https://github.com/onejune2018/awesome-llm-eval
Awesome-LLM-Eval: a curated list of tools, datasets/benchmark, demos, leaderboard, papers, docs and models, mainly for Evaluation on LLMs. 一个由工具、基准/数据、演示、排行榜和大模型等组成的精选列表,主要面向基础大模型评测,旨在探求生成式AI的技术边界.
https://github.com/onejune2018/awesome-llm-eval
List: awesome-llm-eval
awsome-list awsome-lists benchmark bert chatglm chatgpt dataset evaluation gpt3 large-language-model leaderboard llama llm llm-evaluation machine-learning nlp openai qwen rag
Last synced: 4 days ago
JSON representation
Awesome-LLM-Eval: a curated list of tools, datasets/benchmark, demos, leaderboard, papers, docs and models, mainly for Evaluation on LLMs. 一个由工具、基准/数据、演示、排行榜和大模型等组成的精选列表,主要面向基础大模型评测,旨在探求生成式AI的技术边界.
- Host: GitHub
- URL: https://github.com/onejune2018/awesome-llm-eval
- Owner: onejune2018
- License: mit
- Created: 2023-04-26T10:10:06.000Z (almost 2 years ago)
- Default Branch: main
- Last Pushed: 2024-05-23T02:30:25.000Z (11 months ago)
- Last Synced: 2024-05-23T07:36:58.409Z (11 months ago)
- Topics: awsome-list, awsome-lists, benchmark, bert, chatglm, chatgpt, dataset, evaluation, gpt3, large-language-model, leaderboard, llama, llm, llm-evaluation, machine-learning, nlp, openai, qwen, rag
- Homepage:
- Size: 12.6 MB
- Stars: 294
- Watchers: 8
- Forks: 31
- Open Issues: 0
-
Metadata Files:
- Readme: README.md
- License: LICENSE-DATA.txt
Awesome Lists containing this project
- awesome-ChatGPT-repositories - Awesome-LLM-Eval - Awesome-LLM-Eval: a curated list of tools, demos, papers, docs for Evaluation on Large Language Models like ChatGPT, LLaMA, GLM (Awesome-lists)
- ultimate-awesome - awesome-llm-eval - Awesome-LLM-Eval: a curated list of tools, datasets/benchmark, demos, leaderboard, papers, docs and models, mainly for Evaluation on LLMs. 一个由工具、基准/数据、演示、排行榜和大模型等组成的精选列表,主要面向基础大模型评测,旨在探求生成式AI的技术边界. (Other Lists / Julia Lists)
README
[English](README_EN.md) | [中文](README_CN.md)
Awesome-LLM-Eval: a curated list of tools, datasets/benchmark, demos, learderboard, papers, docs and models, mainly for Evaluation on Large Language Models and exploring the boundaries and limits of Generative AI.
Awesome-LLM-Eval: a curated list of tools, datasets/benchmark, demos, leaderboard, papers, docs and models, mainly for Evaluation on LLMs. 一个由工具、基准/数据、演示、排行榜和大模型等组成的精选列表,主要面向基础大模型评测,旨在探求生成式AI的技术边界.
## Table of Contents- [News](#News)
- [Tools](#Tools)
- [Datasets / Benchmark](#Datasets-or-Benchmark)
- [通用](#通用)
- [垂直领域](#垂直领域)
- [RAG检索增强生成评估](#RAG检索增强生成评估)
- [Agent能力](#Agent能力)
- [代码能力](#代码能力)
- [多模态/跨模态](#多模态-跨模态)
- [长上下文](#长上下文)
- [推理速度](#推理速度)
- [量化压缩](#量化压缩)
- [Demos](#Demos)
- [Leaderboards](#Leaderboards)
- [Papers](#papers)
- [LLM-List](#LLM-List)
- [Pre-trained LLM](#Pre-trained-LLM)
- [Instruction finetuned LLM](#Instruction-finetuned-LLM)
- [Aligned LLM](#Aligned-LLM)
- [Open LLM](#Open-LLM)
- [Popular LLM](#Popular-LLM)
- [LLMOps](#LLMOps)
- [Frameworks for Training](#Frameworks-for-Training)
- [Courses](#Courses)
- [Others](#Others)
- [Other-Awesome-Lists](#Other-Awesome-Lists)
- [Licenses](#Licenses)
- [Citation](#引用)

## News- [2024/04/26] We add [推理速度](#推理速度) section.
- [2024/02/26] We add [Coding Evaluation](#代码能力) section.
- [2024/02/08] We add [lighteval](https://github.com/huggingface/lighteval) tool from Huggingface.
- [2024/01/15] We add [CRUXEval](https://arxiv.org/abs/2401.03065), [DebugBench](https://github.com/thunlp/DebugBench), [OpenFinData](https://opencompass.org.cn) and [LAiW](https://github.com/Dai-shen/LAiW).
- [2023/12/20] We add [RAG Evaluation](#RAG检索增强生成评估) section.
- [2023/11/15] We add [Instruction_Following_Eval](https://github.com/google-research/google-research/tree/master/instruction_following_eval) and [LLMBar](https://github.com/princeton-nlp/LLMBar) for the evaluation of Instruction Following ability of LLMs.
- [2023/10/20] We add [SuperCLUE-Agent](https://github.com/CLUEbenchmark/SuperCLUE-Agent) for LLM agent evaluation.
- [2023/09/25] We add [ColossalEval](https://github.com/hpcaitech/ColossalAI/tree/main/applications/ColossalEval) from Colossal-AI.- [2023/09/22] We add [Leaderboards](#Leaderboards) chapter.
- [2023/09/20] We add [DeepEval](https://github.com/mr-gpt/deepeval), [FinEval](https://github.com/SUFE-AIFLM-Lab/FinEval) and [SuperCLUE-Safety](https://github.com/CLUEbenchmark/SuperCLUE-Safety) from CLUEbenchmark.
- [2023/09/18] We add [OpenCompass](https://github.com/InternLM/opencompass/tree/main) from Shanghai AI Lab.
- [2023/08/03] We add new Chinese LLMs: [Baichuan](https://github.com/baichuan-inc/Baichuan-13B) and [Qwen](https://github.com/QwenLM/Qwen-7B).
- [2023/06/28] We add [AlpacaEval](https://github.com/tatsu-lab/alpaca_eval) and multiple tools.
- [2023/04/26] We released the V0.1 Eval list with multiple benchmarks, etc.
## Tools| 名称 | 机构 | 网址 | 简介 |
| :--: | :--: | :--: | :--: |
| prometheus-eval | prometheus-eval | [prometheus-eval](https://github.com/prometheus-eval/prometheus-eval) | PROMETHEUS开放第二版比其前身更强大的评估专用的语言模型,它能密切模仿人类和 GPT-4 的判断。此外,它能够处理直接评估和成对排序两种格式,并配合使用者定义的评估标准。在四个直接评估基准和四个成对排序基准上,PROMETHEUS 2 在所有测试的开源评估语言模型中,与人类和专有语言模型评判者取得最高的相关性和一致性 (2024-05-04) |
| athina-evals | athina-ai |[athina-ai](https://github.com/athina-ai/athina-evals) | athina-ai是一个开源库,提供即插即用的预设评估(preset evals)/模块化、可扩展的框架来编写和运行评估,帮助工程师通过评估驱动的开发来系统性地提高他们的大型语言模型的可靠性和性能,athina-ai提供了一个系统,用于评估驱动的开发,克服了传统工作流程的限制,允许快速实验和具有一致指标的可定制评估器 |
| LeaderboardFinder | Huggingface | [LeaderboardFinder](https://huggingface.co/spaces/leaderboards/LeaderboardFinder) | LeaderboardFinder帮你找到适合特定场景的大模型排行榜,排行榜的排行榜 (2024-04-02) |
| LightEval | Huggingface | [lighteval](https://github.com/huggingface/lighteval) | LightEval 是由 Hugging Face 开发的一个轻量级框架,专门用于大型语言模型(LLM)的评估。这个框架是 Hugging Face 内部用于评估最近发布的 LLM 数据处理库 datatrove 和 LLM 训练库 nanotron 的工具。现在,Hugging Face 将其开源,以便社区可以共同使用和改进。LightEval 的一些主要特点:(1) 轻量级:LightEval 设计为一个轻量级工具,易于使用和集成。(2) 评估套件:它提供了一个评估套件,支持多种任务和模型的评估。(3) 兼容性:LightEval 支持在 CPU 或 GPU 上评估模型,并且可以与 Hugging Face 的加速库(Accelerate)和 Nanotron 等框架一起使用。(4) 分布式评估:它支持在分布式环境中评估模型,这对于处理大型模型尤其有用。(5) Open LLM Leaderboard:LightEval 可以用来在 Open LLM Leaderboard 的所有基准测试上评估模型。(6) 自定义:用户可以添加新的度量标准和任务,以适应特定的评估需求。 (2024-02-08) |
| LLM Comparator | Google | [LLM Comparator](https://arxiv.org/html/2402.10524v1) | 一个用于比较和评估大型语言模型(LLM)的可视化分析工具。相较于传统的基于人工评分的方法,该工具提供了一种可扩展的自动化方面对比评估方法,旨在解决规模化评估和解释性挑战。利用另一个LLM作为评判,工具可以展示模型间质量对比,并给出理由。LLM Comparator通过交互式表格和汇总可视化,帮助用户理解模型在特定情境下表现好坏的原因,以及两种模型响应的定性差异。本文通过与Google的研究员和工程师紧密合作开发此工具,并通过观察研究评估其有效性。该工具在Google内部广泛使用,三个月内吸引了400多名用户,评估了超过1000个实验 (2024-02-16) |
| Arthur Bench | Arthur-AI |[Arthur Bench](https://github.com/arthur-ai/bench)| Arthur Bench 是一个开源的评估工具,专门设计来比较和分析大型语言模型(LLM)的性能。它支持多种评估任务,包括问答、摘要、翻译和代码生成等,能够为LLM在这些任务上的表现提供详细的报告。Arthur Bench的一些关键特性和优势:(1)模型比较:Arthur Bench 能够比较不同供应商、不同版本以及不同训练数据集的LLM模型性能。(2)提示和超参数评估:它可以评估不同的提示对LLM性能的影响,以及测试不同超参数设置对模型行为的控制。(3)任务定义与模型选择:用户可以定义具体的评估任务,并从支持的多种LLM模型中选择评估对象。(4)参数配置:Arthur Bench 允许用户调整提示和超参数,以精细化控制LLM的行为。(5)自动化评估流程:提供自动化的评估流程,简化了评估任务的执行。(6)使用场景:Arthur Bench 适用于模型选择和验证、预算和隐私优化,以及将学术基准转化为现实世界表现的评估。(7)特性分析:它具备全套评分指标,支持本地和云版本,且完全开源,鼓励社区协作和项目发展 (2023-10-06) |
| llm-benchmarker-suite | FormulaMonks | [llm-benchmarker-suite](https://github.com/FormulaMonks/llm-benchmarker-suite) | This open-source effort aims to address fragmentation and ambiguity in LLM benchmarking. The suite provides a structured methodology, a collection of diverse benchmarks, and toolkits to streamline assessing LLM performance. By offering a common platform, this project seeks to promote collaboration, transparency, and quality research in NLP. (2023-07-06) |
| autoevals | braintrust | [autoevals](https://github.com/braintrustdata/autoevals) | AutoEvals是一个AI模型输出评估工具,利用最佳实践快速轻松评估AI模型输出,集成多种自动评估方法,支持定制评估提示和自定义评分器,简化模型输出的评估过程. Autoevals uses model-graded evaluation for a variety of subjective tasks including fact checking, safety, and more. Many of these evaluations are adapted from OpenAI's excellent evals project but are implemented so you can flexibly run them on individual examples, tweak the prompts, and debug their outputs. |
| EVAL | OPENAI | [EVAL](https://github.com/openai/evals) | EVAL是OpenAI开发的一个用于评估大型语言模型(LLM)的工具,可以测试模型在不同任务和数据集上的性能和泛化能力. |
| lm-evaluation-harness | EleutherAI | [lm-evaluation-harness](https://github.com/EleutherAI/lm-evaluation-harness) | lm-evaluation-harness是EleutherAI开发的一个用于评估大型语言模型(LLM)的工具,可以测试模型在不同任务和数据集上的性能和泛化能力. |
| lm-evaluation | AI21Labs | [lm-evaluation](https://github.com/AI21Labs/lm-evaluation) | The evaluations and reproducing the results from the Jurassic-1 Technical [Paper](https://www.ai21.com/blog/announcing-ai21-studio-and-jurassic-1), with current support for running the tasks through both the AI21 Studio API and OpenAI's GPT3 API. |
| OpenCompass | Shanghai AI Lab | [OpenCompass](https://github.com/InternLM/opencompass/tree/main) | OpenCompass 是面向大模型评测的一站式平台。其主要特点如下:开源可复现:提供公平、公开、可复现的大模型评测方案;全面的能力维度:五大维度设计,提供 50+ 个数据集约 30 万题的的模型评测方案,全面评估模型能力;丰富的模型支持:已支持 20+ HuggingFace 及 API 模型;分布式高效评测:一行命令实现任务分割和分布式评测,数小时即可完成千亿模型全量评测;多样化评测范式:支持零样本、小样本及思维链评测,结合标准型或对话型提示词模板,轻松激发各种模型最大性能 |
| Large language model evaluation and workflow framework from Phase AI | wgryc | [phasellm](https://github.com/wgryc/phasellm) | phasellm是Phase AI提供的一个用于评估和管理LLM的框架,可以帮助用户选择合适的模型、数据集和指标,以及可视化和分析结果. |
| Evaluation benchmark for LLM | FreedomIntelligence | [LLMZoo](https://github.com/FreedomIntelligence/LLMZoo) | LLMZoo是FreedomIntelligence开发的一个用于评估LLM的基准,包含了多个领域和任务的数据集和指标,以及一些预训练的模型和结果. |
| Holistic Evaluation of Language Models (HELM) | Stanford | [HELM](https://github.com/stanford-crfm/helm) | HELM是Stanford研究团队提出的一个用于全面评估LLM的方法,考虑了模型的语言能力、知识、推理、公平性和安全性等多个方面. |
| A lightweight evaluation tool for question-answering | Langchain | [auto-evaluator](https://github.com/rlancemartin/auto-evaluator) | auto-evaluator是Langchain开发的一个用于评估问答系统的轻量级工具,可以自动生成问题和答案,并计算模型的准确率、召回率和F1分数等指标. |
| PandaLM | WeOpenML | [PandaLM](https://github.com/WeOpenML/PandaLM) | ReProducible and Automated Language Model Assessment | PandaLM是WeOpenML开发的一个用于自动化和可复现地评估LLM的工具,可以根据用户的需求和偏好,选择合适的数据集、指标和模型,并生成报告和图表. |
| FlagEval | Tsinghua University | [FlagEval](https://github.com/FlagOpen/FlagEval) | FlagEval是清华大学开发的一个用于评估LLM的平台,可以提供多种任务和数据集,以及在线测试、排行榜和分析等功能 |
| AlpacaEval | tatsu-lab | [alpaca_eval](https://github.com/tatsu-lab/alpaca_eval) | AlpacaEval是tatsu-lab开发的一个用于评估LLM的工具,可以使用多种语言、领域和任务进行测试,并提供可解释性、鲁棒性和可信度等指标. |
|Prompt flow | Microsoft | [promptflow](github.com/microsoft/promptflow) | 一套开发工具,旨在简化基于 LLM 的AI应用的端到端开发周期,从构思、原型设计、测试、评估到生产部署和监控。它使提示工程变得更加容易,使您能构建具有产品级质量的 LLM 应用. |
| DeepEval | mr-gpt | [DeepEval](github.com/confident-ai/deepeval) | DeepEval:提供一种 Pythonic 方式在 LLM 管线上运行离线评估,以便轻松投入生产. DeepEval is a simple-to-use, open-source LLM evaluation framework. It is similar to Pytest but specialized for unit testing LLM outputs. DeepEval incorporates the latest research to evaluate LLM outputs based on metrics such as G-Eval, hallucination, answer relevancy, RAGAS, etc., which uses LLMs and various other NLP models that runs locally on your machine for evaluation. |
| CONNER | Tencent AI Lab | [CONNER](https://github.com/ChanLiang/CONNER) | CONNER:一个综合性的大模型知识评估框架,旨在从六个重要角度系统地、自动地评估生成的信息——事实性、相关性、连贯性、信息性、有用性和有效性。 |
## Datasets-or-Benchmark
### 通用| 名称 | 机构 | 网址 | 简介 |
| :--: | :--: | :--: | :-- |
| MMLU-Pro | TIGER-AI-Lab | [MMLU-Pro](https://github.com/TIGER-AI-Lab/MMLU-Pro) | MMLU-Pro 是 MMLU 数据集的改进版本。MMLU 一直是多选知识数据集的参考。然而,最近的研究表明它既包含噪音(一些问题无法回答),又太容易(通过模型能力的进化和污染的增加)。MMLU-Pro 向模型提供十个选择而不是四个,要求在更多问题上进行推理,并经过专家审查以减少噪音量。它比原版质量更高且更难. MMLU-Pro减少了提示变化对模型性能的影响,这是其前身MMLU常见的问题。研究发现,在这个新基准上使用“Chain of Thought”推理的模型表现更好,这表明MMLU-Pro更适合评估人工智能的微妙推理能力. (2024-05-20) |
