices.jl)
- [JuliaMatrices/SpecialMatrices.jl: Julia package for working with special matrix types.](https://github.com/JuliaMatrices/SpecialMatrices.jl)
- [JuliaMatrices/InfiniteLinearAlgebra.jl: A Julia repository for linear algebra with infinite matrices](https://github.com/JuliaMatrices/InfiniteLinearAlgebra.jl)[RalphAS](https://github.com/RalphAS)
[JuliaLinearAlgebra](https://github.com/JuliaLinearAlgebra)
[JuliaSparse](https://github.com/JuliaSparse)
[JuliaLang/SparseArrays.jl: SparseArrays.jl is a Julia stdlib](https://github.com/JuliaLang/SparseArrays.jl)
[SciML/LabelledArrays.jl: Arrays which also have a label for each element for easy scientific machine learning (SciML)](https://github.com/SciML/LabelledArrays.jl)
[SciML/RecursiveArrayTools.jl: Tools for easily handling objects like arrays of arrays and deeper nestings in scientific machine learning (SciML) and other applications](https://github.com/SciML/RecursiveArrayTools.jl)
Python:
numpy
numba
[scikit-hep/awkward-1.0: Manipulate JSON-like data with NumPy-like idioms.](https://github.com/scikit-hep/awkward-1.0)
#### Special Matrix and Arrays
[JuliaMatrices/SpecialMatrices.jl: Julia package for working with special matrix types.](https://github.com/JuliaMatrices/SpecialMatrices.jl)
[SciML/LabelledArrays.jl: Arrays which also have a label for each element for easy scientific machine learning (SciML)](https://github.com/SciML/LabelledArrays.jl)
#### Computation
BLAS and LAPACK[JuliaLinearAlgebra/MKL.jl: Intel MKL linear algebra backend for Julia](https://github.com/JuliaLinearAlgebra/MKL.jl)
[mcabbott/Tullio.jl: ⅀](https://github.com/mcabbott/Tullio.jl)
[JuliaLinearAlgebra/Octavian.jl: Multi-threaded BLAS-like library that provides pure Julia matrix multiplication](https://github.com/JuliaLinearAlgebra/Octavian.jl)
[JuliaGPU/GemmKernels.jl: Flexible and performant GEMM kernels in Julia](https://github.com/JuliaGPU/GemmKernels.jl)
[MasonProtter/Gaius.jl: Divide and Conquer Linear Algebra](https://github.com/MasonProtter/Gaius.jl)
#### Eigenvalues and Solvers
Eig[nep-pack/NonlinearEigenproblems.jl: Nonlinear eigenvalue problems in Julia: Iterative methods and benchmarks](https://github.com/nep-pack/NonlinearEigenproblems.jl)
Solver[SciML/LinearSolve.jl: LinearSolve.jl: High-Performance Unified Linear Solvers](https://github.com/SciML/LinearSolve.jl)
Julia:
Eig:
[JuliaLinearAlgebra/Arpack.jl: Julia Wrappers for the arpack-ng Fortran library](https://github.com/JuliaLinearAlgebra/Arpack.jl)[JuliaLinearAlgebra/ArnoldiMethod.jl: Implicitly Restarted Arnoldi Method, natively in Julia](https://github.com/JuliaLinearAlgebra/ArnoldiMethod.jl)
[Jutho/KrylovKit.jl: Krylov methods for linear problems, eigenvalues, singular values and matrix functions](https://github.com/Jutho/KrylovKit.jl)
[pablosanjose/QuadEig.jl: Julia implementation of the `quadeig` algorithm for the solution of quadratic matrix pencils](https://github.com/pablosanjose/QuadEig.jl)
[JuliaApproximation/SpectralMeasures.jl: Julia package for finding the spectral measure of structured self adjoint operators](https://github.com/JuliaApproximation/SpectralMeasures.jl)
Solver:
[JuliaInv/KrylovMethods.jl: Simple and fast Julia implementation of Krylov subspace methods for linear systems.](https://github.com/JuliaInv/KrylovMethods.jl)
[JuliaSmoothOptimizers/Krylov.jl: A Julia Basket of Hand-Picked Krylov Methods](https://github.com/JuliaSmoothOptimizers/Krylov.jl)
Eig Too[JuliaLinearAlgebra/IterativeSolvers.jl: Iterative algorithms for solving linear systems, eigensystems, and singular value problems](https://github.com/JuliaLinearAlgebra/IterativeSolvers.jl)
[tjdiamandis/RandomizedPreconditioners.jl](https://github.com/tjdiamandis/RandomizedPreconditioners.jl)
[JuliaLinearAlgebra/RecursiveFactorization.jl](https://github.com/JuliaLinearAlgebra/RecursiveFactorization.jl)
Spectral methods
[JuliaApproximation/SpectralMeasures.jl: Julia package for finding the spectral measure of structured self adjoint operators](https://github.com/JuliaApproximation/SpectralMeasures.jl)
[tpapp/SpectralKit.jl: Building blocks of spectral methods for Julia.](https://github.com/tpapp/SpectralKit.jl)
Spasrse Slover
Sparse[JuliaSparse/Pardiso.jl: Calling the PARDISO library from Julia](https://github.com/JuliaSparse/Pardiso.jl)
Sparse[JuliaSparse/MKLSparse.jl: Make available to Julia the sparse functionality in MKL](https://github.com/JuliaSparse/MKLSparse.jl)
Sparse[JuliaLang/SuiteSparse.jl: Development of SuiteSparse.jl, which ships as part of the Julia standard library.](https://github.com/JuliaLang/SuiteSparse.jl)
Python:
[scipy.sparse.linalg.eigs — SciPy v1.7.1 Manual](https://docs.scipy.org/doc/scipy/reference/generated/scipy.sparse.linalg.eigs.html?highlight=scipy%20sparse%20linalg%20eigs#scipy.sparse.linalg.eigs)
#### Maps and Operators
[Jutho/LinearMaps.jl: A Julia package for defining and working with linear maps, also known as linear transformations or linear operators acting on vectors. The only requirement for a LinearMap is that it can act on a vector (by multiplication) efficiently.](https://github.com/Jutho/LinearMaps.jl)
[emmt/LazyAlgebra.jl: A Julia package to extend the notion of vectors and matrices](https://github.com/emmt/LazyAlgebra.jl)
[JuliaSmoothOptimizers/LinearOperators.jl: Linear Operators for Julia](https://github.com/JuliaSmoothOptimizers/LinearOperators.jl)
[kul-optec/AbstractOperators.jl: Abstract operators for large scale optimization in Julia](https://github.com/kul-optec/AbstractOperators.jl)
[matthieugomez/InfinitesimalGenerators.jl: A set of tools to work with Markov Processes](https://github.com/matthieugomez/InfinitesimalGenerators.jl)
[ranocha/SummationByPartsOperators.jl: A Julia library of summation-by-parts (SBP) operators used in finite difference, Fourier pseudospectral, continuous Galerkin, and discontinuous Galerkin methods to get provably stable semidiscretizations, paying special attention to boundary conditions.](https://github.com/ranocha/SummationByPartsOperators.jl)
[hakkelt/FunctionOperators.jl: Julia package that allows writing code close to mathematical notation memory-efficiently.](https://github.com/hakkelt/FunctionOperators.jl)
[JuliaApproximation/ApproxFun.jl: Julia package for function approximation](https://github.com/JuliaApproximation/ApproxFun.jl)
#### Matrxi Equations
[andreasvarga/MatrixEquations.jl: Solution of Lyapunov, Sylvester and Riccati matrix equations using Julia](https://github.com/andreasvarga/MatrixEquations.jl)
#### Kronecker-based algebra
[MichielStock/Kronecker.jl: A general-purpose toolbox for efficient Kronecker-based algebra.](https://github.com/MichielStock/Kronecker.jl)
### 3.1.4.Platforms, CPU, GPU and TPU
Julia GPU organization
[JuliaGPU](https://github.com/JuliaGPU)
Python:
[tonybaloney/Pyjion: Pyjion - A JIT for Python based upon CoreCLR](https://github.com/tonybaloney/Pyjion)
[numba/numba: NumPy aware dynamic Python compiler using LLVM](https://github.com/numba/numba)
## 3.2. Optimization
An "learn one equals learn all" Julia Package
[SciML/GalacticOptim.jl: Local, global, and beyond optimization for scientific machine learning (SciML)](https://github.com/SciML/GalacticOptim.jl)
Opt Organization:
[JuliaOpt](https://github.com/JuliaOpt)
[JuliaNLSolvers](https://github.com/JuliaNLSolvers)
[Process Systems and Operations Research Laboratory](https://github.com/PSORLab)
[JuliaNLSolvers/Optim.jl: Optimization functions for Julia](https://github.com/JuliaNLSolvers/Optim.jl)
[JuliaOpt/NLopt.jl: Package to call the NLopt nonlinear-optimization library from the Julia language](https://github.com/JuliaOpt/NLopt.jl)
[robertfeldt/BlackBoxOptim.jl: Black-box optimization for Julia](https://github.com/robertfeldt/BlackBoxOptim.jl)
[jump-dev/MathOptInterface.jl: An abstraction layer for mathematical optimization solvers.](https://github.com/jump-dev/MathOptInterface.jl)
[tpapp/MultistartOptimization.jl: Multistart optimization methods in Julia.](https://github.com/tpapp/MultistartOptimization.jl)
[bbopt/NOMAD.jl: Julia interface to the NOMAD blackbox optimization software](https://github.com/bbopt/NOMAD.jl)
[JuliaFirstOrder](https://github.com/JuliaFirstOrder)
[NicolasL-S/SpeedMapping.jl: General fixed point mapping acceleration and optimization in Julia](https://github.com/NicolasL-S/SpeedMapping.jl)
[JuliaManifolds/Manopt.jl: Optimization on Manifolds in Julia](https://github.com/JuliaManifolds/Manopt.jl)
### 3.2.1. Metaheuristic
Julia:
[jmejia8/Metaheuristics.jl: High performance metaheuristics for optimization purely coded in Julia.](https://github.com/jmejia8/Metaheuristics.jl)
[ac-tuwien/MHLib.jl: MHLib.jl - A Toolbox for Metaheuristics and Hybrid Optimization Methods in Julia](https://github.com/ac-tuwien/MHLib.jl)
Python:
[guofei9987/scikit-opt: Genetic Algorithm, Particle Swarm Optimization, Simulated Annealing, Ant Colony Optimization Algorithm,Immune Algorithm, Artificial Fish Swarm Algorithm, Differential Evolution and TSP(Traveling salesman)](https://github.com/guofei9987/scikit-opt)
[scikit-optimize/scikit-optimize: Sequential model-based optimization with a `scipy.optimize` interface](https://github.com/scikit-optimize/scikit-optimize)
[ac-tuwien/pymhlib: pymhlib - A Toolbox for Metaheuristics and Hybrid Optimization Methods](https://github.com/ac-tuwien/pymhlib)
[cvxpy/cvxpy: A Python-embedded modeling language for convex optimization problems.](https://github.com/cvxpy/cvxpy)
[coin-or/pulp: A python Linear Programming API](https://github.com/coin-or/pulp)
### 3.2.2. Evolution Stragegy
Julia:
[wildart/Evolutionary.jl: Evolutionary & genetic algorithms for Julia](https://github.com/wildart/Evolutionary.jl)
[d9w/Cambrian.jl: An Evolutionary Computation framework](https://github.com/d9w/Cambrian.jl)
[jbrea/CMAEvolutionStrategy.jl](https://github.com/jbrea/CMAEvolutionStrategy.jl)
[AStupidBear/GCMAES.jl: Gradient-based Covariance Matrix Adaptation Evolutionary Strategy for Real Blackbox Optimization](https://github.com/AStupidBear/GCMAES.jl)
[itsdfish/DifferentialEvolutionMCMC.jl: A Julia package for Differential Evolution MCMC](https://github.com/itsdfish/DifferentialEvolutionMCMC.jl)
### 3.2.3. Genetic Algorithms
Julia:
[d9w/CartesianGeneticProgramming.jl: Cartesian Genetic Programming for Julia](https://github.com/d9w/CartesianGeneticProgramming.jl)
[WestleyArgentum/GeneticAlgorithms.jl: A lightweight framework for writing genetic algorithms in Julia](https://github.com/WestleyArgentum/GeneticAlgorithms.jl)
Python:
[trevorstephens/gplearn: Genetic Programming in Python, with a scikit-learn inspired API](https://github.com/trevorstephens/gplearn)
### 3.2.4. Nonconvex
Julia:
[JuliaNonconvex/Nonconvex.jl: Toolbox for non-convex constrained optimization.](https://github.com/JuliaNonconvex/Nonconvex.jl)
### 3.2.5. First Order Methods
Proximal
[OPTEC](https://github.com/kul-optec)[kul-optec/CIAOAlgorithms.jl: Coordinate and Incremental Aggregated Optimization Algorithms](https://github.com/kul-optec/CIAOAlgorithms.jl)
## 3.3. Optimal Control
[eleurent/phd-bibliography: References on Optimal Control, Reinforcement Learning and Motion Planning](https://github.com/eleurent/phd-bibliography)
[mintOC](https://mintoc.de/index.php/Main_Page)
Julia: Jump + InfiniteOpt
Jump is powerfull!!!
[jump-dev/JuMP.jl: Modeling language for Mathematical Optimization (linear, mixed-integer, conic, semidefinite, nonlinear)](https://github.com/jump-dev/JuMP.jl)
InfiniteOpt is powerfull!!!
[pulsipher/InfiniteOpt.jl: An intuitive modeling interface for infinite-dimensional optimization problems.](https://github.com/pulsipher/InfiniteOpt.jl)
GAMS unified software[GAMS Documentation Center](https://www.gams.com/latest/docs/index.html)
[GAMS-dev/gams.jl: A MathOptInterface Optimizer to solve JuMP models using GAMS](https://github.com/GAMS-dev/gams.jl)
Matlab: Yalmip unified[YALMIP](https://yalmip.github.io/)
Python: unified[Pyomo/pyomo: An object-oriented algebraic modeling language in Python for structured optimization problems.](https://github.com/Pyomo/pyomo)
[Solver Manuals](https://www.gams.com/latest/docs/S_MAIN.html)
Julia:
[martinbiel/StochasticPrograms.jl: Julia package for formulating and analyzing stochastic recourse models.](https://github.com/martinbiel/StochasticPrograms.jl)
[odow/SDDP.jl: Stochastic Dual Dynamic Programming in Julia](https://github.com/odow/SDDP.jl)
[PSORLab/EAGO.jl: A development environment for robust and global optimization](https://github.com/PSORLab/EAGO.jl)
[JuliaSmoothOptimizers/PDENLPModels.jl: A NLPModel API for optimization problems with PDE-constraints](https://github.com/JuliaSmoothOptimizers/PDENLPModels.jl)
[JuliaControl](https://github.com/JuliaControl)
[JuliaMPC/NLOptControl.jl: nonlinear control optimization tool](https://github.com/JuliaMPC/NLOptControl.jl)
Python:
casadi is powerful!