| TrustLLM Benchmark | TrustLLM | [TrustLLM](https://trustllmbenchmark.github.io/TrustLLM-Website/) | TrustLLM是一个针对大语言模型可信赖的评估基准,该基准测试涵盖了6个可信赖维度,包含了30+的数据集,以全面评估LLMs的功能能力,范围从简单分类到复杂的生成任务。每个数据集都提出了独特的挑战,并在可信度的多个维度上对16个主流的大语言模型(包括商业模型和开源模型)进行了基准测试。|
| DyVal | Microsoft | [DyVal](https://github.com/microsoft/promptbench) | 人们对于其庞大的训练语料库中潜在的数据污染问题表示担忧。此外,当前基准测试的静态性质和固定复杂度可能无法充分衡量LLMs不断进步的能力。DyVal,这是一种用于动态评估LLMs的通用且灵活的协议。基于图的DyVal,利用有向无环图的结构优势动态生成具有可控复杂度的评估样本。DyVal在包括数学、逻辑推理和算法问题在内的推理任务上生成了具有挑战性的评估集。评估了从Flan-T5-large到GPT-3.5-Turbo和GPT-4的各种LLMs。实验表明,LLMs在DyVal生成的不同复杂度的评估样本中表现更差,突显了动态评估的重要性。作者还分析了不同提示方法的失败案例和结果。此外,DyVal生成的样本不仅是评估集,而且有助于微调,以提高LLMs在现有基准测试上的性能 (2024-04-20) |
| RewardBench | AIAI | [RewardBench](https://github.com/allenai/reward-bench) | 语言模型奖励模型评估基准RewardBench,评估各类模型的优劣势,发现现有模型在推理和遵循指令方面仍存在明显不足,包含[Leaderboard](https://hf.co/spaces/allenai/reward-bench),[Code](https://github.com/allenai/reward-bench) 和 [Dataset](https://hf.co/datasets/allenai/reward-bench) (2024-03-20)|
| LV-Eval | Infinigence-AI | [LVEval](https://github.com/infinigence/LVEval) | LV-Eval是一个具备5个长度等级(16k、32k、64k、128k和256k)、最大文本测试长度达到256k的长文本评测基准。LV-Eval的平均文本长度达到102,380字,最小/最大文本长度为11,896/387,406字。LV-Eval主要有两类评测任务——单跳QA和多跳QA,共包含11个涵盖中英文的评测数据子集。LV-Eval设计时引入3个关键技术:干扰事实插入(Confusiong Facts Insertion,CFI)提高挑战性,关键词和短语替换(Keyword and Phrase Replacement,KPR)减少信息泄漏,以及基于关键词召回的评测指标(Answer Keywords,AK,指代结合答案关键词和字词黑名单的评价指标)提高评测数值客观性 (2024-02-06) |
| LLM-Uncertainty-Bench | Tencent | [LLM-Uncertainty-Bench](https://github.com/smartyfh/LLM-Uncertainty-Bench) | 引入了一种新的LLMs基准测试方法,该方法整合了不确定性量化。基于九个LLMs针对五个代表性的自然语言处理任务试验,发现I) 准确度更高的LLMs可能表现出较低的确定性;II) 规模更大的LLMs可能比规模较小的模型表现出更大的不确定性;III) 指令微调倾向于增加LLMs的不确定性。这些结果强调了在评估LLMs时纳入不确定性的重要性 (2024-01-22)|
| Psychometrics Eval | 微软亚洲研究院 | [Psychometrics Eval](https://arxiv.org/abs/2310.16379) | 微软亚洲研究院提出了一种基于心理测量学的通用型人工智能评估方法,旨在解决传统评估方法在预测力、信息量和测试工具质量上的局限。该方法借鉴心理测量学的理论,通过识别AI的关键心理构念,设计针对性测验并应用项目反应理论进行精准评分,同时引入信度和效度概念确保评估的可靠性和准确性。这一框架扩展了心理测量学方法,适用于评估AI在处理未知复杂任务时的表现,但同时也面临着如何区分AI的“个体”与“总体”、处理prompts敏感性以及评估人类与AI构念差异等开放性问题 (2023-10-19) |
| CommonGen-Eval | allenai |[CommonGen-Eval](https://github.com/allenai/CommonGen-Eval) | 使用CommonGen-lite数据集对LLM进行评估的研究,使用了GPT-4模型进行评估,比较了不同模型的性能,并列出了排行榜上的模型结果 (2024-01-04) |
| felm | hkust | [felm](https://github.com/hkust-nlp/felm)| | FELM is a meta benchmark to evaluate factuality evaluation for large language models. The benchmark comprises 847 questions that span five distinct domains: world knowledge, science/technology, writing/recommendation, reasoning, and math. We gather prompts corresponding to each domain by various sources including standard datasets like truthfulQA, online platforms like Github repositories, ChatGPT generation or drafted by authors. We then obtain responses from ChatGPT for these prompts. For each response, we employ fine-grained annotation at the segment level, which includes reference links, identified error types, and the reasons behind these errors as provided by our annotators. (2023-10-03) |
| just-eval | AI2 Mosaic |[just-eval](https://github.com/Re-Align/just-eval)| 基于GPT的评估工具,用于对LLM进行多方面、可解释的评估,可以评估帮助性、清晰度、真实性、深度和参与度等方面 (2023-12-05) |
| EQ-Bench | EQ-Bench | [EQ-Bench](https://github.com/EQ-bench/EQ-Bench) | 用于评估语言模型情感智能的基准测试,包含171个问题(相比v1的60个问题)和一种新的评分系统,能更好地区分模型之间的性能差异 (2023-12-20) |
| CRUXEval | MIT CSAIL | [CRUXEval](https://arxiv.org/abs/2401.03065) | CRUXEval是一个用于代码推理、理解和执行评估的基准,包含800个Python函数及其输入输出对,测试输入预测和输出预测任务。许多在HumanEval上得分高的模型在CRUXEval上表现不佳,突显了改进代码推理能力的需求。最佳模型GPT-4结合了思维链(CoT),在输入预测和输出预测上的pass@1分别达到了75%和81%。该基准测试暴露了开源和闭源模型之间的差距。GPT-4未能完全通过CRUXEval,提供了对其局限性和改进方向的见解 (2024-01-05)|
| MLAgentBench | snap-stanford | [MLAgentBench](https://github.com/snap-stanford/MLAgentBench) | MLAgentBench是一套端到端的机器学习(ML)研究任务,用于对AI研究代理进行基准测试,其中代理的目标是根据给定的数据集和机器学习任务描述,自主地开发或改进一个ML模型。每个任务都是一个交互式的环境,直接反映了人类研究者所看到的情况,其中代理可以读取可用的文件,在计算集群上运行多个实验,并分析结果以实现指定的研究目标。具体来说,包括了15个不同的ML工程任务,可以通过尝试不同的机器学习方法、数据处理、架构、训练过程来实现 (2023-10-05) |
| AlignBench | THUDM | [AlignBench](https://github.com/THUDM/AlignBench) | AlignBench是一个用于评估中文大语言模型对齐性能的全面、多维度的评测基准。AlignBench 构建了人类参与的数据构建流程,来保证评测数据的动态更新。AlignBench 采用多维度、规则校准的模型评价方法(LLM-as-Judge),并且结合思维链(Chain-of-Thought)生成对模型回复的多维度分析和最终的综合评分,增强了评测的高可靠性和可解释性 (2023-12-01)|
| UltraEval | OpenBMB | [UltraEval](https://github.com/OpenBMB/UltraEval) | UltraEval是一个开源的基础模型能力评测框架,提供了一套轻量级、易于使用的评测体系,支持主流大模型的性能评估。它的主要特色如下:(1)轻量易用的评测框架:具备简洁直观的设计,依赖少,易于部署,具有良好的扩展性,适用多种评测场景。()2灵活多样的评测方法:提供了统一的prompt模板和丰富的评估指标,同时支持自定义。(3)高效快速的推理部署:支持包括torch和vLLM在内的多种模型部署方案,并实现了多实例部署以加速评测过程。(4)公开透明的开源榜单:维护一个公开的、可追溯和可复现的评测榜单,由社区推动更新,确保透明度。(5)官方权威的评测数据:采用广泛认可的官方评测集,保证评测的公平性和标准化,确保结果具有可比性和复现性 (2023-11-24) |
| IFEval | google-research | [Instruction_Following_Eval](https://github.com/google-research/google-research/tree/master/instruction_following_eval) | 大型语言模型的一个核心能力是遵循自然语言指令。然而,对这种能力的评估并没有标准化:人工评估费用高、速度慢,并且缺乏客观的可重复性,而基于LLM的自动评估可能存在评估者LLM的偏见或能力限制。为了克服这些问题,google的研究者引入了用于大型语言模型的指令遵循评估(IFEval)。IFEval是一个简单易复现的评估基准,侧重于一组“可验证指令”,例如“写入超过400字”和“至少提到AI关键词3次”。IFEval确定了25种这些可验证指令,并构建了约500个提示,每个提示包含一个或多个可验证指令 (2023-11-15) |
| LLMBar | princeton-nlp | [LLMBar](https://github.com/princeton-nlp/LLMBar) | 一个名为LLMBar的具有挑战性的元评估基准,旨在测试LLM评估器在识别遵循指令的输出方面的能力。LLMBar包含419个实例,每个实例包含一条指令和两个输出:一个忠实并正确地遵循指令,另一个则偏离指令。每个实例还有一个金标签,指示哪个输出在客观上更好 (2023-10-29) |
| HalluQA | Fudan, Shanghai AI Lab | [HalluQA](https://github.com/xiami2019/HalluQA/) | 幻觉是文本生成领域的一个经典问题,HalluQA是一个中文大模型幻觉评测基准,收集了450条数据,其中misleading部分175条,misleading-hard部分69条,knowledge部分206条,每个问题平均有2.8个正确答案和错误答案标注。为了提高HalluQA的可用性,作者设计了一个使用GPT-4担任评估者的评测方法。具体来说,把幻觉的标准以及作为参考的正确答案以指令的形式输入给GPT-4,让GPT-4判断模型的回复有没有出现幻觉 (2023-11-08) |
| FMTI | stanford | [FMTI](https://crfm.stanford.edu/fmti/) | 提出基础模型透明度指数(The Foundation Model Transparency Index),来评估不同开发者在模型训练和部署方面的透明度。该指数包含100个指标,评估范围广泛,包括数据、计算资源、劳动力等多个方面。对10家公司旗舰模型的评估显示,平均透明度指数仅为37/100,存在很大提升空间 (2023-10-18) |
| LLMBar | princeton-nlp | [LLMBar](https://github.com/princeton-nlp/LLMBar) | LLMBar引入了一个具有挑战性的meta评估基准LLMBAR,旨在测试LLM评估者识别指令跟随输出的能力。还提出了一套新颖的提示策略,进一步缩小了LLM和人类评估者之间的差距 (2023-10-11) |
| BAMBOO | RUCAIBox | [BAMBOO](https://github.com/RUCAIBox/BAMBOO) | BAMBOO基准测试是一个用于分析LLMs的长文本建模能力的综合基准测试。在BAMBOO基准测试中,有来自5个任务的10个数据集,即,问答、幻觉检测、语言建模、代码补全和文本排序。我们的基准测试是根据以下原则构建的:综合能力评估、避免数据污染、准确的自动评估、不同的长度等级 (2023-10-11) |
| TRACE | Fudan University | [TRACE](https://arxiv.org/abs/2310.06762) | TRACE是一个专为评估大型语言模型(LLMs)的持续学习能力而设计的新型基准测试。TRACE包含了8个不同的数据集,涵盖了包括领域特定任务、多语言能力、代码生成和数学推理等在内的挑战性任务 (2023-10-05) |
| ColossalEval | Colossal-AI | [ColossalEval](https://github.com/hpcaitech/ColossalAI/tree/main/applications/ColossalEval) | ColossalEval 是一个项目,提供一个统一评估流程的项目,用于在不同的公共数据集或自己的数据集上评估语言模型,使用传统指标以及来自 GPT(生成式预训练模型)的帮助 |
| LLMEval²-WideDeep | AlibabaResearch | [LLMEval²](https://github.com/AlibabaResearch/DAMO-ConvAI/tree/main/WideDeep) | 构建了最大、最多样化的英语评估基准LLMEval²,供LLM评估者使用,包括15个任务、8个能力和2,553个样本。实验结果表明,一个更宽的网络(涉及许多审阅者)和2层(一轮讨论)的性能最佳,将Kappa相关系数从0.28提高到0.34。我们还利用WideDeep来辅助评估中文LLM,这加速了评估时间4.6倍,节省了60%的成本 |
| Aviary | ray-project | [Aviary](github.com/ray-project/aviary) |允许在一个地方与各种大型语言模型(LLM)进行交互。可以直接比较不同模型的输出,按质量进行排名,获得成本和延迟估计等功能。特别支持在Hugging Face上托管的Transformer模型,并在许多情况下还支持DeepSpeed推理加速 (202306) |
| Do-Not-Answer | Libr-AI | [Do-Not-Answer](https://github.com/Libr-AI/do-not-answer)| "Do not answer" 是一个开源数据集,旨在以低成本评估LLM(大型语言模型)的安全机制。该数据集经过策划和筛选,仅包含那些负责任的语言模型不回答的提示。除了人工标注外,“Do not answer” 还实施了基于模型的评估,其中一个经过6亿次微调的类似BERT的评估器获得了与人类和GPT-4相媲美的结果 |
| LucyEval | 甲骨文 | [LucyEval](http://lucyeval.besteasy.com/) | 中文大语言模型成熟度评测——LucyEval,能够通过对模型各方面能力的客观测试,找到模型的不足,帮助设计者和工程师更加精准地调整、训练模型,助力大模型不断迈向更智能的未来 |
| Zhujiu | Institute of Automation, CAS | [Zhujiu](http://www.zhujiu-benchmark.com) | 多维能力覆盖,涵盖了7个能力维度和51个任务;多方面的评估方法协作,综合使用3种不同但互补的评估方法;全面的中文基准测试,同时提供英文评估能力 |
| ChatEval | THU-NLP | [ChatEval](https://github.com/thunlp/ChatEval) |ChatEval旨在简化人类对生成的文本进行评估的过程。当给定不同的文本片段时,ChatEval中的角色(由法学硕士扮演)可以自主地讨论细微差别和差异,利用他们指定的角色,随后提供他们的判断|
| FlagEval | 智源/清华 | [FlagEval](https://flageval.baai.ac.cn/#/home) | 智源出品,结合主观和客观评分,提供了LLM的评分榜单|
| InfoQ大模型综合能力评估 | InfoQ | [InfoQ评测](https://mp.weixin.qq.com/s?__biz=MjM5MDE0Mjc4MA==&mid=2651170429&idx=1&sn=b98af3bd14c9f97f1aa07f0f839bb3ec&scene=21#wechat_redirect) | 面向中文,排名为 ChatGPT > 文心一言 > Claude > 星火|
| Chain-of-thought评估 | Yao Fu | [COT评估](https://github.com/FranxYao/chain-of-thought-hub) | 包括 GSM8k、MATH 等复杂问题排名|
| Z-Bench中文真格基金评测 | 真格基金 | [ Z-Bench](https://github.com/zhenbench/z-bench) | 国产中文模型的编程可用性相对较低,模型水平差异不大,两个版本的 ChatGLM 有明显提升|
| CMU开源聊天机器人评测应用 | CMU | [zeno-build](https://github.com/zeno-ml/zeno-build) | 在对话场景中进行训练可能很重要,排名为 ChatGPT > Vicuna > 其他|
| lmsys-arena | Berkley | [lmsys排名榜](https://lmsys.org/blog/2023-05-03-arena/) | 使用 Elo 评分机制,排名为 GPT4 > Claude > GPT3.5 > Vicuna > 其他|
| Huggingface开源LLM排行榜 | huggingface | [HF开源LLM排行榜](https://huggingface.co/spaces/HuggingFaceH4/open_llm_leaderboard) | 仅评估开源模型,在 Eleuther AI 的四个评估集上排名,Falcon 夺冠,vicuna 亦夺冠|
| AlpacaEval | tatsu-lab | [AlpacaEval](https://tatsu-lab.github.io/alpaca_eval/) | 开源模型领先者 vicuna、openchat 和 wizardlm 的基于LLM的自动评估|
| chinese-llm-benchmark | jeinlee1991 | [llm-benchmark](https://github.com/jeinlee1991/chinese-llm-benchmark) | 中文大模型能力评测榜单:覆盖百度文心一言、chatgpt、阿里通义千问、讯飞星火、belle / chatglm6b 等开源大模型,多维度能力评测。不仅提供能力评分排行榜,也提供所有模型的原始输出结果 |
| Open LLM Leaderboard | HuggingFace | [Leaderboard](https://huggingface.co/spaces/HuggingFaceH4/open_llm_leaderboard) | 由HuggingFace组织的一个LLM评测榜单,目前已评估了较多主流的开源LLM模型。评估主要包括AI2 Reasoning Challenge, HellaSwag, MMLU, TruthfulQA四个数据集上的表现,主要以英文为主 |
| SQUAD | Stanford NLP Group | [SQUAD](https://rajpurkar.github.io/SQuAD-explorer/) | 评估模型在阅读理解任务上的表现 |
| MultiNLI | New York University, DeepMind, Facebook AI Research, Allen Institute for AI, Google AI Language | [MultiNLI](https://cims.nyu.edu/~sbowman/multinli/) | 评估模型在不同文体之间理解句子关系的能力 |
| LogiQA | Tsinghua University and Microsoft Research Asia | [LogiQA](https://github.com/lgw863/LogiQA-dataset) | 评估模型的逻辑推理能力 |
| HellaSwag | University of Washington and Allen Institute for AI | [HellaSwag](https://rowanzellers.com/hellaswag/) | 评估模型的推理能力 |
| LAMBADA | University of Trento and Fondazione Bruno Kessler | [LAMBADA](https://zenodo.org/record/2630551#.ZFUKS-zML0p) | 评估模型使用预测段落最后一个词的方式来衡量长期理解能力 |
| CoQA | Stanford NLP Group | [CoQA](https://stanfordnlp.github.io/coqa/) | 评估模型在理解文本段落并回答一系列相互关联的问题,这些问题出现在对话中的能力 |
| ParlAI | Facebook AI Research | [ParlAI](https://github.com/facebookresearch/ParlAI) | 评估模型在准确性、F1分数、困惑度(模型预测序列中下一个词的能力)、人类评价(相关性、流畅度和连贯性)、速度和资源利用率、鲁棒性(模型在不同条件下的表现,如噪声输入、对抗攻击或数据质量变化)、泛化能力等方面的表现 |
| LIT (Language Interpretability Tool) | Google | [LIT](https://pair-code.github.io/lit/) | 提供一个平台,可以根据用户定义的指标进行评估,分析模型的优势、弱点和潜在偏差 |
| Adversarial NLI (ANLI) | Facebook AI Research, New York University, Johns Hopkins University, University of Maryland, Allen Institute for AI | [Adversarial NLI (ANLI)](https://github.com/facebookresearch/anli) | 评估模型在对抗样本下的鲁棒性、泛化能力、推理解释能力和一致性,以及资源使用效率(内存使用、推理时间和训练时间)|
| AlpacaEval | tatsu-lab | [AlpacaEval](https://github.com/tatsu-lab/alpaca_eval) | AlpacaEval是一种基于LLM的快速、廉价且可靠的自动评估方法。它基于AlpacaFarm评估集,测试模型遵循一般用户指令的能力。然后,这些响应与提供的GPT-4、Claude或ChatGPT基于自动注释者的参考Davinci003响应进行比较,从而产生上述的胜率 |
| OpenAI Evals | OpenAI | [OpenAI Evals](https://github.com/openai/evals) | 评估生成文本的准确性、多样性、一致性、鲁棒性、迁移性、效率和公平性 |
| EleutherAI LM Eval | EleutherAI | [EleutherAI LM Eval](https://github.com/EleutherAI/lm-evaluation-harness) | 评估模型在少量样本下的表现和在多种任务上的微调效果 |
| OpenAI Moderation API | OpenAI | [OpenAI Moderation API](https://platform.openai.com/docs/api-reference/moderations) | 过滤有害或不安全的内容 |
| GLUE Benchmark | NYU, University of Washington, DeepMind, Facebook AI Research, Allen Institute for AI, Google AI Language | [GLUE Benchmark](https://gluebenchmark.com/) | 评估模型在语法、改写、文本相似度、推理、文本蕴含、代词指代等任务上的表现 |
| MT-bench | UC Berkeley, UCSD, CMU, Stanford, MBZUAI | [MT-bench](https://arxiv.org/abs/2306.05685) | MT-bench旨在测试多轮对话和遵循指令的能力,包含80个高质量的多轮问题,涵盖常见用例并侧重于具有挑战性的问题,以区分不同的模型。它包括8个常见类别的用户提示,以指导其构建:写作、角色扮演、提取、推理、数学、编程等 |