[python-control/python-control: The Python Control Systems Library is a Python module that implements basic operations for analysis and design of feedback control systems.](https://github.com/python-control/python-control)
[Shunichi09/PythonLinearNonlinearControl: PythonLinearNonLinearControl is a library implementing the linear and nonlinear control theories in python.](https://github.com/Shunichi09/PythonLinearNonlinearControl)
Matlab:
[OpenOCL/OpenOCL: Open Optimal Control Library for Matlab. Trajectory Optimization and non-linear Model Predictive Control (MPC) toolbox.](https://github.com/OpenOCL/OpenOCL)
[jkoendev/optimal-control-literature-software: List of literature and software for optimal control and numerical optimization.](https://github.com/jkoendev/optimal-control-literature-software)
## 3.4. Bayesian Inference
[StatisticalRethinkingJulia](https://github.com/StatisticalRethinkingJulia)
[StanJulia](https://github.com/StanJulia)
Julia:
[The Turing Language](https://github.com/TuringLang)
[cscherrer/Soss.jl: Probabilistic programming via source rewriting](https://github.com/cscherrer/Soss.jl)
[probcomp/Gen.jl: A general-purpose probabilistic programming system with programmable inference](https://github.com/probcomp/Gen.jl)
[Laboratory of Applied Mathematical Programming and Statistics](https://github.com/LAMPSPUC)
[BIASlab](https://github.com/biaslab)
[StatisticalRethinkingJulia/StatisticalRethinking.jl: Julia package with selected functions in the R package `rethinking`. Used in the SR2... projects.](https://github.com/StatisticalRethinkingJulia/StatisticalRethinking.jl)
Python:
[pymc-devs/pymc: Probabilistic Programming in Python: Bayesian Modeling and Probabilistic Machine Learning with Aesara](https://github.com/pymc-devs/pymc)
[pints-team/pints: Probabilistic Inference on Noisy Time Series](https://github.com/pints-team/pints)
[pyro-ppl/pyro: Deep universal probabilistic programming with Python and PyTorch](https://github.com/pyro-ppl/pyro)
[tensorflow/probability: Probabilistic reasoning and statistical analysis in TensorFlow](https://github.com/tensorflow/probability)
[thu-ml/zhusuan: A probabilistic programming library for Bayesian deep learning, generative models, based on Tensorflow](https://github.com/thu-ml/zhusuan)
[jmschrei/pomegranate: Fast, flexible and easy to use probabilistic modelling in Python.](https://github.com/jmschrei/pomegranate)
### 3.4.1. MCMC
Methods like HMC, SGLD are Covered by above-mentioned packages.
Julia:
[mauro3/KissMCMC.jl: Keep it simple, stupid, MCMC](https://github.com/mauro3/KissMCMC.jl)
[BigBayes/SGMCMC.jl: Stochastic Gradient Markov Chain Monte Carlo and Optimisation](https://github.com/BigBayes/SGMCMC.jl)
[tpapp/DynamicHMC.jl: Implementation of robust dynamic Hamiltonian Monte Carlo methods (NUTS) in Julia.](https://github.com/tpapp/DynamicHMC.jl)
emcee[madsjulia/AffineInvariantMCMC.jl: Affine Invariant Markov Chain Monte Carlo (MCMC) Ensemble sampler](https://github.com/madsjulia/AffineInvariantMCMC.jl)
[TuringLang/EllipticalSliceSampling.jl: Julia implementation of elliptical slice sampling.](https://github.com/TuringLang/EllipticalSliceSampling.jl)
Nested Sampling[TuringLang/NestedSamplers.jl: Implementations of single and multi-ellipsoid nested sampling](https://github.com/TuringLang/NestedSamplers.jl)
[bat/UltraNest.jl: Julia wrapper for UltraNest: advanced nested sampling for model comparison and parameter estimation](https://github.com/bat/UltraNest.jl)
Python:
[AdamCobb/hamiltorch: PyTorch-based library for Riemannian Manifold Hamiltonian Monte Carlo (RMHMC) and inference in Bayesian neural networks](https://github.com/AdamCobb/hamiltorch)
[jeremiecoullon/SGMCMCJax: Lightweight library of stochastic gradient MCMC algorithms written in JAX.](https://github.com/jeremiecoullon/SGMCMCJax)
Nested Sampling[joshspeagle/dynesty: Dynamic Nested Sampling package for computing Bayesian posteriors and evidences](https://github.com/joshspeagle/dynesty)
[JohannesBuchner/UltraNest: Fit and compare complex models reliably and rapidly. Advanced nested sampling.](https://github.com/JohannesBuchner/UltraNest)
[dfm/emcee: The Python ensemble sampling toolkit for affine-invariant MCMC](https://github.com/dfm/emcee)
[joshspeagle/dynesty: Dynamic Nested Sampling package for computing Bayesian posteriors and evidences](https://github.com/joshspeagle/dynesty)
### 3.4.2. Approximate Bayesian Computation (ABC)
Also called likelihood free or simulation based methods
Julia: (few)
[tanhevg/GpABC.jl](https://github.com/tanhevg/GpABC.jl)
[marcjwilliams1/ApproxBayes.jl: Approximate Bayesian Computation (ABC) algorithms for likelihood free inference in julia](https://github.com/marcjwilliams1/ApproxBayes.jl)
[francescoalemanno/KissABC.jl: Pure julia implementation of Multiple Affine Invariant Sampling for efficient Approximate Bayesian Computation](https://github.com/francescoalemanno/KissABC.jl)
Python:
[sbi-benchmark/sbibm: Simulation-based inference benchmark](https://github.com/sbi-benchmark/sbibm)
[elfi-dev/elfi: ELFI - Engine for Likelihood-Free Inference](https://github.com/elfi-dev/elfi)
[eth-cscs/abcpy: ABCpy package](https://github.com/eth-cscs/abcpy)
[pints-team/pints: Probabilistic Inference on Noisy Time Series](https://github.com/pints-team/pints)
[mackelab/sbi: Simulation-based inference in PyTorch](https://github.com/mackelab/sbi)
[ICB-DCM/pyABC: distributed, likelihood-free inference](https://github.com/ICB-DCM/pyABC)
### 3.4.3. Data Assimilation (SMC, particles filter)
Julia:
[Alexander-Barth/DataAssim.jl: Implementation of various ensemble Kalman Filter data assimilation methods in Julia](https://github.com/Alexander-Barth/DataAssim.jl)
[baggepinnen/LowLevelParticleFilters.jl: Simple particle/kalman filtering, smoothing and parameter estimation](https://github.com/baggepinnen/LowLevelParticleFilters.jl)
[JuliaGNSS/KalmanFilters.jl: Various Kalman Filters: KF, UKF, AUKF and their Square root variant](https://github.com/JuliaGNSS/KalmanFilters.jl)
[CliMA/EnsembleKalmanProcesses.jl: Implements Optimization and approximate uncertainty quantification algorithms, Ensemble Kalman Inversion, and Ensemble Kalman Processes.](https://github.com/CliMA/EnsembleKalmanProcesses.jl)
[FRBNY-DSGE/StateSpaceRoutines.jl: Package implementing common state-space routines.](https://github.com/FRBNY-DSGE/StateSpaceRoutines.jl)
[simsurace/FeedbackParticleFilters.jl: A Julia package that provides (feedback) particle filters for nonlinear stochastic filtering and data assimilation problems](https://github.com/simsurace/FeedbackParticleFilters.jl)
[mjb3/DiscretePOMP.jl: Bayesian inference for Discrete state-space Partially Observed Markov Processes in Julia. See the docs:](https://github.com/mjb3/DiscretePOMP.jl)
Python:
[nchopin/particles: Sequential Monte Carlo in python](https://github.com/nchopin/particles)
[rlabbe/filterpy: Python Kalman filtering and optimal estimation library. Implements Kalman filter, particle filter, Extended Kalman filter, Unscented Kalman filter, g-h (alpha-beta), least squares, H Infinity, smoothers, and more. Has companion book 'Kalman and Bayesian Filters in Python'.](https://github.com/rlabbe/filterpy)
[tingiskhan/pyfilter: Particle filtering and sequential parameter inference in Python](https://github.com/tingiskhan/pyfilter)
### 3.4.4. Variational Inference
Julia:
[bat/MGVI.jl: Metric Gaussian Variational Inference](https://github.com/bat/MGVI.jl)
[TuringLang/AdvancedVI.jl: A library for variational Bayesian methods in Julia](https://github.com/TuringLang/AdvancedVI.jl)
[ngiann/ApproximateVI.jl: Approximate variational inference in Julia](https://github.com/ngiann/ApproximateVI.jl)
Python:
### 3.4.5. Gaussion, non-Gaussion and Kernel
Julia:
[Gaussian Processes for Machine Learning in Julia](https://github.com/JuliaGaussianProcesses)
[Laboratory of Applied Mathematical Programming and Statistics](https://github.com/LAMPSPUC)
[JuliaRobotics](https://github.com/JuliaRobotics)
[JuliaStats/KernelDensity.jl: Kernel density estimators for Julia](https://github.com/JuliaStats/KernelDensity.jl)
[JuliaRobotics/KernelDensityEstimate.jl: Kernel Density Estimate with product approximation using multiscale Gibbs sampling](https://github.com/JuliaRobotics/KernelDensityEstimate.jl)
[theogf/AugmentedGaussianProcesses.jl: Gaussian Process package based on data augmentation, sparsity and natural gradients](https://github.com/theogf/AugmentedGaussianProcesses.jl)
[JuliaGaussianProcesses/TemporalGPs.jl: Fast inference for Gaussian processes in problems involving time](https://github.com/JuliaGaussianProcesses/TemporalGPs.jl)
[aterenin/SparseGaussianProcesses.jl: A Julia implementation of sparse Gaussian processes via path-wise doubly stochastic variational inference.](https://github.com/aterenin/SparseGaussianProcesses.jl)
[PieterjanRobbe/GaussianRandomFields.jl: A package for Gaussian random field generation in Julia](https://github.com/PieterjanRobbe/GaussianRandomFields.jl)
[JuliaGaussianProcesses/Stheno.jl: Probabilistic Programming with Gaussian processes in Julia](https://github.com/JuliaGaussianProcesses/Stheno.jl)
[STOR-i/GaussianProcesses.jl: A Julia package for Gaussian Processes](https://github.com/STOR-i/GaussianProcesses.jl)
Python:
[cornellius-gp/gpytorch: A highly efficient and modular implementation of Gaussian Processes in PyTorch](https://github.com/cornellius-gp/gpytorch)
[GPflow/GPflow: Gaussian processes in TensorFlow](https://github.com/GPflow/GPflow)
[SheffieldML/GPy: Gaussian processes framework in python](https://github.com/SheffieldML/GPy)
### 3.4.6. Bayesian Optimization
Julia:
[SciML/Surrogates.jl: Surrogate modeling and optimization for scientific machine learning (SciML)](https://github.com/SciML/Surrogates.jl)
[jbrea/BayesianOptimization.jl: Bayesian optimization for Julia](https://github.com/jbrea/BayesianOptimization.jl)
[baggepinnen/Hyperopt.jl: Hyperparameter optimization in Julia.](https://github.com/baggepinnen/Hyperopt.jl)
Python:
[fmfn/BayesianOptimization: A Python implementation of global optimization with gaussian processes.](https://github.com/fmfn/BayesianOptimization)
[pytorch/botorch: Bayesian optimization in PyTorch](https://github.com/pytorch/botorch)
[optuna/optuna: A hyperparameter optimization framework](https://github.com/optuna/optuna)
[huawei-noah/HEBO: Bayesian optimisation library developped by Huawei Noah's Ark Library](https://github.com/huawei-noah/HEBO)
### 3.4.7. Information theory
Julia:
entropy and kldivengence for distributions or vectors can be seen in Distributions.jlKL divergence for functions[RafaelArutjunjan/InformationGeometry.jl: Methods for computational information geometry](https://github.com/RafaelArutjunjan/InformationGeometry.jl)
not maintained[kzahedi/Shannon.jl: Entropy, Mutual Information, KL-Divergence related to Shannon's information theory and functions to binarize data](https://github.com/kzahedi/Shannon.jl)
[gragusa/Divergences.jl: A Julia package for evaluation of divergences between distributions](https://github.com/gragusa/Divergences.jl)
[Tchanders/InformationMeasures.jl: Entropy, mutual information and higher order measures from information theory, with various estimators and discretisation methods.](https://github.com/Tchanders/InformationMeasures.jl)
[JuliaDynamics/TransferEntropy.jl: Transfer entropy (conditional mutual information) estimators for the Julia language](https://github.com/JuliaDynamics/TransferEntropy.jl)
[cynddl/Discreet.jl: A Julia package to estimate discrete entropy and mutual information](https://github.com/cynddl/Discreet.jl)
### 3.4.8. Uncertanty
Julia:
[uncertainty-toolbox/uncertainty-toolbox: A python toolbox for predictive uncertainty quantification, calibration, metrics, and visualization](https://github.com/uncertainty-toolbox/uncertainty-toolbox)
[JuliaPhysics/Measurements.jl: Error propagation calculator and library for physical measurements. It supports real and complex numbers with uncertainty, arbitrary precision calculations, operations with arrays, and numerical integration.](https://github.com/JuliaPhysics/Measurements.jl)
### 3.4.9. Casual
[zenna/Omega.jl: Causal, Higher-Order, Probabilistic Programming](https://github.com/zenna/Omega.jl)
[mschauer/CausalInference.jl: Causal inference, graphical models and structure learning with the PC algorithm.](https://github.com/mschauer/CausalInference.jl)
[JuliaDynamics/CausalityTools.jl: Algorithms for causal inference and the detection of dynamical coupling from time series, and for approximation of the transfer operator and invariant measures.](https://github.com/JuliaDynamics/CausalityTools.jl)
python
Review: [rguo12/awesome-causality-algorithms: An index of algorithms for learning causality with data](https://github.com/rguo12/awesome-causality-algorithms)
### 3.4.10. Sampling
[MrUrq/LatinHypercubeSampling.jl: Julia package for the creation of optimised Latin Hypercube Sampling Plans](https://github.com/MrUrq/LatinHypercubeSampling.jl)
[SciML/QuasiMonteCarlo.jl: Lightweight and easy generation of quasi-Monte Carlo sequences with a ton of different methods on one API for easy parameter exploration in scientific machine learning (SciML)](https://github.com/SciML/QuasiMonteCarlo.jl)
## 3.5. Machine Learning and Deep Learning
Python:
Survey [ritchieng/the-incredible-pytorch at pythonrepo.com](https://github.com/ritchieng/the-incredible-pytorch?ref=pythonrepo.com#GANsVAEsandAEs)
### 3.5.1. Machine Learning
Julia: MLJ is enough
[alan-turing-institute/MLJ.jl: A Julia machine learning framework](https://github.com/alan-turing-institute/MLJ.jl)