| XieZhi | Fudan Univesity | [XieZhi](https://github.com/MikeGu721/XiezhiBenchmark) | A comprehensive evaluation suite for Language Models (LMs). It consists of 249587 multi-choice questions spanning 516 diverse disciplines and four difficulty levels. 新的领域知识综合评估基准测试:Xiezhi。对于多选题,Xiezhi涵盖了516种不同学科中的220,000个独特问题,其中涵盖了13个学科。作者还提出了Xiezhi-Specialty和Xiezhi-Interdiscipline,每个都含有15k个问题。使用Xiezhi基准测试评估了47种先进的LLMs的性能|
| C_Eval | 上交、清华以及爱丁堡大学 | [C_Eval](https://cevalbenchmark.com/index.html#home) | 上交、清华以及爱丁堡大学合作产出的一个评测集,包含52个学科来评估大模型高级知识和推理能力,其评估了包含 GPT-4、ChatGPT、Claude、LLaMA、Moss 等多个模型的性能。|
| AGIEval | 微软研究院 | [AGIEval](https://www.microsoft.com/en-us/research/project/agi-evaluation/) | 由微软研究院发起,旨在全面评估基础模型在人类认知和问题解决相关任务上的能力,包含了中国的高考、司法考试,以及美国的SAT、LSAT、GRE和GMAT等20个公开且严谨的官方入学和职业资格考试|
| MMCU | 甲骨易AI研究院 | [MMCU](https://github.com/Felixgithub2017/MMCU) | 甲骨易AI研究院提出一种衡量中文大模型处理多任务准确度的测试, 数据集的测试内容涵盖四大领域:医疗、法律、心理学和教育。题目的数量达到1万+,其中包括医疗领域2819道题,法律领域3695道题,心理学领域2001道,教育领域3331道 |
| CMMLU | MBZUAI & ShangHai JiaoTong & Microsoft | [CMMLU](https://github.com/haonan-li/CMMLU) | Measuring massive multitask language understanding in Chinese |
| MMLU | paperswithcode.com | [MMLU](https://paperswithcode.com/dataset/mmlu) | 该测评数据集涵盖 STEM、人文学科、社会科学等领域的 57 个学科。难度从初级到专业高级,既考验世界知识,又考验解决问题的能力。学科范围从数学和历史等传统领域到法律和伦理等更专业的领域。主题的粒度和广度使基准成为识别模型盲点的理想选择 |
| Gaokao | ExpressAI | [Gaokao](https://github.com/ExpressAI/AI-Gaokao) | “高考基准”旨在评估和追踪我们在达到人类智力水平方面取得的进展。它不仅可以提供对现实世界场景中实际有用的不同任务和领域的全面评估,还提供丰富的人类表现,以便大模型等可以直接与人类进行比较 |
| GAOKAO-Bench | OpenLMLab | [GAOKAO-Bench](https://github.com/OpenLMLab/GAOKAO-Bench) | GAOKAO-bench是一个以中国高考题目为数据集,测评大模型语言理解能力、逻辑推理能力的测评框架 |
| Safety Eval | 清华大学 | [Safety Eval 安全大模型评测](http://115.182.62.166:18000) | 清华收集的一个评测集,涵盖了仇恨言论、偏见歧视言论、犯罪违法、隐私、伦理道德等八大类别,包括细粒度划分的40余个二级安全类别,并依托于一套系统的安全评测框架 |
| SuperCLUE-Safety | CLUEbenchmark | [SuperCLUE-Safety](github.com/CLUEbenchmark/SuperCLUE-Safety) | 中文大模型多轮对抗安全基准 |
| SuperCLUE | CLUEbenchmark | [SuperCLUE](https://github.com/CLUEbenchmark/SuperCLUE) | 中文的一个榜单,这里从基础能力、专业能力、中文特性三个角度进行准备测试集 基础能力能力包括:语义理解、对话、逻辑推理、角色模拟、代码、生成与创作等10项能力。专业能力包括:包括了中学、大学与专业考试,涵盖了从数学、物理、地理到社会科学等50多项能力。中文特性能力:针对有中文特点的任务,包括了中文成语、诗歌、文学、字形等10项多种能力 |
| BIG-Bench-Hard | Stanford NLP | [BIG-Bench-Hard](https://github.com/suzgunmirac/BIG-Bench-Hard) | 一组包含23个具有挑战性的BIG-Bench任务,我们称之为BIG-Bench Hard(BBH)。这些任务是以前的语言模型评估未能超越平均人工评分者的任务 |
| BIG-bench | google | [BIG-bench](https://github.com/google/BIG-bench) | BIG bench由 204 项任务组成,任务主题涉及语言学、儿童发展、数学、常识推理、生物学、物理学、社会偏见、软件开发等等领域的问题 |
| JioNLP-LLM评测数据集 | jionlp | [JioNLP-LLM评测数据集](https://github.com/dongrixinyu/JioNLP/wiki/LLM评测数据集) | LLM 评测数据集主要用于评测通用 LLM 的效果评价。着眼考察 LLM 模型对人类用户的帮助效果、辅助能力,可否达到一个【智能助手】的水平。题型包括:选择题来源于中国大陆国内各种专业性考试,重点在于考察模型对客观知识的覆盖面,占比 32%;主观题来源于日常总结,主要考察用户对 LLM 常用功能的效果 |
| promptbench | microsoft | [promptbench](https://github.com/microsoft/promptbench) | PromptBench是一个强大的工具,旨在仔细研究和分析大型语言模型与各种提示的互动。它提供了一个方便的基础设施,用于模拟对模型的黑盒对抗提示攻击并评估它们的性能。这个存储库托管了必要的代码库、数据集和说明,以促进这些实验的进行 |
| KoLA | THU-KEG | [KoLA](http://103.238.162.37:31622) | Knowledge-oriented LLM Assessment benchmark(KoLA)由清华大学知识工程组(THU-KEG)托管,旨在通过进行认真的设计,考虑数据、能力分类和评估指标,来精心测试LLMs的全球知识。这个基准测试包含80个高质量的多轮问题 |
| M3Exam | DAMO | [M3Exam](https://github.com/DAMO-NLP-SG/M3Exam) | 一个多语言、多模态、多层次的用于研究大型语言模型的基准测试 |
### 垂直领域| 名称 | 机构 | 领域 | 网址 | 简介 |
| :--: | :--: | :--: | :--: | :-- |
| Seismometer | Epic | 医疗 | [seismomete](github.com/epic-open-source/seismometer) | Seismometer是一个医疗保健领域AI模型性能评估工具,提供标准化评估标准,帮助决策基于本地数据和工作流程的模型验证,支持模型性能的持续监控. seismometer is a suite of tools that allows you to evaluate AI model performance using these standardized evaluation criteria to help you make decisions based on your own local data and workflows. You can use it to validate a model’s initial performance and continue to monitor its performance over time. Although it can be used for models in any field, it was designed with a focus on validation for healthcare AI models where local validation requires cross-referencing data about patients (such as demographics, clinical interventions, and patient outcomes) and model performance. (2024-05-22) |
|Medbench| OpenMEDLab | 医疗 | [medbench](https://github.com/open-compass/opencompass/tree/main/opencompass/datasets/medbench/) | MedBench致力于打造一个科学、公平且严谨的中文医疗大模型评测体系及开放平台。我们基于医学权威标准,不断更新维护高质量的医学数据集,全方位多维度量化模型在各个医学维度的能力. MedBench, a comprehensive benchmark for the Chinese medical domain, comprising 40,041 questions sourced from authentic examination exercises and medical reports of diverse branches of medicine. In particular, this benchmark is composed of four key components: the Chinese Medical Licensing Examination, the Resident Standardization Training Examination, the Doctor In-Charge Qualification Examination, and real-world clinic cases encompassing examinations, diagnoses, and treatments. (2023-12-20)|
|Fin-Eva| 蚂蚁集团、上海财经大学 | 金融 | [Fin-Eva](https://github.com/alipay/financial_evaluation_dataset) | 蚂蚁集团、上海财经大学联合推出金融评测集Fin-Eva Version 1.0,覆盖财富管理、保险、投资研究等多个金融场景以及金融专业主题学科,总评测题数目达到1.3w+。蚂蚁数据源包括各业务领域数据、互联网公开数据,经过数据脱敏、文本聚类、语料精筛、数据改写等处理过程后,结合金融领域专家的评审构建而成。上海财经大学数据源主要基于相关领域权威性考试的各类真题和模拟题对知识大纲的要求。蚂蚁部分涵盖金融认知、金融知识、金融逻辑、内容生成以及安全合规五大类能力33个子维度共8445个测评题; 上财部分涵盖金融,经济,会计和证书等四大领域,包括4661个问题,涵盖34个不同的学科。Fin-Eva Version 1.0 全部采用单选题这类有固定答案的问题,配合相应指令让模型输出标准格式 (2023-12-20) |
| GenMedicalEval | SJTU | 医疗 |[GenMedicalEval](https://github.com/MediaBrain-SJTU/GenMedicalEval)| 1.**大规模综合性能评测**:GenMedicalEval构建了一个覆盖16大主要科室、3个医生培养阶段、6种医学临床应用场景、基于40,000+道医学考试真题和55,000+三甲医院患者病历构建的总计100,000+例医疗评测数据。这一数据集从医学基础知识、临床应用、安全规范等层面全面评估大模型在真实医疗复杂情境中的整体性能,弥补了现有评测基准未能覆盖医学实践中众多实际挑战的不足。 2.**深入细分的多维度场景评估**:GenMedicalEval融合了医师的临床笔记与医学影像资料,围绕检查、诊断、治疗等关键医疗场景,构建了一系列多样化和主题丰富的生成式评估题目,为现有问答式评测模拟真实临床环境的开放式诊疗流程提供了有力补充。 3.**创新性的开放式评估指标和自动化评估模型**:为解决开放式生成任务缺乏有效评估指标的难题,GenMedicalEval采用先进的结构化抽取和术语对齐技术,构建了一套创新的生成式评估指标体系,这一体系能够精确衡量生成答案的医学知识准确性。进一步地,基于自建知识库训练了与人工评价相关性较高的医疗自动评估模型,提供多维度医疗评分和评价理由。这一模型的特点是无数据泄露和自主可控,相较于GPT-4等其他模型,具有独特优势 (2023-12-08)|
| OpenFinData | 上海人工智能实验室 | 金融 | [OpenFinData](https://opencompass.org.cn) |首个全场景金融测评数据集OpenFinData(基于"OpenCompass"框架),由上海人工智能实验室发布,包含的六大模块和十九项金融任务维度,覆盖多层次数据类型和多样化金融场景,每一条数据均由实际金融业务场景产生 (2024-01-04)|
|LAiW| Sichuan University | 法律 |[LAiW](https://github.com/Dai-shen/LAiW)|从法学角度和可实现性上对法律 NLP的能力进行划分,将其分成了3大能力,共计13个基础任务:(1)法律 NLP 基础能力:评测法律基础任务、 NLP 基础任务和法律信息抽取的能力,包括法条推送、要素识别、命名实体识别、司法要点摘要和案件识别 5 个基础任务;(2)法律基础应用能力:评测大模型对法律领域知识的基础应用能力,包括争议焦点挖掘、类案匹配、刑事裁判预测、民事裁判预测和法律问答 5 个基础任务;(3)法律复杂应用能力:评测大模型对法律领域知识的复杂应用能力,包括司法说理生成、案情理解和法律咨询 3 个基础任务 ([2023-10-08)|
| LawBench | Nanjing University | 法律 | [LawBench](https://github.com/open-compass/lawbench) | LawBench经过精心设计,可对大语言模型的法律能力进行精确评估。 在设计测试任务时,模拟了司法认知的三个维度,并选择了20个任务来评估大模型的能力。与一些仅有多项选择题的现有基准相比,LawBench包含了更多与现实世界应用密切相关的任务类型,如法律实体识别、阅读理解、犯罪金额计算和咨询等。 LawBench认识到当前大模型的安全性策略可能会拒绝回应某些法律询问,或在理解指令方面遇到困难,从而导致缺乏回应。因此,LawBench中开发了一个单独的评估指标 "弃权率",以衡量模型拒绝提供答案或未能正确理解指令的频率。 研究者评测了51种大语言模型在LawBench上的表现,包括20种多语言模型、22种中文模型和9种法律专用大语言模型 (2023-09-28) |
| PsyEval | SJTU | 心理 | [PsyEval](https://arxiv.org/abs/2311.09189) | 在心理健康研究中,大型语言模型(LLMs)的使用越来越受到关注,尤其是其疾病检测等显著能力。研究者为心理健康领域量身定制了第一个全面基准,以系统地评估LLMs在这个领域的能力。这个基准包括六个子任务,涵盖了三个维度,以全面评估LLMs在心理健康领域的能力。为每个子任务设计了相应的简洁提示。并全面评估了八个高级LLM (2023-11-15) |
| PPTC| Microsoft, PKU | 办公 | [PPTC](https://github.com/gydpku/PPTC) | PPTC是用于测试大模型在PPT生成方面的能力的基准,包含 279 个涵盖不同主题的多回合会话和数百条涉及多模式操作的说明。研究团队还提出了PPTX-Match评估系统,该系统根据预测文件而不是标签API序列来评估大语言模型是否完成指令,因此它支持各种LLM生成的API序列目前PPT生成存在三个方面的不足:多轮会话中的错误累积、长PPT模板处理和多模态感知问题 (2023-11-04) |
| LLMRec | Alibaba | 推荐 | [LLMRec](https://github.com/williamliujl/LLMRec)| 对热门LLMs((如ChatGPT、LLaMA、ChatGLM等),在5种推荐相关任务上进行基准测试,这些任务包括:评分预测、顺序推荐、直接推荐、解释生成和评论摘要。此外,还研究了监督微调的有效性,以提高LLMs的指令遵从能力 (2023-10-08)|
| LAiW | Dai-shen | 法律 | [LAiW](https://github.com/Dai-shen/LAiW) | 针对法律大型语言模型的快速发展,提出了第一个基于法律能力的中文法律大型语言模型基准。将法律能力划分为基本的法律自然语言处理能力、基本的法律应用能力和复杂的法律应用能力三个层次。完成了第一阶段的评估,主要集中在基本法律自然语言处理能力的能力评估。评估结果显示,尽管一些法律大型语言模型的性能优于其基础模型,但与ChatGPT相比仍存在差距 (2023-10-25)|
| OpsEval | Tsinghua University | AIOps | [OpsEval](https://arxiv.org/abs/2310.07637) | OpsEval是一个针对大型语言模型的综合任务导向的AIOps基准测试,评估了LLMs在三个关键场景(有线网络操作、5G通信操作和数据库操作)中的熟练程度,这些场景涉及不同的能力水平(知识回忆、分析思维和实际应用)。该基准包含了7,200个问题,分为多项选择和问答(QA)两种格式,支持英文和中文 (2023-10-02) |
| SWE-bench | princeton-nlp | 软件 | [SWE-bench](https://github.com/princeton-nlp/SWE-bench) | SWE-bench 是一个用于评估大型语言模型在从 GitHub 收集的实际软件问题上的基准测试。给定一个代码库和一个问题,语言模型的任务是生成一个能够解决所描述问题的补丁 |
| BLURB | Mindrank AI | 医疗 | [BLURB](https://microsoft.github.io/BLURB/index.html) | BLURB包括一个基于PubMed的生物医学自然语言处理应用的全面基准测试,以及一个用于跟踪社区进展的排行榜。BLURB包含六种多样化任务、十三个公开可用数据集。为了避免过于强调具有许多可用数据集的任务(例如命名实体识别NER),BLURB以所有任务的宏平均作为主要分数进行报告。BLURB的排行榜不依赖于模型,任何能够使用相同的训练和开发数据生成测试预测的系统都可以参与。BLURB的主要目标是降低在生物医学自然语言处理中的参与门槛,并帮助加速这个对社会和人类有积极影响的重要领域的进展 |
| SmartPlay | microsoft | 游戏 | [SmartPlay](github.com/microsoft/SmartPlay) | SmartPlay是一个大型语言模型 (LLM) 基准,设计为易于使用,提供各种游戏来测试 |
| FinEval | SUFE-AIFLM-Lab | 金融 | [FinEval](github.com/SUFE-AIFLM-Lab/FinEval) | FinEval:包含金融、经济、会计和证书等领域高质量多项选择题的集合 |
| GSM8K | OpenAI | 数学 | [GSM8K](https://github.com/openai/grade-school-math) | GSM8K是一个包含8.5K个高质量语言多样性的小学数学单词问题的数据集。GSM8K将它们分为7.5K个训练问题和1K个测试问题。这些问题需要2到8个步骤来解决,解决方案主要涉及执行一系列基本算术运算(+ - / *)以达到最终答案 |
### RAG检索增强生成评估| 名称 | 机构 | 网址 | 简介 |
| :--: | :--: | :--: | :-- |
| BERGEN | NAVER | [BERGEN](https://github.com/naver/bergen) | BERGEN:RAG系统基准测试库,专注于问答(QA)领域的性能评估,旨在提高RAG管线中各组件影响的理解和比较的一致性,通过HuggingFace简化新数据集和模型的复现性和集成. BERGEN (BEnchmarking Retrieval-augmented GENeration), a library to benchmark RAG systems, focusing on question-answering (QA). Inconsistent benchmarking poses a major challenge in comparing approaches and understanding the impact of each component in a RAG pipeline. BERGEN was designed to ease the reproducibility and integration of new datasets and models thanks to HuggingFace. (2024-05-31) |
| CRAG | Meta Reality Labs | [CRAG](https://arxiv.org/abs/2406.04744) | CRAG是一个包含近4500个QA对和模拟API的RAG基准集,覆盖广泛领域和问题类型,可以启发各方研究人员提升问答系统的可靠性和准确性. A factual question answering benchmark of 4,409 question-answer pairs and mock APIs to simulate web and Knowledge Graph (KG) search. CRAG is designed to encapsulate a diverse array of questions across five domains and eight question categories, reflecting varied entity popularity from popular to long-tail, and temporal dynamisms ranging from years to seconds. (2024-06-07) |
| raga-llm-hub | RAGA-AI | [raga-llm-hub](https://github.com/raga-ai-hub/raga-llm-hub) | raga-llm-hub是一个全面的语言和学习模型(LLM)评估工具包。它拥有超过100个精心设计的评价指标,是允许开发者和组织有效评估和比较LLM的最具综合性的平台,并为LLM和检索增强生成(RAG)应用建立基本的防护措施。这些测试评估包括相关性与理解、内容质量、幻觉、安全与偏见、上下文相关性、防护措施以及漏洞扫描等多个方面,同时提供一系列基于指标的测试用于定量分析 (2024-03-10) |
| ARES | Stanford | [ARES](https://github.com/stanford-futuredata/ARES) | ARES是一个用于检索增强生成系统的自动评估框架,包含三个组件:(1)一组用于评估标准(例如上下文相关性、答案忠实度和/或答案相关性)的已注释查询、文档和答案三元组的人工首选验证集。至少应有50个示例,但最好有几百个示例。(2)一组用于在您的系统中评分上下文相关性、答案忠实度和/或答案相关性的少量示例。(3)由您的RAG系统输出的用于评分的大量未标记查询-文档-答案三元组。ARES培训流程包括三个步骤:(1)从领域内段落生成合成查询和答案。(2)通过在合成生成的训练数据上进行微调,为评分RAG系统准备LLM评委。(3)部署准备好的LLM评委以评估您的RAG系统在关键性能指标上的表现 (2023-09-27) |
| RGB | CAS | [RGB](https://github.com/chen700564/RGB) | RGB是一个用于英语和中文RAG评估的新语料库/检索增强生成基准(RGB),RGB分析了不同大型语言模型在RAG所需的4种基本能力方面的表现,包括噪声鲁棒性、负面拒绝、信息整合和反事实鲁棒性。RGB将基准中的实例分为4个独立的测试集,基于上述基本能力以解决案例。然后,在RGB中评估了6个代表性的LLMs,以诊断当前LLMs在应用RAG时面临的挑战。评估显示,虽然LLMs表现出一定程度的噪声鲁棒性,但在负面拒绝、信息整合和处理虚假信息方面仍然存在显著困难。上述评估结果表明,在有效应用RAG到LLMs方面仍有相当长的路要走 (2023-09-04) |
| tvalmetrics | TonicAI | [tvalmetrics](https://github.com/TonicAI/tvalmetrics) | Tonic Validate Metrics中的指标使用LLM辅助评估,这意味着它们使用一个LLM(例如gpt-4)来评分RAG应用的输出的不同方面。Tonic Validate Metrics中的指标使用这些对象和LLM辅助评估来回答有关RAG应用的问题。(1)答案相似度分数: RAG答案与答案应该是多么匹配?(2)检索精度: 检索的上下文是否与问题相关?(3)增强精度: 答案中是否包含与问题相关的检索上下文?(4)增强准确度: 检索上下文在答案中的占比如何?(5)答案一致性(二进制): 答案是否包含来自检索上下文之外的任何信息?(6)检索k-召回: 对于前k个上下文向量,在检索上下文是前k个上下文向量的子集的情况下,检索上下文是否是回答问题的前k个上下文向量中所有相关上下文? (2023-11-11) |
### Agent能力| 名称 | 机构 | 网址 | 简介 |
| :--: | :--: | :--: | :-- |
| SuperCLUE-Agent | CLUE | [SuperCLUE-Agent](https://github.com/CLUEbenchmark/SuperCLUE-Agent) | SuperCLUE-Agent,一个聚焦于Agent能力的多维度基准测试,包括3大核心能力、10大基础任务,可以用于评估大语言模型在核心Agent能力上的表现,包括工具使用、任务规划和长短期记忆能力。经过对16个支持中文的大语言模型的测评发现:在Agent的核心基础能力中文任务上,GPT4模型大幅领先;同时,代表性国内模型,包括开源和闭源模型,已经较为接近GPT3.5水平 (2023-10-20) |
| AgentBench | Tsinghua University | [AgentBench](https://github.com/THUDM/AgentBench) | AgentBench是一个用于评估LLM作为agent智能体的系统化基准评测工具,突出了商业LLM和开源竞争对手之间的性能差距 (2023-08-01) |
| AgentBench推理决策评估榜单 | THUDM | [AgentBench](https://github.com/THUDM/AgentBench) | 清华联合多所高校推出,涵盖不同任务环境,如购物、家居、操作系统等场景下模型的推理决策能力|
| ToolBench工具调用评测 | 智源/清华 | [ToolBench](https://github.com/OpenBMB/ToolBench) | 通过与工具微调模型和 ChatGPT 进行比较,提供评测脚本|
### 代码能力| 名称 | 机构 | 网址 | 简介 |
| :--: | :--: | :--: | :-- |
| McEval | Beihang | [McEval](https://github.com/MCEVAL/McEval) | 为了更加全面的探究大语言模型的代码能力,该工作提出了一个涵盖40种编程语言的大规模多语言多任务代码评测基准(McEval),包含了16000个测试样本。评测结果表明开源模型与GPT-4相比,在多语言的编程能力上仍然存在较大差距,绝大多数开源模型甚至无法超越GPT-3.5。此外测试也表明开源模型中如Codestral,DeepSeek-Coder, CodeQwen以及一些衍生模型也展现出优异的多语言能力. McEval is a massively multilingual code benchmark covering 40 programming languages with 16K test samples, which substantially pushes the limits of code LLMs in multilingual scenarios. The benchmark contains challenging code completion, understanding, and generation evaluation tasks with finely curated massively multilingual instruction corpora McEval-Instruct. McEval leaderboard can be found [here](https://mceval.github.io/). (2024-06-11) |
| HumanEval-XL | FloatAI | [SuperCLUE-Agent](https://github.com/FloatAI/HumanEval-XL) | 现有的基准测试主要集中在将英文提示翻译成多语言代码,或者局限于非常有限的自然语言。这些基准测试忽视了大规模多语言NL到多语言代码生成的广阔领域,留下了评估多语言LLMs的一个重要空白。为了应对这一挑战,作者们提出了HumanEval-XL,这是一个大规模多语言代码生成基准测试,旨在填补这一缺陷。HumanEval-XL在23种自然语言和12种编程语言之间建立了联系,包含22,080个提示,平均每个提示有8.33个测试用例。通过确保跨多种NL和PLs的平行数据,HumanEval-XL为多语言LLMs提供了一个全面的评估平台,允许评估对不同NLs的理解。这项工作是填补多语言代码生成领域NL泛化评估空白的开创性步骤。 (2024-02-26) |
| DebugBench | 清华大学 |[DebugBench](https://github.com/thunlp/DebugBench)| DebugBench是一个包含4,253个实例的LLM调试基准,涵盖了C++、Java和Python中四个主要的漏洞类别和18个次要类别。为构建DebugBench,作者从LeetCode社区收集了代码片段,使用GPT-4向源数据植入漏洞,并确保了严格的质量检查 (2024-01-09) |
### 多模态-跨模态| 名称 | 机构 | 网址 | 简介 |
| :--: | :--: | :--: | :-- |
| ChartVLM | Shanghai AI Lab | [ChartVLM](https://github.com/UniModal4Reasoning/ChartVLM) | ChartX是一个多模式评估集,包括18种图表类型、7种图表任务、22个学科主题和高质量的图表数据。此外本文作者还开发了ChartVLM,为处理依赖于可解释模式的多模态任务(比如图表或几何图像领域的推理任务)提供了新视角。 (2024-02-19) |
| ReForm-Eval | FudanDISC | [ReForm-Eval](https://github.com/FudanDISC/ReForm-Eval) | ReForm-Eval是一个用于综合评估大视觉语言模型的基准数据集。ReForm-Eval通过对已有的、不同任务形式的多模态基准数据集进行重构,构建了一个具有统一且适用于大模型评测形式的基准数据集。所构建的ReForm-Eval具有如下特点:构建了横跨8个评估维度,并为每个维度提供足量的评测数据(平均每个维度4000余条);具有统一的评测问题形式(包括单选题和文本生成问题);方便易用,评测方法可靠高效,且无需依赖ChatGPT等外部服务;高效地利用了现存的数据资源,无需额外的人工标注,并且可以进一步拓展到更多数据集上 (2023-10-24) |
| LVLM-eHub | OpenGVLab | [LVLM-eHub](https://github.com/OpenGVLab/Multi-Modality-Arena) | "Multi-Modality Arena"是一个用于大型多模态模型的评估平台。在Fastchat之后,两个匿名模型在视觉问答任务上进行并排比较,"Multi-Modality Arena"允许你在提供图像输入的同时,对视觉-语言模型进行并排基准测试。支持MiniGPT-4,LLaMA-Adapter V2,LLaVA,BLIP-2等多种模型 |
### 长上下文| 名称 | 机构 | 网址 | 简介 |
| :--: | :--: | :--: | :-- |
| InfiniteBench | OpenBMB | [InfiniteBench](https://github.com/OpenBMB/InfiniteBench) |理解、处理长文本,是大模型迈向更深层次理解与交互阶段必备的能力。现已有大模型声称可以处理100k+的长序列,但是对应的标准评测集却是空缺的。InfiniteBench构建了一个面向 100k+ 的评测集,该评测集针对大模型在长文本方面的五项能力而设计:检索、数学、代码、问答、和摘要。(1)长上下文: InfiniteBench 测试数据的平均上下文长度为195k,远超现有评测数据。(2)多领域多语言: InfiniteBench 评测集包含12个任务,包括中英双语,涵盖了检索、数学、代码、问答、和摘要等5个领域。(3)前瞻性挑战性: InfiniteBench 测试任务,对标当前最强的模型如 GPT-4, Claude 2 等。(4)真实场景与合成场景: InfiniteBench 既包含真实场景数据,探测大模型在处理实际问题的能力;也包含合成数据,为测试数据拓展上下文窗口提供了便捷. InfiniteBench is the first LLM benchmark featuring an average data length surpassing 100K tokens. InfiniteBench comprises synthetic and realistic tasks spanning diverse domains, presented in both English and Chinese. The tasks in InfiniteBench are designed to require well understanding of long dependencies in contexts, and make simply retrieving a limited number of passages from contexts not sufficient for these tasks. (2024-03-19) |
### 推理速度| 名称 | 机构 | 网址 | 简介 |
| :--: | :--: | :--: | :-- |
| llmperf | Ray | [llmperf](https://github.com/ray-project/llmperf) | 用于检验和基准测试LLM性能的库,可以测量第一个token出现的时间(TTFT)、两个token之间的响应时间(ITL)以及超过3秒没有返回数据的请求数量,还可以验证LLM的输出是否正确,主要检查是否有请求之间的交叉(请求A得到请求B的响应)。输入和输出token长度的变化也是设计考虑,目的是更好地代表实际情况。当前支持的端点包括OpenAI兼容端点(如Anyscale端点、私有端点、OpenAI、Fireworks等)、Together、Vertex AI和SageMaker (2023-11-03)|
| llm-analysis | Databricks | [llm-analysis](https://github.com/cli99/llm-analysis) | Latency and Memory Analysis of Transformer Models for Training and Inference. |
| llm-inference-benchmark | Nankai University | [llm-inference-benchmark](https://github.com/ninehills/llm-inference-benchmark) | LLM Inference framework benchmark. |
| llm-inference-bench | CentML | [llm-inference-bench](https://github.com/CentML/llm-inference-bench) | This benchmark operates entirely external to any serving framework, and can easily be extended and modified. Provides a variety of statistics and profiling modes. It is intended to be a standalone tool for precise statistically significant benchmarking with a particular input/output distribution. Each request consists of a single prompt and single decode. |
| GPU-Benchmarks-on-LLM-Inference | UIUC | [GPU-Benchmarks-on-LLM-Inference](https://github.com/XiongjieDai/GPU-Benchmarks-on-LLM-Inference) | Use llama.cpp to test the LLaMA models inference speed of different GPUs on RunPod, 16-inch M1 Max MacBook Pro, M2 Ultra Mac Studio, 14-inch M3 MacBook Pro and 16-inch M3 Max MacBook Pro. |
### 量化压缩| 名称 | 机构 | 网址 | 简介 |
| :--: | :--: | :--: | :-- |
| LLM-QBench | Beihang/SenseTime | [LLM-QBench](https://github.com/ModelTC/llmc)| LLM-QBench is a Benchmark Towards the Best Practice for Post-training Quantization of Large Language Models", and it is also an efficient LLM compression tool with various advanced compression methods, supporting multiple inference backends. (2024-05-09) |
## Demos
- [Chat Arena: anonymous models side-by-side and vote for which one is better](https://chat.lmsys.org/?arena) - 开源AI大模型“匿名”竞技场!你在这里可以成为一名裁判,给两个事先不知道名字的模型回答打分,评分后将给出他们的真实身份。目前已经“参赛”的选手包括Vicuna、Koala、OpenAssistant (oasst)、Dolly、ChatGLM、StableLM、Alpaca、LLaMA等。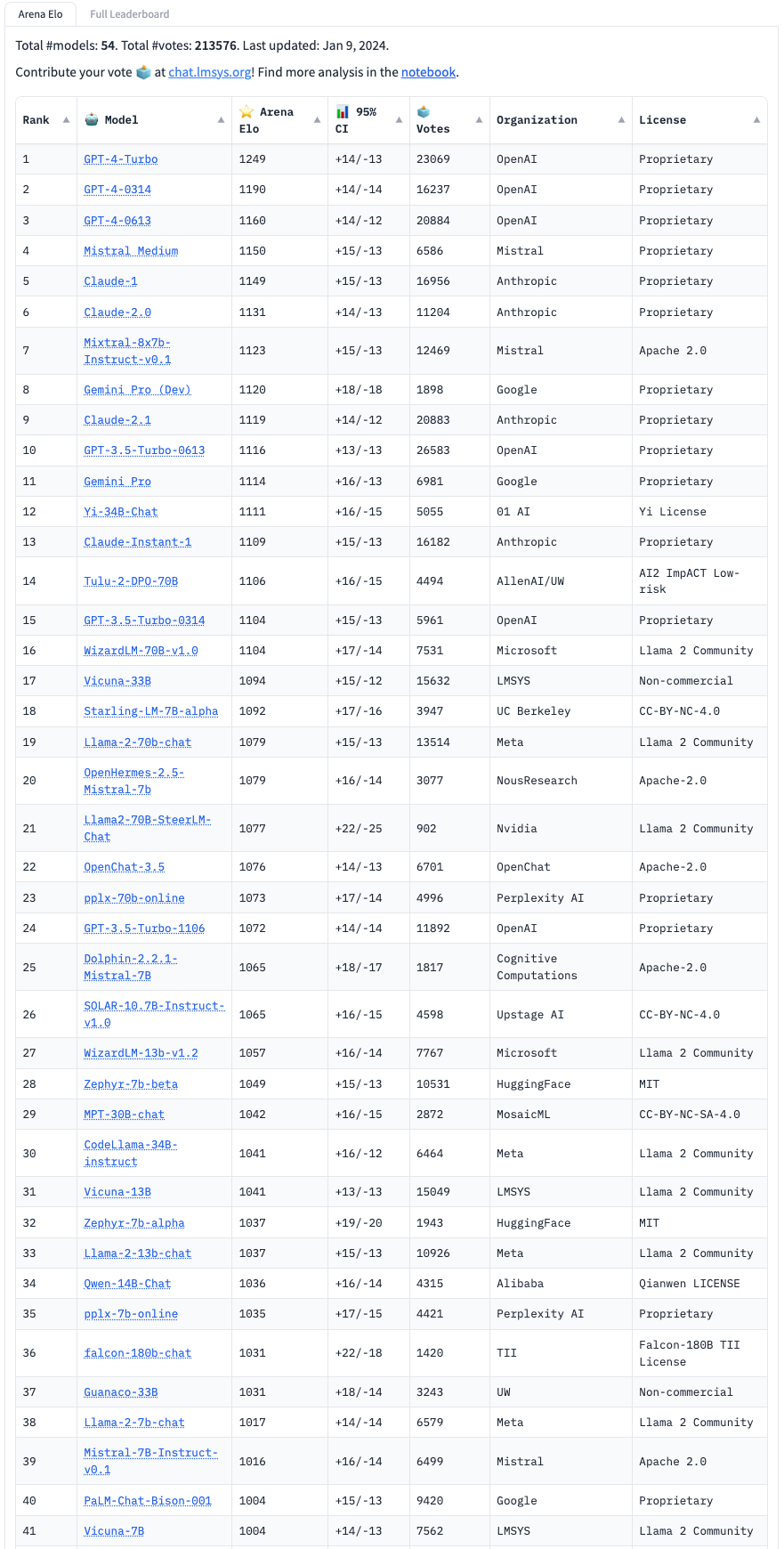
## Leaderboards| Platform | Access |
| :------------------------------------: | ---------------------------------------------------------------------------------------|
| ACLUE | [[Source](https://github.com/isen-zhang/ACLUE) |
| AgentBench | [[Source](https://llmbench.ai/agent)] |
| AlpacaEval | [[Source](https://tatsu-lab.github.io/alpaca_eval/)] |
| ANGO | [[Source](https://huggingface.co/spaces/AngoHF/ANGO-Leaderboard)] |
| BeHonest | [[Source](https://gair-nlp.github.io/BeHonest/#leaderboard)] |
| Big Code Models Leaderboard | [[Source](https://huggingface.co/spaces/bigcode/bigcode-models-leaderboard)] |
| Chatbot Arena | [[Source](https://lmarena.ai/?leaderboard)] |
| Chinese Large Model Leaderboard | [[Source](https://github.com/jeinlee1991/chinese-llm-benchmark)] |
| CLEVA | [[Source](http://www.lavicleva.com/)] |
| CompassRank | [[Source](https://rank.opencompass.org.cn/)] |
| CompMix | [[Source](https://qa.mpi-inf.mpg.de/compmix)] |
| C-Eval | [[Source](https://cevalbenchmark.com/)] |
| DreamBench++ | [[Source](https://dreambenchplus.github.io/#leaderboard)] |
| FELM | [[Source](https://hkust-nlp.github.io/felm)] |
| FlagEval | [[Source](https://flageval.baai.ac.cn/)] |
| Hallucination Leaderboard | [[Source](https://github.com/vectara/hallucination-leaderboard)] |
| HELM | [[Source](https://crfm.stanford.edu/helm/)] |
| Huggingface Open LLM Leaderboard | [[Source](https://huggingface.co/spaces/open-llm-leaderboard/open_llm_leaderboard)] |
| Huggingface LLM Perf Leaderboard | [[Source](https://huggingface.co/spaces/optimum/llm-perf-leaderboard)] |
| Indico LLM Leaderboard | [[Source](https://indicodata.ai/llm) |
| InfiBench | [[Source](https://infi-coder.github.io/infibench)] |
| InterCode | [[Source](https://intercode-benchmark.github.io/)] |
| LawBench | [[Source](https://lawbench.opencompass.org.cn/leaderboard)] |
| LLMEval | [[Source](https://openrouter.ai/rankings)] |
| LLM Rankings | [[Source](http://llmeval.com)] |
| LLM Use Case Leaderboard | [[Source](https://llmleaderboard.goml.io)] |
| LucyEval | [[Source](https://openrouter.ai/rankings)] |
| M3CoT | [[Source](https://lightchen233.github.io/m3cot.github.io/leaderboard.html)] |
| MMLU by Task Leaderboard | [[Source](https://huggingface.co/spaces/CoreyMorris/MMLU-by-task-Leaderboard)] |
| MMToM-QA | [[Source](https://chuanyangjin.com/mmtom-qa-leaderboard)] |
| MathEval | [[Source](https://matheval.ai/)] |
| OlympicArena | [[Source](https://gair-nlp.github.io/OlympicArena/#leaderboard)] |
| OpenEval | [[Source](http://openeval.org.cn/#/rank)] |
| Open Multilingual LLM Eval | [[Source](https://huggingface.co/spaces/uonlp/open_multilingual_llm_leaderboard)] |
| PubMedQA | [[Source](https://pubmedqa.github.io/)] |
| SafetyBench | [[Source](https://llmbench.ai/safety)] |
| SciBench | [[Source](https://scibench-ucla.github.io/#leaderboard)] |
| SciKnowEval | [[Source](https://github.com/HICAI-ZJU/SciKnowEval)] |
| SEED-Bench | [[Source](https://huggingface.co/spaces/AILab-CVC/SEED-Bench_Leaderboard)] |
| SuperBench | [[Source](https://fm.ai.tsinghua.edu.cn/superbench/#/leaderboard)] |
| SuperCLUE | [[Source](https://www.superclueai.com/)] |
| SuperGLUE | [[Source](https://super.gluebenchmark.com/)] |
| SuperLim | [[Source](https://lab.kb.se/leaderboard/results)] |
| TAT-DQA | [[Source](https://nextplusplus.github.io/TAT-DQA)] |
| TAT-QA | [[Source](https://nextplusplus.github.io/TAT-QA)] |
| TheoremOne LLM Benchmarking Metrics | [[Source](https://llm-evals.formula-labs.com/)] |
| Toloka | [[Source](https://toloka.ai/llm-leaderboard/)] |
| Toolbench | [[Source](https://huggingface.co/spaces/qiantong-xu/toolbench-leaderboard)] |
| VisualWebArena | [[Source](https://jykoh.com/vwa)] |
| We-Math | [[Source](https://we-math.github.io/#leaderboard)] |
| WHOOPS! | [[Source](https://whoops-benchmark.github.io)] |
### Leaderboards for popular Provider (performance and cost, 2024-05-14)
| Provider (link to pricing) | [OpenAI](https://openai.com/pricing) | [OpenAI](https://openai.com/pricing) | [Anthropic](https://www.anthropic.com/api) | [Google](https://cloud.google.com/vertex-ai/generative-ai/pricing) | [Replicate](https://replicate.com/pricing) | [DeepSeek](https://www.deepseek.com/) | [Mistral](https://docs.mistral.ai/platform/pricing/) | [Anthropic](https://www.anthropic.com/api) | [Google](https://cloud.google.com/vertex-ai/generative-ai/pricing) | [Mistral](https://docs.mistral.ai/platform/pricing/) | [Cohere](https://cohere.com/command) | [Anthropic](https://www.anthropic.com/api) | [Mistral](https://docs.mistral.ai/platform/pricing/) | [Replicate](https://replicate.com/pricing) | [Mistral](https://docs.mistral.ai/platform/pricing/) | [OpenAI](https://openai.com/pricing) | | [Groq](https://wow.groq.com/) | [OpenAI](https://openai.com/pricing) | [Mistral](https://docs.mistral.ai/platform/pricing/) | [Anthropic](https://www.anthropic.com/api) | [Groq](https://wow.groq.com/) | [Anthropic](https://www.anthropic.com/api) | [Anthropic](https://www.anthropic.com/api) | Microsoft | Microsoft | [Mistral](https://docs.mistral.ai/platform/pricing/) |