[JuliaML](https://github.com/JuliaML)
[JuliaAI](https://github.com/JuliaAI)
[Evovest/EvoTrees.jl: Boosted trees in Julia](https://github.com/Evovest/EvoTrees.jl)
Dimention Reduction:[madeleineudell/LowRankModels.jl: LowRankModels.jl is a julia package for modeling and fitting generalized low rank models.](https://github.com/madeleineudell/LowRankModels.jl)
Linear Regression[JuliaAI/MLJLinearModels.jl: Generalized Linear Regressions Models (penalized regressions, robust regressions, ...)](https://github.com/JuliaAI/MLJLinearModels.jl)
[gerdm/pknn.jl: Probabilistic k-nearest neighbours](https://github.com/gerdm/pknn.jl)
[IBM/AutoMLPipeline.jl: A package that makes it trivial to create and evaluate machine learning pipeline architectures.](https://github.com/IBM/AutoMLPipeline.jl)
Python:
[scikit-learn: machine learning in Python — scikit-learn 1.0.1 documentation](https://scikit-learn.org/stable/)
[automl/auto-sklearn: Automated Machine Learning with scikit-learn](https://github.com/automl/auto-sklearn)
[h2oai/h2o-3: H2O is an Open Source, Distributed, Fast & Scalable Machine Learning Platform: Deep Learning, Gradient Boosting (GBM) & XGBoost, Random Forest, Generalized Linear Modeling (GLM with Elastic Net), K-Means, PCA, Generalized Additive Models (GAM), RuleFit, Support Vector Machine (SVM), Stacked Ensembles, Automatic Machine Learning (AutoML), etc.](https://github.com/h2oai/h2o-3)
[pycaret/pycaret: An open-source, low-code machine learning library in Python](https://github.com/pycaret/pycaret)
[nubank/fklearn: fklearn: Functional Machine Learning](https://github.com/nubank/fklearn)
[wecarsoniv/augmented-pca: Repository for the AugmentedPCA Python package.](https://github.com/wecarsoniv/augmented-pca)
Data Generation
[snorkel-team/snorkel: A system for quickly generating training data with weak supervision](https://github.com/snorkel-team/snorkel)
[lk-geimfari/mimesis: Mimesis is a high-performance fake data generator for Python, which provides data for a variety of purposes in a variety of languages.](https://github.com/lk-geimfari/mimesis)
### 3.5.2. Deep Learning
Julia: Flux and Knet
[FluxML/Flux.jl: Relax! Flux is the ML library that doesn't make you tensor](https://github.com/FluxML/Flux.jl)
[sdobber/FluxArchitectures.jl: Complex neural network examples for Flux.jl](https://github.com/sdobber/FluxArchitectures.jl)
[denizyuret/Knet.jl: Koç University deep learning framework.](https://github.com/denizyuret/Knet.jl)
Python: Jax, Pytorch, Tensorflow
[google/jax: Composable transformations of Python+NumPy programs: differentiate, vectorize, JIT to GPU/TPU, and more](https://github.com/google/jax)
[pytorch/pytorch: Tensors and Dynamic neural networks in Python with strong GPU acceleration](https://github.com/pytorch/pytorch)
[tensorflow/tensorflow: An Open Source Machine Learning Framework for Everyone](https://github.com/tensorflow/tensorflow)
[catalyst-team/catalyst: Accelerated deep learning R&D](https://github.com/catalyst-team/catalyst)
[murufeng/awesome_lightweight_networks: MobileNetV1-V2,MobileNeXt,GhostNet,AdderNet,ShuffleNetV1-V2,Mobile+ViT etc. ⭐⭐⭐⭐⭐](https://github.com/murufeng/awesome_lightweight_networks)
### 3.5.3. Reinforce Learning
Julia:
[JuliaPOMDP](https://github.com/JuliaPOMDP)
[JuliaReinforcementLearning](https://github.com/JuliaReinforcementLearning)
Python:
[ray-project/ray: An open source framework that provides a simple, universal API for building distributed applications. Ray is packaged with RLlib, a scalable reinforcement learning library, and Tune, a scalable hyperparameter tuning library.](https://github.com/ray-project/ray)
[tensorlayer/tensorlayer: Deep Learning and Reinforcement Learning Library for Scientists and Engineers 🔥](https://github.com/tensorlayer/tensorlayer)
[pfnet/pfrl: PFRL: a PyTorch-based deep reinforcement learning library](https://github.com/pfnet/pfrl)
### 3.5.4. GNN
Julia:
[CarloLucibello/GraphNeuralNetworks.jl: Graph Neural Networks in Julia](https://github.com/CarloLucibello/GraphNeuralNetworks.jl)
[FluxML/GeometricFlux.jl: Geometric Deep Learning for Flux](https://github.com/FluxML/GeometricFlux.jl)
Python:
[pyg-team/pytorch_geometric: Graph Neural Network Library for PyTorch](https://github.com/pyg-team/pytorch_geometric)
[benedekrozemberczki/pytorch_geometric_temporal: PyTorch Geometric Temporal: Spatiotemporal Signal Processing with Neural Machine Learning Models (CIKM 2021)](https://github.com/benedekrozemberczki/pytorch_geometric_temporal)
[dmlc/dgl: Python package built to ease deep learning on graph, on top of existing DL frameworks.](https://github.com/dmlc/dgl)
[THUDM/cogdl: CogDL: An Extensive Toolkit for Deep Learning on Graphs](https://github.com/THUDM/cogdl)
### 3.5.5. Transformer
Julia:
[chengchingwen/Transformers.jl: Julia Implementation of Transformer models](https://github.com/chengchingwen/Transformers.jl)
Python:
[huggingface/transformers: 🤗 Transformers: State-of-the-art Natural Language Processing for Pytorch, TensorFlow, and JAX.](https://github.com/huggingface/transformers)
### 3.5.6. Transfer Learning
Survey[jindongwang/transferlearning: Transfer learning / domain adaptation / domain generalization / multi-task learning etc. papers, codes. datasets, applications, tutorials.-迁移学习](https://github.com/jindongwang/transferlearning)
### 3.5.7. Neural Tangent
Python:
[google/neural-tangents: Fast and Easy Infinite Neural Networks in Python](https://github.com/google/neural-tangents)
### 3.5.8. Visulization
Python:
[ashishpatel26/Tools-to-Design-or-Visualize-Architecture-of-Neural-Network: Tools to Design or Visualize Architecture of Neural Network](https://github.com/ashishpatel26/Tools-to-Design-or-Visualize-Architecture-of-Neural-Network)
[julrog/nn_vis: A project for processing neural networks and rendering to gain insights on the architecture and parameters of a model through a decluttered representation.](https://github.com/julrog/nn_vis)
PowerPoints[dair-ai/ml-visuals: 🎨 ML Visuals contains figures and templates which you can reuse and customize to improve your scientific writing.](https://github.com/dair-ai/ml-visuals)
### Semi-supervised Learning
Python:
[TorchSSL/TorchSSL: A PyTorch-based library for semi-supervised learning (NeurIPS'21)](https://github.com/TorchSSL/TorchSSL)
## 3.6. Probablistic Machine Learning and Deep Learning
Julia:
[mcosovic/FactorGraph.jl: The FactorGraph package provides the set of different functions to perform inference over the factor graph with continuous or discrete random variables using the belief propagation algorithm.](https://github.com/mcosovic/FactorGraph.jl)
[stefan-m-lenz/BoltzmannMachines.jl: A Julia package for training and evaluating multimodal deep Boltzmann machines](https://github.com/stefan-m-lenz/BoltzmannMachines.jl)