| ------------------------------------------------------------ | ------------------------------------ | ------------------------------------ | ------------------------------------------ | ------------------------------------------------------------ | ------------------------------------------------------------ | ------------------------------------------------------------ | ------------------------------------------------------------ | ------------------------------------------ | ------------------------------------------------------------ | ---------------------------------------------------- | ------------------------------------------------------------ | ------------------------------------------ | ---------------------------------------------------- | ------------------------------------------------------------ | ------------------------------------------------------------ | ------------------------------------ | ---- | ----------------------------- | ------------------------------------ | ---------------------------------------------------- | ------------------------------------------ | ----------------------------- | ------------------------------------------ | ------------------------------------------ | ------------------------------------------------------------ | ------------------------------------------------------------ | ---------------------------------------------------- |
| Model name | GPT-4o | GPT-4 Turbo | Claude 3 Opus | Gemini 1.5 Pro | [Llama 3 70B](https://huggingface.co/meta-llama/Meta-Llama-3-70B-Instruct) | [DeepSeek-V2](https://huggingface.co/deepseek-ai/DeepSeek-V2-Chat) | [Mixtral 8x22B](https://huggingface.co/mistralai/Mixtral-8x22B-Instruct-v0.1) | Claude 3 Sonnet | Gemini 1.5 Flash | Mistral Large | [Command R+](https://huggingface.co/CohereForAI/c4ai-command-r-plus) | Claude 3 Haiku | Mistral Small | [Llama 3 8B](https://huggingface.co/meta-llama/Meta-Llama-3-8B-Instruct) | [Mixtral 8x7B](https://huggingface.co/mistralai/Mixtral-8x7B-Instruct-v0.1) | GPT-3.5 Turbo | | Llama 3 70B (Groq) | GPT-4 | Mistral Medium | Claude 2.0 | Mixtral 8x7B (Groq) | Claude 2.1 | Claude Instant | [Phi-Medium 4k](https://huggingface.co/microsoft/Phi-3-medium-4k-instruct) | [Phi-3-Small 8k](https://huggingface.co/microsoft/Phi-3-small-8k-instruct) | Mistral 7B |
| Column Last Updated | 5/14/2024 | 5/14/2024 | 5/14/2024 | 5/14/2024 | 5/20/2024 | 5/20/2024 | 5/20/2024 | 5/14/2024 | 5/14/2024 | 5/20/2024 | 5/20/2024 | 5/14/2024 | 5/20/2024 | 5/21/2024 | 5/14/2024 | 5/14/2024 | | 5/14/2024 | 5/14/2024 | 5/20/2024 | 5/14/2024 | 5/14/2024 | 5/14/2024 | 5/14/2024 | 5/21/2024 | 5/21/2024 | 5/22/2024 |
| CAPABILITY | | | | | | | | | | | | | | | | | | | | | | | | | | | |
| Artificial Analysis Index | 100 | 94 | 94 | 88 | 88 | 82 | 81 | 78 | 76 | 75 | 74 | 72 | 71 | 65 | 65 | 65 | | 88 | 83 | 73 | 69 | 65 | 63 | 63 | | | 39 |
| LMSys Chatbot Arena ELO | 1310 | 1257 | 1256 | 1249 | 1208 | | | 1204 | | 1158 | 1193 | 1182 | | 1154 | 1114 | 1102 | | 1208 | 1189 | 1148 | 1126 | 1114 | 1115 | 1104 | | | 1006 |
| | | | | | | | | | | | | | | | | | | | | | | | | | | | |
| **General knowledge:** | | | | | | | | | | | | | | | | | | | | | | | | | | | |
| MMLU | 88.70 | 86.40 | 86.80 | 81.90 | 82.00 | 78.50 | 77.75 | 79.00 | 78.90 | 81.20 | 75.70 | 75.20 | 72.20 | 68.40 | 70.60 | 70.00 | | 82.00 | 86.40 | 75.30 | 78.50 | 70.60 | | 73.40 | 78.00 | 75.70 | 62.50 |
| **Math:** | | | | | | | | | | | | | | | | | | | | | | | | | | | |
| MATH | 76.60 | 73.40 | 60.10 | 58.50 | 50.40 | | | 43.10 | 54.90 | 45.00 | | 38.90 | | 30.00 | | 34.10 | | 50.40 | 52.90 | | | | | | | | |
| MGSM / GSM8K | 90.50 | 88.60 | 95.00 | | 93.00 | | | 92.30 | | | | 88.90 | | 79.60 | | 57.10 | | 93.00 | 92.00 | | | | | | 91.00 | 89.60 | |
| **Reasoning:** | | | | | | | | | | | | | | | | | | | | | | | | | | | |
| GPQA | 53.60 | 49.10 | 50.40 | 41.50 | 39.50 | | | 40.40 | 39.50 | | | 33.30 | | 34.20 | | 28.10 | | 39.50 | 35.70 | | | | | | | | |
| BIG-BENCH-HARD | | | 86.80 | 84.00 | | | | 82.90 | 85.50 | | | 73.70 | | | | 66.60 | | | 83.10 | | | | | | | | |
| DROP, F1 Score | 83.40 | 85.40 | 83.10 | | | | | 78.90 | | | | 78.40 | | | | 64.10 | | | 80.90 | | | | | | | | |
| HellaSwag | | | 95.40 | | | | | 89.00 | | 89.20 | | 85.90 | 86.90 | | 86.70 | 85.50 | | | 95.30 | 88.00 | | 86.70 | | | 82.40 | 77.00 | |
| **Code:** | | | | | | | | | | | | | | | | | | | | | | | | | | | |
| HumanEval | 90.20 | 87.60 | 84.90 | 71.90 | 81.70 | | | 73.00 | | | | 75.90 | | 62.20 | | 48.10 | | 81.70 | 67.00 | | | | | | 62.20 | 61.00 | |
| Natural2Code | | | | 77.70 | | | | | 77.20 | | | | | | | | | | | | | | | | | | |
| **Conversational:** | | | | | | | | | | | | | | | | | | | | | | | | | | | |
| MT Bench | | 93.20 | | | | | | | | | | | | | 83.00 | 83.90 | | | | 86.10 | 80.60 | 83.00 | 81.80 | 78.50 | | | 68.40 |
| Benchmark Avg Not useful - selection bias has significant impact. | 80.50 | 80.53 | 80.31 | 69.25 | 69.32 | 78.50 | 77.75 | 72.33 | 67.20 | 71.80 | 75.70 | 68.78 | 79.55 | 54.88 | 80.10 | 59.72 | | 69.32 | 74.16 | 83.13 | 79.55 | 80.10 | 81.80 | 75.95 | 78.40 | 75.83 | 65.45 |
| | | | | | | | | | | | | | | | | | | | | | | | | | | | |
| **THROUGHPUT** | | | | | | | | | | | | | | | | | | | | | | | | | | | |
| Throughput (median tokens/sec) | 90.40 | 21.10 | 25.60 | 46.20 | 26.30 | 15.60 | 76.50 | 61.30 | 161.70 | 32.30 | 42.20 | 116.70 | 80.70 | 75.40 | 60.00 | 58.60 | | 305.30 | 28.30 | 18.30 | 37.20 | 477.10 | 42.10 | 85.70 | | | 62.20 |
| Throughput (median seconds per 1K tokens) | 11.06 | 47.39 | 39.06 | 21.65 | 38.02 | 64.10 | 13.07 | 16.31 | 6.18 | 30.96 | 23.70 | 8.57 | 12.39 | 13.26 | 16.67 | 17.06 | | 3.28 | 35.34 | 54.64 | 26.88 | 2.10 | 23.75 | 11.67 | | | 16.08 |
| | | | | | | | | | | | | | | | | | | | | | | | | | | | |
| **COST** | | | | | | | | | | | | | | | | | | | | | | | | | | | |
| Cost Input (1M tokens) aka "context window tokens" | $5.00 | $10.00 | $15.00 | $7.00 | $0.65 | $0.14 | $2.00 | $3.00 | $0.35 | $4.00 | $3.00 | $0.25 | $1.00 | $0.05 | $0.70 | $0.50 | | $0.59 | $30.00 | $2.70 | $8.00 | $0.27 | $8.00 | $0.80 | | | $0.25 |
| Cost Output (1M tokens) | $15.00 | $30.00 | $75.00 | $21.00 | $2.75 | $0.28 | $6.00 | $15.00 | $0.53 | $12.00 | $15.00 | $1.25 | $3.00 | $0.25 | $0.70 | $1.50 | | $0.79 | $60.00 | $8.10 | $24.00 | $0.27 | $24.00 | $2.40 | | | $0.25 |
| Cost 1M Input + 1M Output tokens | $20.00 | $40.00 | $90.00 | $28.00 | $3.40 | $0.42 | $8.00 | $18.00 | $0.88 | $16.00 | $18.00 | $1.50 | $4.00 | $0.30 | $1.40 | $2.00 | | $1.38 | $90.00 | $10.80 | $32.00 | $0.54 | $32.00 | $3.20 | | | $0.50 |
| | | | | | | | | | | | | | | | | | | | | | | | | | | | |
| **COST VS PERFORMANCE** | | | | | | | | | | | | | | | | | | | | | | | | | | | |
| Cost 1M+1M IO tokens per AA Index point | $0.20 | $0.43 | $0.96 | $0.32 | $0.04 | $0.01 | $0.10 | $0.23 | $0.01 | $0.21 | $0.24 | $0.02 | $0.06 | $0.00 | $0.02 | $0.03 | | $0.02 | $1.08 | $0.15 | $0.46 | $0.01 | $0.51 | $0.05 | | | $0.01 |
| Cost 1M+1M IO tokens per Chatbot ELO point | $0.02 | $0.03 | $0.07 | $0.02 | $0.00 | #DIV/0! | #DIV/0! | $0.01 | #DIV/0! | $0.01 | $0.02 | $0.00 | #DIV/0! | $0.00 | $0.00 | $0.00 | | $0.00 | $0.08 | $0.01 | $0.03 | $0.00 | $0.03 | $0.00 | | | $0.00 |
| | | | | | | | | | | | | | | | | | | | | | | | | | | | |
| Cost 1M+1M IO tokens per Throughput (tokens/sec) | $0.22 | $1.90 | $3.52 | $0.61 | $0.13 | $0.03 | $0.10 | $0.29 | $0.01 | $0.50 | $0.43 | $0.01 | $0.05 | $0.00 | $0.02 | $0.03 | | $0.00 | $3.18 | $0.59 | $0.86 | $0.00 | $0.76 | $0.04 | | | $0.01 |
| | | | | | | | | | | | | | | | | | | | | | | | | | | | |
| **SPECS** | | | | | | | | | | | | | | | | | | | | | | | | | | | |
| Context Window (k) | 128 | 128 | 200 | 1,000 | 8 | 32 | 65 | 200 | 1,000 | 32 | 128 | 200 | 32 | 8 | 32 | 16 | | | 8 | 32 | 100 | 32 | 200 | 100 | 4 | 8 | 33 |
| Max Output Tokens (k) | 4 | 4 | 4 | 8 | | | | 4 | 8 | | | 4 | | | | | | | 4 | | 4 | | 4 | 4 | | | |
| Rate Limit (requests / minute) | tiered | tiered | tiered | 5 | 600 | | | tiered | 360 | | | tiered | | 600 | | tiered | | 30 | tiered | | tiered | 30 | tiered | tiered | | | |
| Rate Limit (requests / day) | tiered | tiered | tiered | 2,000 | | | | tiered | 10,000 | | | tiered | | | | tiered | | 14,400 | tiered | | tiered | 14,400 | tiered | tiered | | | |
| Rate Limit (tokens / minute) | tiered | tiered | tiered | 10,000,000 | | | | tiered | 10,000,000 | | | tiered | | | | tiered | | 6,000 | tiered | | tiered | 5,000 | tiered | tiered | | | |
## Papers- [
](https://arxiv.org/abs/2310.07289) [**Beyond Factuality: A Comprehensive Evaluation of Large Language Models as Knowledge Generators**](https://arxiv.org/abs/2310.07289),
by *Liang Chen, Yang Deng, Yatao Bian et al.*
- [](https://arxiv.org/abs/2307.03109) [**A Closer Look into Automatic Evaluation Using Large Language Models**](https://browse.arxiv.org/pdf/2310.05657.pdf),
by *Cheng-han Chiang, Hungyi Li*
- [](https://arxiv.org/abs/2307.03109) [**A Survey on Evaluation of Large Language Models**](https://arxiv.org/abs/2307.03109),
by *Yupeng Chang, Xu Wang, Jindong Wang, Yuan Wu, Linyi Yang, Kaijie Zhu, Hao Chen, Xiaoyuan Yi et al.*
- [](https://www.microsoft.com/en-us/research/publication/gpteval-nlg-evaluation-using-gpt-4-with-better-human-alignment/) [**G-Eval: NLG Evaluation using GPT-4 with Better Human Alignment**](https://www.microsoft.com/en-us/research/publication/gpteval-nlg-evaluation-using-gpt-4-with-better-human-alignment/),
by *Yang Liu, Dan Iter, Yichong Xu, Shuohang Wang, Ruochen Xu, Chenguang Zhu*
- [](https://doi.org/10.48550/arXiv.2302.04023) [**A Multitask, Multilingual, Multimodal Evaluation of ChatGPT on Reasoning,
Hallucination, and Interactivity**](https://doi.org/10.48550/arXiv.2302.04023),
by *Yejin Bang, Samuel Cahyawijaya, Nayeon Lee, Wenliang Dai, Dan Su, Bryan Wilie, Holy Lovenia, Ziwei Ji et al.*
- [](https://doi.org/10.48550/arXiv.2302.12095) [**Beyond Factuality: A Comprehensive Evaluation of Large Language Models as Knowledge Generators**](https://arxiv.org/abs/2310.07289),
by *Liang Chen, Yang Deng, Yatao Bian, Zeyu Qin, Bingzhe Wu, Tat-Seng Chua, Kam-Fai Wong*
- [](https://arxiv.org/abs/2302.06476) [**Is ChatGPT a General-Purpose Natural Language Processing Task Solver?**](https://arxiv.org/abs/2302.06476),
by *Qin, Chengwei, Zhang, Aston, Zhang, Zhuosheng, Chen, Jiaao, Yasunaga, Michihiro and Yang, Diyi*
- [](https://doi.org/10.48550/arXiv.2302.06466) [**ChatGPT versus Traditional Question Answering for Knowledge Graphs:
Current Status and Future Directions Towards Knowledge Graph Chatbots**](https://doi.org/10.48550/arXiv.2302.06466),
by *Reham Omar, Omij Mangukiya, Panos Kalnis and Essam Mansour*
- [](https://doi.org/10.48550/arXiv.2301.13867) [**Mathematical Capabilities of ChatGPT**](https://doi.org/10.48550/arXiv.2301.13867),
by *Simon Frieder, Luca Pinchetti, Ryan-Rhys Griffiths, Tommaso Salvatori, Thomas Lukasiewicz, Philipp Christian Petersen, Alexis Chevalier and Julius Berner*
- [](https://doi.org/10.48550/arXiv.2302.08081) [**Exploring the Limits of ChatGPT for Query or Aspect-based Text Summarization**](https://doi.org/10.48550/arXiv.2302.08081),
by *Xianjun Yang, Yan Li, Xinlu Zhang, Haifeng Chen and Wei Cheng*
- [](https://doi.org/10.48550/arXiv.2302.12095) [**On the Robustness of ChatGPT: An Adversarial and Out-of-distribution
Perspective**](https://doi.org/10.48550/arXiv.2302.12095),
by *Jindong Wang, Xixu Hu, Wenxin Hou, Hao Chen, Runkai Zheng, Yidong Wang, Linyi Yang, Haojun Huang et al.*
- [](https://doi.org/10.48550/arXiv.2301.04655) [**ChatGPT is not all you need. A State of the Art Review of large
Generative AI models**](https://doi.org/10.48550/arXiv.2301.04655),
by *Roberto Gozalo-Brizuela and Eduardo C. Garrido-Merch\'an*
- [](https://arxiv.org/abs/2302.10198) [**Can ChatGPT Understand Too? A Comparative Study on ChatGPT and Fine-tuned
BERT**](https://arxiv.org/abs/2302.10198),
by *Qihuang Zhong, Liang Ding, Juhua Liu, Bo Du and Dacheng Tao*