[BIASlab](https://github.com/biaslab)
[biaslab/ReactiveMP.jl: Julia package for automatic Bayesian inference on a factor graph with reactive message passing](https://github.com/biaslab/ReactiveMP.jl)
Python:
[Probabilistic machine learning](https://github.com/probml)
[thu-ml/zhusuan: A probabilistic programming library for Bayesian deep learning, generative models, based on Tensorflow](https://github.com/thu-ml/zhusuan)
[OATML/bdl-benchmarks: Bayesian Deep Learning Benchmarks](https://github.com/OATML/bdl-benchmarks)
[pgmpy/pgmpy: Python Library for learning (Structure and Parameter) and inference (Probabilistic and Causal) in Bayesian Networks.](https://github.com/pgmpy/pgmpy)
[scikit-learn-contrib/imbalanced-learn: A Python Package to Tackle the Curse of Imbalanced Datasets in Machine Learning](https://github.com/scikit-learn-contrib/imbalanced-learn)
### 3.6.1. GAN
Julia:
Python:
[torchgan/torchgan: Research Framework for easy and efficient training of GANs based on Pytorch](file:///F:/Zotero/Zotero/storage/DMJ4DGLN/torchgan.html)
[kwotsin/mimicry: [CVPR 2020 Workshop] A PyTorch GAN library that reproduces research results for popular GANs.](https://github.com/kwotsin/mimicry)
### 3.6.2. Normilization Flows
Julia:
[TuringLang/Bijectors.jl: Implementation of normalising flows and constrained random variable transformations](https://github.com/TuringLang/Bijectors.jl)
[slimgroup/InvertibleNetworks.jl: A Julia framework for invertible neural networks](https://github.com/slimgroup/InvertibleNetworks.jl)
FFJord is impleted in DiffEqFlux.jl
Python:
Survey[janosh/awesome-normalizing-flows: A list of awesome resources on normalizing flows.](https://github.com/janosh/awesome-normalizing-flows)
[RameenAbdal/StyleFlow: StyleFlow: Attribute-conditioned Exploration of StyleGAN-generated Images using Conditional Continuous Normalizing Flows (ACM TOG 2021)](https://github.com/RameenAbdal/StyleFlow)
### 3.6.3. VAE
Julia:
Python:
[Variational Autoencoders — Pyro Tutorials 1.7.0 documentation](https://pyro.ai/examples/vae.html)
[AntixK/PyTorch-VAE: A Collection of Variational Autoencoders (VAE) in PyTorch.](https://github.com/AntixK/PyTorch-VAE)
[timsainb/tensorflow2-generative-models: Implementations of a number of generative models in Tensorflow 2. GAN, VAE, Seq2Seq, VAEGAN, GAIA, Spectrogram Inversion. Everything is self contained in a jupyter notebook for easy export to colab.](https://github.com/timsainb/tensorflow2-generative-models)
[altosaar/variational-autoencoder: Variational autoencoder implemented in tensorflow and pytorch (including inverse autoregressive flow)](https://github.com/altosaar/variational-autoencoder)
[subinium/Pytorch-AutoEncoders at pythonrepo.com](https://github.com/subinium/Pytorch-AutoEncoders?ref=pythonrepo.com)
[Ritvik19/pyradox-generative at pythonrepo.com](https://github.com/Ritvik19/pyradox-generative?ref=pythonrepo.com)
### 3.6.4 BNN
[JavierAntoran/Bayesian-Neural-Networks: Pytorch implementations of Bayes By Backprop, MC Dropout, SGLD, the Local Reparametrization Trick, KF-Laplace, SG-HMC and more](https://github.com/JavierAntoran/Bayesian-Neural-Networks)
[RajDandekar/MSML21_BayesianNODE](https://github.com/RajDandekar/MSML21_BayesianNODE)
[bayesian-neural-networks · GitHub Topics](https://github.com/topics/bayesian-neural-networks)
## 3.7. Differential Equations and Scientific Computation
Julia:
All you need is the following organization (My Idol Prof. Christopher Rackauckas):
[SciML Open Source Scientific Machine Learning](https://github.com/SciML)
Including agent based models
[JuliaDynamics](https://github.com/JuliaDynamics)[BioJulia](https://github.com/BioJulia)
[nathanaelbosch/ProbNumDiffEq.jl: Probabilistic ODE Solvers via Bayesian Filtering and Smoothing](https://github.com/nathanaelbosch/ProbNumDiffEq.jl)
[PerezHz/TaylorIntegration.jl: ODE integration using Taylor's method, and more, in Julia](https://github.com/PerezHz/TaylorIntegration.jl)
[gideonsimpson/BasicMD.jl: A collection of basic routines for Molecular Dynamics simulations implemented in Julia](https://github.com/gideonsimpson/BasicMD.jl)
Probablistic Numerical Methods:
Julia:
[nathanaelbosch/ProbNumDiffEq.jl: Probabilistic ODE Solvers via Bayesian Filtering and Smoothing](https://github.com/nathanaelbosch/ProbNumDiffEq.jl)
Python:
[ProbNum — probnum 0.1 documentation](http://www.probabilistic-numerics.org/en/latest/)
### 3.7.1. Partial differential equation
Survey[JuliaPDE/SurveyofPDEPackages: Survey of the packages of the Julia ecosystem for solving partial differential equations](https://github.com/JuliaPDE/SurveyofPDEPackages)
[SciML/DiffEqOperators.jl: Linear operators for discretizations of differential equations and scientific machine learning (SciML)](https://github.com/SciML/DiffEqOperators.jl)
[vavrines/Kinetic.jl: Universal modeling and simulation of fluid dynamics upon machine learning](https://github.com/vavrines/Kinetic.jl)
[Gridap](https://github.com/gridap)
[kailaix/AdFem.jl: Innovative, efficient, and computational-graph-based finite element simulator for inverse modeling](https://github.com/kailaix/AdFem.jl)
[SciML/ExponentialUtilities.jl: Utility functions for exponential integrators for the SciML scientific machine learning ecosystem](https://github.com/SciML/ExponentialUtilities.jl)
[trixi-framework/Trixi.jl: Trixi.jl: Adaptive high-order numerical simulations of hyperbolic PDEs in Julia](https://github.com/trixi-framework/Trixi.jl)
[JuliaIBPM](file:///F:/Zotero/Zotero/storage/FB6Y7GEQ/JuliaIBPM.html)
[ranocha/SummationByPartsOperators.jl: A Julia library of summation-by-parts (SBP) operators used in finite difference, Fourier pseudospectral, continuous Galerkin, and discontinuous Galerkin methods to get provably stable semidiscretizations, paying special attention to boundary conditions.](https://github.com/ranocha/SummationByPartsOperators.jl)
[Ferrite-FEM/Ferrite.jl: Finite element toolbox for Julia](https://github.com/Ferrite-FEM/Ferrite.jl)
[JuliaFEM](https://github.com/JuliaFEM)
Python:
[DedalusProject/dedalus: A flexible framework for solving PDEs with modern spectral methods.](https://github.com/DedalusProject/dedalus)
### 3.7.2 Fractional Differential and Calculus
Julia
[SciFracX](https://github.com/SciFracX)
[SciFracX/FractionalDiffEq.jl: FractionalDiffEq.jl: A Julia package aiming at solving Fractional Differential Equations using high performance numerical methods](https://github.com/SciFracX/FractionalDiffEq.jl)
[SciFracX/FractionalSystems.jl: Fractional order modeling and analysis in Julia.](https://github.com/SciFracX/FractionalSystems.jl)
[SciFracX/FractionalCalculus.jl: FractionalCalculus.jl: A Julia package for high performance, fast convergence and high precision numerical fractional calculus computing.](https://github.com/SciFracX/FractionalCalculus.jl)