- [](https://doi.org/10.48550/arXiv.2303.07992) [**Evaluation of ChatGPT as a Question Answering System for Answering
Complex Questions**](https://doi.org/10.48550/arXiv.2303.07992),
by *Yiming Tan, Dehai Min, Yu Li, Wenbo Li, Nan Hu, Yongrui Chen and Guilin Qi*
- [](https://arxiv.org/abs/2303.16421) [**ChatGPT is a Knowledgeable but Inexperienced Solver: An Investigation of Commonsense Problem in Large Language Models**](https://arxiv.org/abs/2303.16421),
by *Ning Bian, Xianpei Han, Le Sun, Hongyu Lin, Yaojie Lu and Ben He*
- [](https://doi.org/10.48550/arXiv.2211.09110) [**Holistic Evaluation of Language Models**](https://doi.org/10.48550/arXiv.2211.09110),
by *Percy Liang, Rishi Bommasani, Tony Lee, Dimitris Tsipras, Dilara Soylu, Michihiro Yasunaga, Yian Zhang, Deepak Narayanan et al.*
- [](https://doi.org/10.48550/arXiv.2204.00498) [**Evaluating the Text-to-SQL Capabilities of Large Language Models**](https://doi.org/10.48550/arXiv.2204.00498),
by *Nitarshan Rajkumar, Raymond Li and Dzmitry Bahdanau*
- [](https://aclanthology.org/2022.coling-1.491) [**Are Visual-Linguistic Models Commonsense Knowledge Bases?**](https://aclanthology.org/2022.coling-1.491),
by *Hsiu-Yu Yang and Carina Silberer*
- [](https://doi.org/10.48550/arXiv.2212.10529) [**Is GPT-3 a Psychopath? Evaluating Large Language Models from a Psychological
Perspective**](https://doi.org/10.48550/arXiv.2212.10529),
by *Xingxuan Li, Yutong Li, Linlin Liu, Lidong Bing and Shafiq R. Joty*
- [](https://aclanthology.org/2022.emnlp-main.132) [**GeoMLAMA: Geo-Diverse Commonsense Probing on Multilingual Pre-Trained
Language Models**](https://aclanthology.org/2022.emnlp-main.132),
by *Da Yin, Hritik Bansal, Masoud Monajatipoor, Liunian Harold Li and Kai-Wei Chang*
- [](https://aclanthology.org/2022.emnlp-main.653) [**RobustLR: A Diagnostic Benchmark for Evaluating Logical Robustness
of Deductive Reasoners**](https://aclanthology.org/2022.emnlp-main.653),
by *Soumya Sanyal, Zeyi Liao and Xiang Ren*
- [](https://arxiv.org/abs/2202.13169) [**A Systematic Evaluation of Large Language Models of Code**](https://arxiv.org/abs/2202.13169),
by *Frank F. Xu, Uri Alon, Graham Neubig and Vincent J. Hellendoorn*
- [](https://arxiv.org/abs/2107.03374) [**Evaluating Large Language Models Trained on Code**](https://arxiv.org/abs/2107.03374),
by *Mark Chen, Jerry Tworek, Heewoo Jun, Qiming Yuan, Henrique Pond\'e de Oliveira Pinto, Jared Kaplan, Harrison Edwards, Yuri Burda et al.*
- [](https://doi.org/10.18653/v1/2021.findings-acl.36) [**GLGE: A New General Language Generation Evaluation Benchmark**](https://doi.org/10.18653/v1/2021.findings-acl.36),
by *Dayiheng Liu, Yu Yan, Yeyun Gong, Weizhen Qi, Hang Zhang, Jian Jiao, Weizhu Chen, Jie Fu et al.*
- [](https://arxiv.org/abs/2104.05861) [**Evaluating Pre-Trained Models for User Feedback Analysis in Software
Engineering: A Study on Classification of App-Reviews**](https://arxiv.org/abs/2104.05861),
by *Mohammad Abdul Hadi and Fatemeh H. Fard*
- [](https://doi.org/10.18653/v1/2021.findings-acl.322) [**Do Language Models Perform Generalizable Commonsense Inference?**](https://doi.org/10.18653/v1/2021.findings-acl.322), [
](https://github.com/wangpf3/LM-for-CommonsenseInference)
by *Peifeng Wang, Filip Ilievski, Muhao Chen and Xiang Ren*
- [](https://doi.org/10.18653/v1/2021.emnlp-main.598) [**RICA: Evaluating Robust Inference Capabilities Based on Commonsense
Axioms**](https://doi.org/10.18653/v1/2021.emnlp-main.598),
by *Pei Zhou, Rahul Khanna, Seyeon Lee, Bill Yuchen Lin, Daniel Ho, Jay Pujara and Xiang Ren*
- [](https://arxiv.org/abs/2006.14799) [**Evaluation of Text Generation: A Survey**](https://arxiv.org/abs/2006.14799),
by *Asli Celikyilmaz, Elizabeth Clark and Jianfeng Gao*
- [](https://arxiv.org/abs/2007.15780) [**Neural Language Generation: Formulation, Methods, and Evaluation**](https://arxiv.org/abs/2007.15780),
by *Cristina Garbacea and Qiaozhu Mei*
- [](https://openreview.net/forum?id=SkeHuCVFDr) [**BERTScore: Evaluating Text Generation with BERT**](https://openreview.net/forum?id=SkeHuCVFDr),
by *Tianyi Zhang, Varsha Kishore, Felix Wu, Kilian Q. Weinberger and Yoav Artzi*
## LLM-List### Typical LLM details
| 模型 | 参数规模 | 层数 | 自注意力头数 | 嵌入表示维度 | 学习率 | 全局批次大小 | 训练Token数 |
|--------------|----------|------|------------|--------------|---------------|--------------|----------------|
| LLaMA2 | 6.7B | 32 | 32 | 4096 | 3.00E-04 | 400万 | 1.0万亿 |
| LLaMA2 | 13.0B | 40 | 40 | 5120 | 3.00E-04 | 400万 | 1.0万亿 |
| LLaMA2 | 32.5B | 60 | 52 | 6656 | 1.50E-04 | 400万 | 1.4万亿 |
| LLaMA2 | 65.2B | 80 | 64 | 8192 | 1.50E-04 | 400万 | 1.4万亿 |
| nano-GPT | 85,584 | 3 | 3 | 768 | 3.00E-04 | | |
| GPT2-small | 0.12B | 12 | 12 | 768 | 2.50E-04 | | |
| GPT2-XL | 1.5B | 48 | 25 | 1600 | 1.50E-04 | | |
| GPT3 | 175B | 96 | 96 | 12288 | 1.50E-04 | | 0.5万亿 |### Pre-trained-LLM
| Model | Size | Architecture | Access | Date | Origin |
| :----------------: | :--: | :-------------: | :---------------------------------------------------------------------------------------------------------------------------------------------------------------------------------------------------: | :-----: | ----------------------------------------------------------------------------------------------------------------------------- |
| Switch Transformer | 1.6T | Decoder(MOE) | - | 2021-01 | [Paper](https://arxiv.org/pdf/2101.03961.pdf) |
| GLaM | 1.2T | Decoder(MOE) | - | 2021-12 | [Paper](https://arxiv.org/pdf/2112.06905.pdf) |
| PaLM | 540B | Decoder | - | 2022-04 | [Paper](https://arxiv.org/pdf/2204.02311.pdf) |
| MT-NLG | 530B | Decoder | - | 2022-01 | [Paper](https://arxiv.org/pdf/2201.11990.pdf) |
| J1-Jumbo | 178B | Decoder | [api](https://docs.ai21.com/docs/complete-api) | 2021-08 | [Paper](https://uploads-ssl.webflow.com/60fd4503684b466578c0d307/61138924626a6981ee09caf6_jurassic_tech_paper.pdf) |
| OPT | 175B | Decoder | [api](https://opt.alpa.ai) \| [ckpt](https://github.com/facebookresearch/metaseq/tree/main/projects/OPT) | 2022-05 | [Paper](https://arxiv.org/pdf/2205.01068.pdf) |
| BLOOM | 176B | Decoder | [api](https://huggingface.co/bigscience/bloom) \| [ckpt](https://huggingface.co/bigscience/bloom) | 2022-11 | [Paper](https://arxiv.org/pdf/2211.05100.pdf) |
| GPT 3.0 | 175B | Decoder | [api](https://openai.com/api/) | 2020-05 | [Paper](https://arxiv.org/pdf/2005.14165.pdf) |
| LaMDA | 137B | Decoder | - | 2022-01 | [Paper](https://arxiv.org/pdf/2201.08239.pdf) |
| GLM | 130B | Decoder | [ckpt](https://github.com/THUDM/GLM-130B) | 2022-10 | [Paper](https://arxiv.org/pdf/2210.02414.pdf) |
| YaLM | 100B | Decoder | [ckpt](https://github.com/yandex/YaLM-100B) | 2022-06 | [Blog](https://medium.com/yandex/yandex-publishes-yalm-100b-its-the-largest-gpt-like-neural-network-in-open-source-d1df53d0e9a6) |
| LLaMA | 65B | Decoder | [ckpt](https://github.com/facebookresearch/llama) | 2022-09 | [Paper](https://research.facebook.com/publications/llama-open-and-efficient-foundation-language-models/) |
| GPT-NeoX | 20B | Decoder | [ckpt](https://github.com/EleutherAI/gpt-neox) | 2022-04 | [Paper](https://arxiv.org/pdf/2204.06745.pdf) |
| UL2 | 20B | agnostic | [ckpt](https://huggingface.co/google/ul2#:~:text=UL2%20is%20a%20unified%20framework%20for%20pretraining%20models,downstream%20fine-tuning%20is%20associated%20with%20specific%20pre-training%20schemes.) | 2022-05 | [Paper](https://arxiv.org/pdf/2205.05131v1.pdf) |
| 鹏程.盘古α | 13B | Decoder | [ckpt](https://github.com/huawei-noah/Pretrained-Language-Model/tree/master/PanGu-α#模型下载) | 2021-04 | [Paper](https://arxiv.org/pdf/2104.12369.pdf) |
| T5 | 11B | Encoder-Decoder | [ckpt](https://huggingface.co/t5-11b) | 2019-10 | [Paper](https://jmlr.org/papers/v21/20-074.html) |
| CPM-Bee | 10B | Decoder | [api](https://live.openbmb.org/models/bee) | 2022-10 | [Paper](https://arxiv.org/pdf/2012.00413.pdf) |
| rwkv-4 | 7B | RWKV | [ckpt](https://huggingface.co/BlinkDL/rwkv-4-pile-7b) | 2022-09 | [Github](https://github.com/BlinkDL/RWKV-LM) |
| GPT-J | 6B | Decoder | [ckpt](https://huggingface.co/EleutherAI/gpt-j-6B) | 2022-09 | [Github](https://github.com/kingoflolz/mesh-transformer-jax) |
| GPT-Neo | 2.7B | Decoder | [ckpt](https://github.com/EleutherAI/gpt-neo) | 2021-03 | [Github](https://github.com/EleutherAI/gpt-neo) |
| GPT-Neo | 1.3B | Decoder | [ckpt](https://github.com/EleutherAI/gpt-neo) | 2021-03 | [Github](https://github.com/EleutherAI/gpt-neo) |
### Instruction-finetuned-LLM
| Model | Size | Architecture | Access | Date | Origin |
| :----------------: | :--: | :-------------: | :---------------------------------------------------------------------------------------------------------------------------------------------------------------------------------------------------: | :-----: | ----------------------------------------------------------------------------------------------------------------------------- |
|Flan-PaLM| 540B | Decoder |-|2022-10|[Paper](https://arxiv.org/pdf/2210.11416.pdf)|
|BLOOMZ| 176B | Decoder | [ckpt](https://huggingface.co/bigscience/bloomz) |2022-11|[Paper](https://arxiv.org/pdf/2211.01786.pdf)|
| InstructGPT |175B| Decoder | [api](https://platform.openai.com/overview) | 2022-03 | [Paper](https://arxiv.org/pdf/2203.02155.pdf) |
|Galactica|120B|Decoder|[ckpt](https://huggingface.co/facebook/galactica-120b)|2022-11| [Paper](https://arxiv.org/pdf/2211.09085.pdf)|
| OpenChatKit| 20B | - |[ckpt](https://github.com/togethercomputer/OpenChatKit)| 2023-3 |-|
| Flan-UL2| 20B | Decoder | [ckpt](https://github.com/google-research/google-research/tree/master/ul2)|2023-03 | [Blog](https://www.yitay.net/blog/flan-ul2-20b)|
| Gopher | - | - | - | - | - |
| Chinchilla | - | - | - | - |- |
|Flan-T5| 11B | Encoder-Decoder |[ckpt](https://github.com/google-research/t5x/blob/main/docs/models.md#flan-t5-checkpoints)|2022-10|[Paper](https://arxiv.org/pdf/2210.11416.pdf)|
|T0|11B|Encoder-Decoder|[ckpt](https://huggingface.co/bigscience/T0)|2021-10|[Paper](https://arxiv.org/pdf/2110.08207.pdf)|
|Alpaca| 7B|Decoder|[demo](https://crfm.stanford.edu/alpaca/)|2023-03|[Github](https://github.com/tatsu-lab/stanford_alpaca)|
### Aligned-LLM
| Model | Size | Architecture | Access | Date | Origin |
| :----------------: | :--: | :-------------: | :---------------------------------------------------------------------------------------------------------------------------------------------------------------------------------------------------: | :-----: | ----------------------------------------------------------------------------------------------------------------------------- |
| GPT 4 | - | - | - | 2023-03 | [Blog](https://openai.com/research/gpt-4)|
| ChatGPT | - | Decoder | [demo](https://openai.com/blog/chatgpt/)\|[api](https://share.hsforms.com/1u4goaXwDRKC9-x9IvKno0A4sk30) | 2022-11 | [Blog](https://openai.com/blog/chatgpt/) |
| Sparrow | 70B | - | - | 2022-09 | [Paper](https://arxiv.org/pdf/2209.14375.pdf)|
| Claude | - | - | [demo](https://poe.com/claude)\|[api](https://www.anthropic.com/earlyaccess) | 2023-03 | [Blog](https://www.anthropic.com/index/introducing-claude) |
### Open-LLM- [LLaMA](https://ai.facebook.com/blog/large-language-model-llama-meta-ai/) - A foundational, 65-billion-parameter large language model. [LLaMA.cpp](https://github.com/ggerganov/llama.cpp) [Lit-LLaMA](https://github.com/Lightning-AI/lit-llama)
- [Alpaca](https://crfm.stanford.edu/2023/03/13/alpaca.html) - A model fine-tuned from the LLaMA 7B model on 52K instruction-following demonstrations. [Alpaca.cpp](https://github.com/antimatter15/alpaca.cpp) [Alpaca-LoRA](https://github.com/tloen/alpaca-lora)
- [Flan-Alpaca](https://github.com/declare-lab/flan-alpaca) - Instruction Tuning from Humans and Machines.
- [Baize](https://github.com/project-baize/baize-chatbot) - Baize is an open-source chat model trained with [LoRA](https://github.com/microsoft/LoRA). It uses 100k dialogs generated by letting ChatGPT chat with itself.
- [Cabrita](https://github.com/22-hours/cabrita) - A portuguese finetuned instruction LLaMA.
- [Vicuna](https://github.com/lm-sys/FastChat) - An Open-Source Chatbot Impressing GPT-4 with 90% ChatGPT Quality.
- [Llama-X](https://github.com/AetherCortex/Llama-X) - Open Academic Research on Improving LLaMA to SOTA LLM.
- [Chinese-Vicuna](https://github.com/Facico/Chinese-Vicuna) - A Chinese Instruction-following LLaMA-based Model.
- [GPTQ-for-LLaMA](https://github.com/qwopqwop200/GPTQ-for-LLaMa) - 4 bits quantization of [LLaMA](https://arxiv.org/abs/2302.13971) using [GPTQ](https://arxiv.org/abs/2210.17323).
- [GPT4All](https://github.com/nomic-ai/gpt4all) - Demo, data, and code to train open-source assistant-style large language model based on GPT-J and LLaMa.
- [Koala](https://bair.berkeley.edu/blog/2023/04/03/koala/) - A Dialogue Model for Academic Research
- [BELLE](https://github.com/LianjiaTech/BELLE) - Be Everyone's Large Language model Engine
- [StackLLaMA](https://huggingface.co/blog/stackllama) - A hands-on guide to train LLaMA with RLHF.
- [RedPajama](https://github.com/togethercomputer/RedPajama-Data) - An Open Source Recipe to Reproduce LLaMA training dataset.
- [Chimera](https://github.com/FreedomIntelligence/LLMZoo) - Latin Phoenix.
- [BLOOM](https://huggingface.co/bigscience/bloom) - BigScience Large Open-science Open-access Multilingual Language Model [BLOOM-LoRA](https://github.com/linhduongtuan/BLOOM-LORA)
- [BLOOMZ&mT0](https://huggingface.co/bigscience/bloomz) - a family of models capable of following human instructions in dozens of languages zero-shot.
- [Phoenix](https://github.com/FreedomIntelligence/LLMZoo)
- [T5](https://arxiv.org/abs/1910.10683) - Text-to-Text Transfer Transformer
- [T0](https://arxiv.org/abs/2110.08207) - Multitask Prompted Training Enables Zero-Shot Task Generalization- [OPT](https://arxiv.org/abs/2205.01068) - Open Pre-trained Transformer Language Models.
- [UL2](https://arxiv.org/abs/2205.05131v1) - a unified framework for pretraining models that are universally effective across datasets and setups.
- [GLM](https://github.com/THUDM/GLM)- GLM is a General Language Model pretrained with an autoregressive blank-filling objective and can be finetuned on various natural language understanding and generation tasks.
- [ChatGLM-6B](https://github.com/THUDM/ChatGLM-6B) - ChatGLM-6B 是一个开源的、支持中英双语的对话语言模型,基于 [General Language Model (GLM)](https://github.com/THUDM/GLM) 架构,具有 62 亿参数.
- [ChatGLM2-6B](https://github.com/THUDM/ChatGLM2-6B) -开源中英双语对话模型 ChatGLM-6B 的第二代版本,在保留了初代模型对话流畅、部署门槛较低等众多优秀特性的基础之上,ChatGLM2-6B 引入了更长的上下文、更好的性能和更高效的推理.
- [RWKV](https://github.com/BlinkDL/RWKV-LM) - Parallelizable RNN with Transformer-level LLM Performance.
- [ChatRWKV](https://github.com/BlinkDL/ChatRWKV) - ChatRWKV is like ChatGPT but powered by my RWKV (100% RNN) language model.
- [StableLM](https://stability.ai/blog/stability-ai-launches-the-first-of-its-stablelm-suite-of-language-models) - Stability AI Language Models.
- [YaLM](https://medium.com/yandex/yandex-publishes-yalm-100b-its-the-largest-gpt-like-neural-network-in-open-source-d1df53d0e9a6) - a GPT-like neural network for generating and processing text. It can be used freely by developers and researchers from all over the world.
- [GPT-Neo](https://github.com/EleutherAI/gpt-neo) - An implementation of model & data parallel [GPT3](https://arxiv.org/abs/2005.14165)-like models using the [mesh-tensorflow](https://github.com/tensorflow/mesh) library.
- [GPT-J](https://github.com/kingoflolz/mesh-transformer-jax/#gpt-j-6b) - A 6 billion parameter, autoregressive text generation model trained on [The Pile](https://pile.eleuther.ai/).
- [Dolly](https://www.databricks.com/blog/2023/03/24/hello-dolly-democratizing-magic-chatgpt-open-models.html) - a cheap-to-build LLM that exhibits a surprising degree of the instruction following capabilities exhibited by ChatGPT.- [Pythia](https://github.com/EleutherAI/pythia) - Interpreting Autoregressive Transformers Across Time and Scale
- [Dolly 2.0](https://www.databricks.com/blog/2023/04/12/dolly-first-open-commercially-viable-instruction-tuned-llm) - the first open source, instruction-following LLM, fine-tuned on a human-generated instruction dataset licensed for research and commercial use.
- [OpenFlamingo](https://github.com/mlfoundations/open_flamingo) - an open-source reproduction of DeepMind's Flamingo model.
- [Cerebras-GPT](https://www.cerebras.net/blog/cerebras-gpt-a-family-of-open-compute-efficient-large-language-models/) - A Family of Open, Compute-efficient, Large Language Models.
- [GALACTICA](https://github.com/paperswithcode/galai/blob/main/docs/model_card.md) - The GALACTICA models are trained on a large-scale scientific corpus.
- [GALPACA](https://huggingface.co/GeorgiaTechResearchInstitute/galpaca-30b) - GALACTICA 30B fine-tuned on the Alpaca dataset.- [Palmyra](https://huggingface.co/Writer/palmyra-base) - Palmyra Base was primarily pre-trained with English text.
- [Camel](https://huggingface.co/Writer/camel-5b-hf) - a state-of-the-art instruction-following large language model designed to deliver exceptional performance and versatility.
- [h2oGPT](https://github.com/h2oai/h2ogpt)
- [PanGu-α](https://openi.org.cn/pangu/) - PanGu-α is a 200B parameter autoregressive pretrained Chinese language model develped by Huawei Noah's Ark Lab, MindSpore Team and Peng Cheng Laboratory.
- [MOSS](https://github.com/OpenLMLab/MOSS) - MOSS是一个支持中英双语和多种插件的开源对话语言模型.
- [Open-Assistant](https://github.com/LAION-AI/Open-Assistant) - a project meant to give everyone access to a great chat based large language model.
- [HuggingChat](https://huggingface.co/chat/) - Powered by Open Assistant's latest model – the best open source chat model right now and @huggingface Inference API.
- [Baichuan](https://github.com/baichuan-inc/Baichuan-13B) - An open-source, commercially available large-scale language model developed by Baichuan Intelligent Technology following Baichuan-7B, containing 13 billion parameters. (20230715)
- [Qwen](https://github.com/QwenLM/Qwen-7B) - Qwen-7B is the 7B-parameter version of the large language model series, Qwen (abbr. Tongyi Qianwen), proposed by Alibaba Cloud. Qwen-7B is a Transformer-based large language model, which is pretrained on a large volume of data, including web texts, books, codes, etc. (20230803)
### Popular-LLM| **Model** | **\#Author** | **\#Link** | **\#Parameter** | **Base Model** | **\#Layer** | **\#Encoder** | **\#Decoder** | **\#Pretrain Tokens** | **\#IFT Sample** | **RLHF** |
|:------------------------------------------------------------------------------------------------------------------------------------------------------------------------------------------------------------------------------------------------------------------------------------------:|:--------------------:|:----------------------:|:----------------------:|:----------------------:|:----------------:|:------------------:|:------------------:|:--------------------------:|:---------------------:|:-------------:|
| GPT3-Ada | brown2020language | https://platform.openai.com/docs/models/gpt-3 | 0.35B | - | 24 | - | 24 | - | - | - |
| Pythia-1B | biderman2023pythia | https://huggingface.co/EleutherAI/pythia-1b | 1B | - | 16 | - | 16 | 300B tokens | - | - |
| GPT3-Babbage | brown2020language | https://platform.openai.com/docs/models/gpt-3 | 1.3B | - | 24 | - | 24 | - | - | - |
| GPT2-XL | radford2019language | https://huggingface.co/gpt2-xl | 1.5B | - | 48 | - | 48 | 40B tokens | - | - |
| BLOOM-1b7 | scao2022bloom | https://huggingface.co/bigscience/bloom-1b7 | 1.7B | - | 24 | - | 24 | 350B tokens | - | - |
| BLOOMZ-1b7 | muennighoff2022crosslingual | https://huggingface.co/bigscience/bloomz-1b7 | 1.7B | BLOOM-1b7 | 24 | - | 24 | - | 8.39B tokens | - |
| Dolly-v2-3b | 2023dolly | https://huggingface.co/databricks/dolly-v2-3b | 2.8B | Pythia-2.8B | 32 | - | 32 | - | 15K | - |
| Pythia-2.8B | biderman2023pythia | https://huggingface.co/EleutherAI/pythia-2.8b | 2.8B | - | 32 | - | 32 | 300B tokens | - | - |
| BLOOM-3b | scao2022bloom | https://huggingface.co/bigscience/bloom-3b | 3B | - | 30 | - | 30 | 350B tokens | - | - |
| BLOOMZ-3b | muennighoff2022crosslingual | https://huggingface.co/bigscience/bloomz-3b | 3B | BLOOM-3b | 30 | - | 30 | - | 8.39B tokens | - |
| StableLM-Base-Alpha-3B | 2023StableLM | https://huggingface.co/stabilityai/stablelm-base-alpha-3b | 3B | - | 16 | - | 16 | 800B tokens | - | - |
| StableLM-Tuned-Alpha-3B | 2023StableLM | https://huggingface.co/stabilityai/stablelm-tuned-alpha-3b | 3B | StableLM-Base-Alpha-3B | 16 | - | 16 | - | 632K | - |
| ChatGLM-6B | zeng2023glm-130b,du2022glm | https://huggingface.co/THUDM/chatglm-6b | 6B | - | 28 | 28 | 28 | 1T tokens | \checkmark | \checkmark |
| DoctorGLM | xiong2023doctorglm | https://github.com/xionghonglin/DoctorGLM | 6B | ChatGLM-6B | 28 | 28 | 28 | - | 6.38M | - |
| ChatGLM-Med | ChatGLM-Med | https://github.com/SCIR-HI/Med-ChatGLM | 6B | ChatGLM-6B | 28 | 28 | 28 | - | 8K | - |
| GPT3-Curie | brown2020language | https://platform.openai.com/docs/models/gpt-3 | 6.7B | - | 32 | - | 32 | - | - | - |
| MPT-7B-Chat | MosaicML2023Introducing | https://huggingface.co/mosaicml/mpt-7b-chat | 6.7B | MPT-7B | 32 | - | 32 | - | 360K | - |
| MPT-7B-Instruct | MosaicML2023Introducing | https://huggingface.co/mosaicml/mpt-7b-instruct | 6.7B | MPT-7B | 32 | - | 32 | - | 59.3K | - |
| MPT-7B-StoryWriter-65k+ | MosaicML2023Introducing | https://huggingface.co/mosaicml/mpt-7b-storywriter | 6.7B | MPT-7B | 32 | - | 32 | - | \checkmark | - |
| Dolly-v2-7b | 2023dolly | https://huggingface.co/databricks/dolly-v2-7b | 6.9B | Pythia-6.9B | 32 | - | 32 | - | 15K | - |
| h2ogpt-oig-oasst1-512-6.9b | 2023h2ogpt | https://huggingface.co/h2oai/h2ogpt-oig-oasst1-512-6.9b | 6.9B | Pythia-6.9B | 32 | - | 32 | - | 398K | - |
| Pythia-6.9B | biderman2023pythia | https://huggingface.co/EleutherAI/pythia-6.9b | 6.9B | - | 32 | - | 32 | 300B tokens | - | - |
| Alpaca-7B | alpaca | https://huggingface.co/tatsu-lab/alpaca-7b-wdiff | 7B | LLaMA-7B | 32 | - | 32 | - | 52K | - |
| Alpaca-LoRA-7B | 2023alpacalora | https://huggingface.co/tloen/alpaca-lora-7b | 7B | LLaMA-7B | 32 | - | 32 | - | 52K | - |
| Baize-7B | xu2023baize | https://huggingface.co/project-baize/baize-lora-7B | 7B | LLaMA-7B | 32 | - | 32 | - | 263K | - |
| Baize Healthcare-7B | xu2023baize | https://huggingface.co/project-baize/baize-healthcare-lora-7B | 7B | LLaMA-7B | 32 | - | 32 | - | 201K | - |
| ChatDoctor | yunxiang2023chatdoctor | https://github.com/Kent0n-Li/ChatDoctor | 7B | LLaMA-7B | 32 | - | 32 | - | 167K | - |
| HuaTuo | wang2023huatuo | https://github.com/scir-hi/huatuo-llama-med-chinese | 7B | LLaMA-7B | 32 | - | 32 | - | 8K | - |
| Koala-7B | koala_blogpost_2023 | https://huggingface.co/young-geng/koala | 7B | LLaMA-7B | 32 | - | 32 | - | 472K | - |
| LLaMA-7B | touvron2023llama | https://huggingface.co/decapoda-research/llama-7b-hf | 7B | - | 32 | - | 32 | 1T tokens | - | - |
| Luotuo-lora-7b-0.3 | luotuo | https://huggingface.co/silk-road/luotuo-lora-7b-0.3 | 7B | LLaMA-7B | 32 | - | 32 | - | 152K | - |
| StableLM-Base-Alpha-7B | 2023StableLM | https://huggingface.co/stabilityai/stablelm-base-alpha-7b | 7B | - | 16 | - | 16 | 800B tokens | - | - |
| StableLM-Tuned-Alpha-7B | 2023StableLM | https://huggingface.co/stabilityai/stablelm-tuned-alpha-7b | 7B | StableLM-Base-Alpha-7B | 16 | - | 16 | - | 632K | - |
| Vicuna-7b-delta-v1.1 | vicuna2023 | https://github.com/lm-sys/FastChat\#vicuna-weights | 7B | LLaMA-7B | 32 | - | 32 | - | 70K | - |
| BELLE-7B-0.2M /0.6M /1M /2M | belle2023exploring | https://huggingface.co/BelleGroup/BELLE-7B-2M | 7.1B | Bloomz-7b1-mt | 30 | - | 30 | - | 0.2M/0.6M/1M/2M | - |
| BLOOM-7b1 | scao2022bloom | https://huggingface.co/bigscience/bloom-7b1 | 7.1B | - | 30 | - | 30 | 350B tokens | - | - |