[SciFracX/FractionalTransforms.jl: FractionalTransforms.jl: A Julia package aiming at providing fractional order transforms with high performance.](https://github.com/SciFracX/FractionalTransforms.jl)
## 3.8. Scientific Machine Learning (Differential Equation and ML)
[Zymrael/awesome-neural-ode: A collection of resources regarding the interplay between differential equations, deep learning, dynamical systems, control and numerical methods.](https://github.com/Zymrael/awesome-neural-ode)
[massastrello/awesome-implicit-neural-models](https://github.com/massastrello/awesome-implicit-neural-models)
### 3.8.1. Universal Differential Equations. (Neural differential equations)
Julia:
[SciML/DiffEqFlux.jl: Universal neural differential equations with O(1) backprop, GPUs, and stiff+non-stiff DE solvers, demonstrating scientific machine learning (SciML) and physics-informed machine learning methods](https://github.com/SciML/DiffEqFlux.jl)
[avik-pal/FastDEQ.jl: Deep Equilibrium Networks (but faster!!!)](https://github.com/avik-pal/FastDEQ.jl)
UDE with Gaussion Process[Crown421/GPDiffEq.jl](https://github.com/Crown421/GPDiffEq.jl)
Python:
[DiffEqML/torchdyn: A PyTorch based library for all things neural differential equations and implicit neural models.](https://github.com/DiffEqML/torchdyn)
[rtqichen/torchdiffeq: Differentiable ODE solvers with full GPU support and O(1)-memory backpropagation.](https://github.com/rtqichen/torchdiffeq)
[patrick-kidger/diffrax at zzun.app](https://github.com/patrick-kidger/diffrax?ref=zzun.app)
### 3.8.2. Physical Informed Neural Netwworks
[Predictive Intelligence Lab](https://github.com/PredictiveIntelligenceLab)
Julia:
[SciML/NeuralPDE.jl: Physics-Informed Neural Networks (PINN) and Deep BSDE Solvers of Differential Equations for Scientific Machine Learning (SciML) accelerated simulation](https://github.com/SciML/NeuralPDE.jl)
Python:
[lululxvi/deepxde: Deep learning library for solving differential equations and more](https://github.com/lululxvi/deepxde)
[sciann/sciann: Deep learning for Engineers - Physics Informed Deep Learning](https://github.com/sciann/sciann)
### 3.8.3. Neural Operator
Julia:
[foldfelis/NeuralOperators.jl: learning the solution operator for partial differential equations in pure Julia.](https://github.com/foldfelis/NeuralOperators.jl)
[CliMA/OperatorFlux.jl: Operator layers for Flux.jl](https://github.com/CliMA/OperatorFlux.jl)
[brekmeuris/DrMZ.jl: Deep renormalized Mori-Zwanzig (DrMZ) Julia package.](https://github.com/brekmeuris/DrMZ.jl)
## 3.9. Data Driven Methods (Equation Searching Methods)
Julia package including SINDy, Symbolic Regression, DMD
[SciML/DataDrivenDiffEq.jl: Data driven modeling and automated discovery of dynamical systems for the SciML Scientific Machine Learning organization](https://github.com/SciML/DataDrivenDiffEq.jl)
[nmheim/NeuralArithmetic.jl: Collection of layers that can perform arithmetic operations](https://github.com/nmheim/NeuralArithmetic.jl)
### 3.9.1. Symbolic Regression
[cavalab/srbench: A living benchmark framework for symbolic regression](https://github.com/cavalab/srbench)
Python:
[trevorstephens/gplearn: Genetic Programming in Python, with a scikit-learn inspired API](https://github.com/trevorstephens/gplearn)
[MilesCranmer/PySR: Simple, fast, and parallelized symbolic regression in Python/Julia via regularized evolution and simulated annealing](https://github.com/MilesCranmer/PySR)
Julia:
[MilesCranmer/SymbolicRegression.jl: Distributed High-Performance symbolic regression in Julia](https://github.com/MilesCranmer/SymbolicRegression.jl)
[sisl/ExprOptimization.jl: Algorithms for optimization of Julia expressions](https://github.com/sisl/ExprOptimization.jl)
### 3.9.2. SINDy (Sparse Identification of Nonlinear Dynamics from Data)
[dynamicslab/pysindy: A package for the sparse identification of nonlinear dynamical systems from data](https://github.com/dynamicslab/pysindy)
[dynamicslab/modified-SINDy: Example code for paper: Automatic Differentiation to Simultaneously Identify Nonlinear Dynamics and Extract Noise Probability Distributions from Data](https://github.com/dynamicslab/modified-SINDy)
### 3.9.3. DMD (Dynamic Mode Decomposition)
[mathLab/PyDMD: Python Dynamic Mode Decomposition](https://github.com/mathLab/PyDMD)
[foldfelis/NeuralOperators.jl: learning the solution operator for partial differential equations in pure Julia.](https://github.com/foldfelis/NeuralOperators.jl)
## 3.10. Model Evaluation
### 3.10.1. Structure Idendification
Julia:
[SciML/StructuralIdentifiability.jl](https://github.com/SciML/StructuralIdentifiability.jl)
[alexeyovchinnikov/SIAN-Julia: Implementation of SIAN in Julia](https://github.com/alexeyovchinnikov/SIAN-Julia)
### 3.10.2. Global Sensitivity Anylysis
Julia:
[lrennels/GlobalSensitivityAnalysis.jl: Julia implementations of global sensitivity analysis methods.](https://github.com/lrennels/GlobalSensitivityAnalysis.jl)
[SciML/GlobalSensitivity.jl](https://github.com/SciML/GlobalSensitivity.jl)
[SciML/DiffEqSensitivity.jl: A component of the DiffEq ecosystem for enabling sensitivity analysis for scientific machine learning (SciML). Optimize-then-discretize, discretize-then-optimize, and more for ODEs, SDEs, DDEs, DAEs, etc.](https://github.com/SciML/DiffEqSensitivity.jl)
Python:
[SALib/SALib: Sensitivity Analysis Library in Python. Contains Sobol, Morris, FAST, and other methods.](https://github.com/SALib/SALib)
R:
sensitivity
fast
sensobol
## 3.11. Optimal Transportation
Julia:
[Optimal transport in Julia](https://github.com/JuliaOptimalTransport)
[JuliaOptimalTransport/OptimalTransport.jl: Optimal transport algorithms for Julia](https://github.com/JuliaOptimalTransport/OptimalTransport.jl)
[JuliaOptimalTransport/ExactOptimalTransport.jl: Solving unregularized optimal transport problems with Julia](https://github.com/JuliaOptimalTransport/ExactOptimalTransport.jl)
Python:
[PythonOT/POT: POT : Python Optimal Transport](https://github.com/PythonOT/POT)
[ott-jax/ott](https://github.com/ott-jax/ott)
## 3.12. Agents, Graph and Networks
[Computational Modeling Software Frameworks](https://www.comses.net/resources/modeling-frameworks/)
Julia:
[JuliaDynamics/Agents.jl: Agent-based modeling framework in Julia](https://github.com/JuliaDynamics/Agents.jl)
Python:
[projectmesa/mesa: Mesa is an agent-based modeling framework in Python](https://github.com/projectmesa/mesa)
Network
[briatte/awesome-network-analysis: A curated list of awesome network analysis resources.](https://github.com/briatte/awesome-network-analysis#julia)
Python:
[networkx/networkx: Network Analysis in Python](https://github.com/networkx/networkx)
[GiulioRossetti/ndlib: Network Diffusion Library - (for NetworkX and iGraph)](https://github.com/GiulioRossetti/ndlib)
[Welcome to Epidemics on Networks’s documentation! — Epidemics on Networks 1.2rc1 documentation](https://epidemicsonnetworks.readthedocs.io/en/latest/index.html)
[寻找人类传播行为的基因 — 计算传播学](https://chengjun.github.io/mybook/)
# 4. Theoretical Analysis
Julia:
[Julia Math](https://github.com/JuliaMath)
[JuliaApproximation](https://github.com/JuliaApproximation)
Python:
[sympy/sympy: A computer algebra system written in pure Python](https://github.com/sympy/sympy)
## 4.0. Special Functions
Julia:
[JuliaMath/SpecialFunctions.jl: Special mathematical functions in Julia](https://github.com/JuliaMath/SpecialFunctions.jl)
InverseFunction
[JuliaMath/InverseFunctions.jl: Interface for function inversion in Julia](https://github.com/JuliaMath/InverseFunctions.jl)[JuliaStats/StatsFuns.jl: Mathematical functions related to statistics.](https://github.com/JuliaStats/StatsFuns.jl)
[JuliaStats/LogExpFunctions.jl: Julia package for various special functions based on `log` and `exp`.](https://github.com/JuliaStats/LogExpFunctions.jl)
[Readme · LambertW.jl](https://docs.juliahub.com/LambertW/7mpiq/0.4.5/)
[scheinerman/Permutations.jl: Permutations class for Julia.](https://github.com/scheinerman/Permutations.jl)
## 4.1. Symbolic Computation
Julia:
[JuliaSymbolics](https://github.com/JuliaSymbolics)
[JuliaSymbolics/Symbolics.jl: A fast and modern CAS for a fast and modern language.](https://github.com/JuliaSymbolics/Symbolics.jl)
[JuliaPy/SymPy.jl: Julia interface to SymPy via PyCall](https://github.com/JuliaPy/SymPy.jl)
[jlapeyre/Symata.jl: language for symbolic mathematics](https://github.com/jlapeyre/Symata.jl)
[wbhart/AbstractAlgebra.jl: Generic abstract algebra functionality in pure Julia (no C dependencies)](https://github.com/wbhart/AbstractAlgebra.jl)
[rjrosati/SymbolicTensors.jl: Manipulate tensors symbolically in Julia! Currently needs a SymPy dependency, but work is ongoing to change the backend to SymbolicUtils.jl](https://github.com/rjrosati/SymbolicTensors.jl)
Python:
[sympy/sympy: A computer algebra system written in pure Python](https://github.com/sympy/sympy)
## 4.3. Roots, Intepolations
### 4.3.1. Roots
Julia:
All[SciML/NonlinearSolve.jl: High-performance and differentiation-enabled nonlinear solvers](https://github.com/SciML/NonlinearSolve.jl)
[SciML/SciMLNLSolve.jl: Nonlinear solver bindings for the SciML Interface](https://github.com/SciML/SciMLNLSolve.jl)
[JuliaMath/Roots.jl: Root finding functions for Julia](https://github.com/JuliaMath/Roots.jl)
[PolynomialRoots · Julia Packages](https://juliapackages.com/p/polynomialroots)
[JuliaNLSolvers/NLsolve.jl: Julia solvers for systems of nonlinear equations and mixed complementarity problems](https://github.com/JuliaNLSolvers/NLsolve.jl)
[sglyon/MINPACK.jl: Wrapper for cminpack multivariate root finding routines](https://github.com/sglyon/MINPACK.jl)
### 4.3.2. Interpolations and Approximations
Julia:
ApproxFun.jl
[PumasAI/DataInterpolations.jl: A library of data interpolation and smoothing functions](https://github.com/PumasAI/DataInterpolations.jl)
[JuliaMath/Interpolations.jl: Fast, continuous interpolation of discrete datasets in Julia](https://github.com/JuliaMath/Interpolations.jl)
[kbarbary/Dierckx.jl: Julia package for 1-d and 2-d splines](https://github.com/kbarbary/Dierckx.jl)
[sisl/GridInterpolations.jl: Multidimensional grid interpolation in arbitrary dimensions](https://github.com/sisl/GridInterpolations.jl)
[floswald/ApproXD.jl: B-splines and linear approximators in multiple dimensions for Julia](https://github.com/floswald/ApproXD.jl)
[sostock/BSplines.jl: A Julia package for working with B-splines](https://github.com/sostock/BSplines.jl)
[stevengj/FastChebInterp.jl: fast multidimensional Chebyshev interpolation and regression in Julia](https://github.com/stevengj/FastChebInterp.jl)
[jipolanco/BSplineKit.jl: A collection of B-spline tools in Julia](https://github.com/jipolanco/BSplineKit.jl)
[NFFT/ANOVAapprox.jl: Approximation Package for High-Dimensional Functions in Julia](https://github.com/NFFT/ANOVAapprox.jl)
## 4.2. Bifurcation
[rveltz/BifurcationKit.jl: A Julia package to perform Bifurcation Analysis](https://github.com/rveltz/BifurcationKit.jl)
## 4.4 Polynomials
[JuliaMath/Polynomials.jl: Polynomial manipulations in Julia](https://github.com/JuliaMath/Polynomials.jl)
# 5. Writings, Blog and Web
[JuliaDocs/Documenter.jl: A documentation generator for Julia.](https://github.com/JuliaDocs/Documenter.jl)
[chriskiehl/Gooey: Turn (almost) any Python command line program into a full GUI application with one line](https://github.com/chriskiehl/Gooey)
Latex:
[Detexify LaTeX handwritten symbol recognition](http://detexify.kirelabs.org/classify.html)
Display Julia Unicode in Latex
[mossr/julia-mono-listings: LaTeX listings style for Julia and Unicode support for the JuliaMono font](https://github.com/mossr/julia-mono-listings)
[wg030/jlcode: A latex package for displaying Julia code using the listings package. The package supports pdftex, luatex and xetex for compilation.](https://github.com/wg030/jlcode)
[davibarreira/NotebookToLaTeX.jl: A Julia package for converting your Pluto and Jupyter Notebooks into beautiful Latex.](https://github.com/davibarreira/NotebookToLaTeX.jl)
Web:
[facebook/docusaurus: Easy to maintain open source documentation websites.](https://github.com/facebook/docusaurus)
Hexo
[Jekyll • Simple, blog-aware, static sites | Transform your plain text into static websites and blogs](https://jekyllrb.com/)
[tlienart/Franklin.jl: (yet another) static site generator. Simple, customisable, fast, maths with KaTeX, code evaluation, optional pre-rendering, in Julia.](https://github.com/tlienart/Franklin.jl)
[一个傻瓜式构建可视化 web的 Python 神器 -- streamlit](https://mp.weixin.qq.com/s/AxZPxQgLfJ6g8bhonTvKxA)
[streamlit/streamlit: Streamlit — The fastest way to build data apps in Python](https://github.com/streamlit/streamlit)
[gradio-app/gradio: Create UIs for your machine learning model in Python in 3 minutes](https://github.com/gradio-app/gradio)
GitHub Profile Settings:
[abhisheknaiidu/awesome-github-profile-readme: 😎 A curated list of awesome GitHub Profile READMEs 📝](https://github.com/abhisheknaiidu/awesome-github-profile-readme)
[Shields.io: Quality metadata badges for open source projects](https://shields.io/)
[ButterAndButterfly/GithubTools: 目标是创建会刷新的ReadMe首页! 在这里,你可以得到Github star/fork总数图标, 项目star历史曲线,star数最多的前N个Repo信息...](https://github.com/ButterAndButterfly/GithubTools)
常用[anuraghazra/github-readme-stats: Dynamically generated stats for your github readmes](https://github.com/anuraghazra/github-readme-stats)
字体:
[be5invis/Sarasa-Gothic: Sarasa Gothic / 更纱黑体 / 更紗黑體 / 更紗ゴシック / 사라사 고딕](https://github.com/be5invis/Sarasa-Gothic)