| BLOOMZ-7b1 /mt /p3 | muennighoff2022crosslingual | https://huggingface.co/bigscience/bloomz-7b1-p3 | 7.1B | BLOOM-7b1 | 30 | - | 30 | - | 4.19B tokens | - |
| Dolly-v2-12b | 2023dolly | https://huggingface.co/databricks/dolly-v2-12b | 12B | Pythia-12B | 36 | - | 36 | - | 15K | - |
| h2ogpt-oasst1-512-12b | 2023h2ogpt | https://huggingface.co/h2oai/h2ogpt-oasst1-512-12b | 12B | Pythia-12B | 36 | - | 36 | - | 94.6K | - |
| Open-Assistant-SFT-4-12B | 2023openassistant | https://huggingface.co/OpenAssistant/oasst-sft-4-pythia-12b-epoch-3.5 | 12B | Pythia-12B-deduped | 36 | - | 36 | - | 161K | - |
| Pythia-12B | biderman2023pythia | https://huggingface.co/EleutherAI/pythia-12b | 12B | - | 36 | - | 36 | 300B tokens | - | - |
| Baize-13B | xu2023baize | https://huggingface.co/project-baize/baize-lora-13B | 13B | LLaMA-13B | 40 | - | 40 | - | 263K | - |
| Koala-13B | koala_blogpost_2023 | https://huggingface.co/young-geng/koala | 13B | LLaMA-13B | 40 | - | 40 | - | 472K | - |
| LLaMA-13B | touvron2023llama | https://huggingface.co/decapoda-research/llama-13b-hf | 13B | - | 40 | - | 40 | 1T tokens | - | - |
| StableVicuna-13B | 2023StableLM | https://huggingface.co/CarperAI/stable-vicuna-13b-delta | 13B | Vicuna-13B v0 | 40 | - | 40 | - | 613K | \checkmark |
| Vicuna-13b-delta-v1.1 | vicuna2023 | https://github.com/lm-sys/FastChat\#vicuna-weights | 13B | LLaMA-13B | 40 | - | 40 | - | 70K | - |
| moss-moon-003-sft | 2023moss | https://huggingface.co/fnlp/moss-moon-003-sft | 16B | moss-moon-003-base | 34 | - | 34 | - | 1.1M | - |
| moss-moon-003-sft-plugin | 2023moss | https://huggingface.co/fnlp/moss-moon-003-sft-plugin | 16B | moss-moon-003-base | 34 | - | 34 | - | 1.4M | - |
| GPT-NeoX-20B | gptneox | https://huggingface.co/EleutherAI/gpt-neox-20b | 20B | - | 44 | - | 44 | 825GB | - | - |
| h2ogpt-oasst1-512-20b | 2023h2ogpt | https://huggingface.co/h2oai/h2ogpt-oasst1-512-20b | 20B | GPT-NeoX-20B | 44 | - | 44 | - | 94.6K | - |
| Baize-30B | xu2023baize | https://huggingface.co/project-baize/baize-lora-30B | 33B | LLaMA-30B | 60 | - | 60 | - | 263K | - |
| LLaMA-30B | touvron2023llama | https://huggingface.co/decapoda-research/llama-30b-hf | 33B | - | 60 | - | 60 | 1.4T tokens | - | - |
| LLaMA-65B | touvron2023llama | https://huggingface.co/decapoda-research/llama-65b-hf | 65B | - | 80 | - | 80 | 1.4T tokens | - | - |
| GPT3-Davinci | brown2020language | https://platform.openai.com/docs/models/gpt-3 | 175B | - | 96 | - | 96 | 300B tokens | - | - |
| BLOOM | scao2022bloom | https://huggingface.co/bigscience/bloom | 176B | - | 70 | - | 70 | 366B tokens | - | - |
| BLOOMZ /mt /p3 | muennighoff2022crosslingual | https://huggingface.co/bigscience/bloomz-p3 | 176B | BLOOM | 70 | - | 70 | - | 2.09B tokens | - |
| ChatGPT~(2023.05.01) | openaichatgpt | https://platform.openai.com/docs/models/gpt-3-5 | - | GPT-3.5 | - | - | - | - | \checkmark | \checkmark |
| GPT-4~(2023.05.01) | openai2023gpt4 | https://platform.openai.com/docs/models/gpt-4 | - | - | - | - | - | - | \checkmark | \checkmark |
## Frameworks-for-Training- [Accelerate](https://github.com/huggingface/accelerate)  - 🚀 A simple way to train and use PyTorch models with multi-GPU, TPU, mixed-precision.
- [Apache MXNet](https://github.com/apache/mxnet)  - Lightweight, Portable, Flexible Distributed/Mobile Deep Learning with Dynamic, Mutation-aware Dataflow Dep Scheduler.
- [Caffe](https://github.com/BVLC/caffe)  - A fast open framework for deep learning.
- [ColossalAI](https://github.com/hpcaitech/ColossalAI)  - An integrated large-scale model training system with efficient parallelization techniques.
- [DeepSpeed](https://github.com/microsoft/DeepSpeed)  - DeepSpeed is a deep learning optimization library that makes distributed training and inference easy, efficient, and effective.
- [Horovod](https://github.com/horovod/horovod)  - Distributed training framework for TensorFlow, Keras, PyTorch, and Apache MXNet.
- [Jax](https://github.com/google/jax)  - Autograd and XLA for high-performance machine learning research.
- [Kedro](https://github.com/kedro-org/kedro)  - Kedro is an open-source Python framework for creating reproducible, maintainable and modular data science code.
- [Keras](https://github.com/keras-team/keras)  - Keras is a deep learning API written in Python, running on top of the machine learning platform TensorFlow.
- [LightGBM](https://github.com/microsoft/LightGBM)  - A fast, distributed, high performance gradient boosting (GBT, GBDT, GBRT, GBM or MART) framework based on decision tree algorithms, used for ranking, classification and many other machine learning tasks.
- [MegEngine](https://github.com/MegEngine/MegEngine)  - MegEngine is a fast, scalable and easy-to-use deep learning framework, with auto-differentiation.
- [metric-learn](https://github.com/scikit-learn-contrib/metric-learn)  - Metric Learning Algorithms in Python.
- [MindSpore](https://github.com/mindspore-ai/mindspore)  - MindSpore is a new open source deep learning training/inference framework that could be used for mobile, edge and cloud scenarios.
- [Oneflow](https://github.com/Oneflow-Inc/oneflow)  - OneFlow is a performance-centered and open-source deep learning framework.
- [PaddlePaddle](https://github.com/PaddlePaddle/Paddle)  - Machine Learning Framework from Industrial Practice.
- [PyTorch](https://github.com/pytorch/pytorch)  - Tensors and Dynamic neural networks in Python with strong GPU acceleration.
- [PyTorch Lightning](https://github.com/lightning-AI/lightning)  - Deep learning framework to train, deploy, and ship AI products Lightning fast.
- [XGBoost](https://github.com/dmlc/xgboost)  - Scalable, Portable and Distributed Gradient Boosting (GBDT, GBRT or GBM) Library.
- [scikit-learn](https://github.com/scikit-learn/scikit-learn)  - Machine Learning in Python.
- [TensorFlow](https://github.com/tensorflow/tensorflow)  - An Open Source Machine Learning Framework for Everyone.
- [VectorFlow](https://github.com/Netflix/vectorflow)  - A minimalist neural network library optimized for sparse data and single machine environments.
## LLMOps| 名称 | Stars 数 | 介绍 |
| ------------------------------------------------------- | ------------------------------------------------------------ | ------------------------------------------------------------ |
| [Byzer-LLM](https://github.com/allwefantasy/byzer-llm) |  | Byzer-LLM是一套大模型基础设施,支持预训练,微调,部署,serving等各种和大模型相关的能力。Byzer-Retrieval则是专门为大模型开发的存储基础设施,支持各种数据源批量导入,实时单条更新,支持全文检索,向量检索,混合检索,方便Byzer-LLM使用数据。Byzer-SQL/Python则提供了易于使用的人机交互API,极低门槛去使用上述产品。 |
| [agenta](https://github.com/Agenta-AI/agenta) |  | 用于构建强大LLM应用的LLMOps平台。轻松尝试和评估不同提示、模型和工作流,以构建稳健的应用程序。 |
| [Arize-Phoenix](https://github.com/Arize-ai/phoenix) |  | 用于LLMs、视觉、语言和表格模型的ML可观测性。 |
| [BudgetML](https://github.com/ebhy/budgetml) |  | 在不到10行代码的情况下,以有限的预算部署ML推理服务。 |
| [CometLLM](https://github.com/comet-ml/comet-llm) |  | 100%开源的LLMOps平台,用于记录、管理和可视化LLM提示和链条。跟踪提示模板、提示变量、提示持续时间、令牌使用等其他元数据。评分提示输出并在单个UI中可视化聊天历史。 |
| [deeplake](https://github.com/activeloopai/deeplake) |  | 流式传输大型多模态数据集,实现接近100%的GPU利用率。查询、可视化和版本控制数据。无需重新计算模型微调的嵌入即可访问数据。 |
| [Dify](https://github.com/langgenius/dify) |  | 开源框架旨在使开发人员(甚至非开发人员)能够快速构建基于大型语言模型的有用应用程序,确保它们具有可视性、可操作性和可改进性。 |
| [Dstack](https://github.com/dstackai/dstack) |  | 在任何云(AWS、GCP、Azure、Lambda等)中进行经济高效的LLM开发。 |
| [Embedchain](https://github.com/embedchain/embedchain) |  | 用于在数据集上创建类似ChatGPT的机器人的框架。 |
| [GPTCache](https://github.com/zilliztech/GPTCache) |  | 创建语义缓存以存储LLM查询的响应。 |
| [Haystack](https://github.com/deepset-ai/haystack) |  | 快速构建带有LLM代理、语义搜索、问答等功能的应用程序。 |
| [langchain](https://github.com/hwchase17/langchain) |  | 通过可组合性构建LLM应用程序。 |
| [LangFlow](https://github.com/logspace-ai/langflow) |  | 通过拖放组件和聊天界面轻松实验和原型化LangChain流程的无忧方式。 |
| [LangKit](https://github.com/whylabs/langkit) |  | 开箱即用的LLM遥测收集库,可提取有关LLM随时间性能如何的特征和提示、响应和元数据的配置文件,以规模找到问题。 |
| [LiteLLM 🚅](https://github.com/BerriAI/litellm/) |  | 一个简单且轻量的100行包,用于跨OpenAI、Azure、Cohere、Anthropic、Replicate API端点标准化LLM API调用。 |
| [LlamaIndex](https://github.com/jerryjliu/llama_index) |  | 提供一个中央接口,将您的LLMs与外部数据连接起来。 |
| [LLMApp](https://github.com/pathwaycom/llm-app) |  | LLM App是一个Python库,帮助您以几行代码构建实时的LLM启用数据管道。 |
| [LLMFlows](https://github.com/stoyan-stoyanov/llmflows) |  | LLMFlows是一个用于构建简单、明确和透明的LLM应用程序的框架,例如聊天机器人、问答系统和代理。 |
| [LLMonitor](https://github.com/llmonitor/llmonitor) |  | 用于AI应用程序和代理的可观测性和监控。通过强大的跟踪和日志记录来调试代理。使用分析工具深入研究请求历史。开发人员友好的模块,可以轻松集成到LangChain中。 |
| [magentic](https://github.com/jackmpcollins/magentic) |  | 无缝集成LLMs作为Python函数。使用类型注释来指定结构化输出。将LLM查询和函数调用与常规Python代码混合使用,创建复杂的LLM驱动功能。 |
| [Pezzo 🕹️](https://github.com/pezzolabs/pezzo) |  | Pezzo是为开发人员和团队构建的开源LLMOps平台。仅需两行代码,您就可以轻松排除AI操作中的问题,协作和管理您的提示,在一个地方立即部署更改。 |
| [promptfoo](https://github.com/typpo/promptfoo) |  | 用于测试和评估提示质量的开源工具。创建测试用例,自动检查输出质量并捕获回归,降低评估成本。 |
| [prompttools](https://github.com/hegelai/prompttools) |  | 用于测试和尝试提示的开源工具。核心思想是使开发人员能够使用熟悉的界面(如代码和笔记本)评估提示。只需几行代码,您就可以在不同模型之间测试提示和参数(无论您是使用OpenAI、Anthropic还是LLaMA模型)。您甚至可以评估向量数据库的检索准确性。 |
| [TrueFoundry](https://www.truefoundry.com/) | 无Github链接 | 在自己的Kubernetes(EKS、AKS、GKE、On-prem)基础设施上部署LLMOps工具,包括Vector DBs、嵌入式服务器等,包括部署、微调、跟踪提示和提供完整的数据安全性和最佳GPU管理的开源LLM模型。使用最佳的软件工程实践在生产规模上训练和启动您的LLM应用程序。 |
| [ReliableGPT 💪](https://github.com/BerriAI/reliableGPT/) |  | 为您的生产LLM应用程序处理OpenAI错误(超载的OpenAI服务器、旋转的密钥或上下文窗口错误)。 |
| [Weights & Biases (Prompts)](https://docs.wandb.ai/guides/prompts) | 无Github链接 | 开发者优先的W&B MLOps平台中的一套LLMOps工具。利用W&B Prompts来可视化和检查LLM执行流程,跟踪输入和输出,查看中间结果,安全管理提示和LLM链配置。 |
| [xTuring](https://github.com/stochasticai/xturing) |  | 使用快速和高效的微调来构建和控制您的个人LLMs。 |
| [ZenML](https://github.com/zenml-io/zenml) |  | 用于编排、实验和部署生产级ML解决方案的开源框架,具有内置的`langchain`和`llama_index`集成。 |
## Courses- [大语言模型课程notebooks集-Large Language Model Course](https://github.com/mlabonne/llm-course) - Course with a roadmap and notebooks to get into Large Language Models (LLMs).
- [Full+Stack+LLM+Bootcamp](https://ihower.tw/notes/技術筆記-AI/Full+Stack+LLM+Bootcamp) - LLM相关学习/应用资源集.
## Others- [Evaluating Language Models by OpenAI, DeepMind, Google, Microsoft](https://levelup.gitconnected.com/how-to-benchmark-language-models-by-openai-deepmind-google-microsoft-783d4307ec50) - Evaluating Language Models by OpenAI, DeepMind, Google, Microsoft.
- [Efficient Finetuning of Quantized LLMs --- 低资源的大语言模型量化训练/部署方案](https://github.com/jianzhnie/Efficient-Tuning-LLMs) - 旨在构建和开源遵循指令的baichuan/LLaMA/Pythia/GLM中文大模型微调训练方法,该方法可以在单个 Nvidia RTX-2080TI上进行训练,多轮聊天机器人可以在单个 Nvidia RTX-3090上进行上下文长度 2048的模型训练。使用bitsandbytes进行量化,并与Huggingface的PEFT和transformers库集成.
## Other-Awesome-Lists- [Awesome LLM](https://github.com/Hannibal046/Awesome-LLM/) - A curated list of papers about large language models.
- [Awesome-Efficient-LLM](https://github.com/horseee/Awesome-Efficient-LLM) - A curated list for Efficient Large Language Models.
- [Awesome-production-machine-learning](https://github.com/EthicalML/awesome-production-machine-learning) - A curated list of awesome open source libraries to deploy, monitor, version and scale your machine learning.
- [Awesome-marketing-datascience](https://github.com/underlines/awesome-marketing-datascience) - Curated list of useful LLM / Analytics / Datascience resources.
- [Awesome-llm-tools](https://github.com/underlines/awesome-marketing-datascience/blob/master/llm-tools.md) - Curated list of useful LLM tool.
- [Awesome-LLM-Compression](https://github.com/HuangOwen/Awesome-LLM-Compression) - A curated list for Efficient LLM Compression.
- [Awesome-Multimodal-Large-Language-Models](https://github.com/BradyFU/Awesome-Multimodal-Large-Language-Models) - A curated list of Multimodal Large Language Models.
- [Awesome-LLMOps](https://github.com/tensorchord/Awesome-LLMOps) - An awesome & curated list of the best LLMOps tools for developers.
- [Awesome-MLops](https://github.com/visenger/awesome-mlops) - An awesome list of references for MLOps - Machine Learning Operations.
- [Awesome ChatGPT Prompts](https://github.com/f/awesome-chatgpt-prompts) - A collection of prompt examples to be used with the ChatGPT model.
- [awesome-chatgpt-prompts-zh](https://github.com/PlexPt/awesome-chatgpt-prompts-zh) - A Chinese collection of prompt examples to be used with the ChatGPT model.
- [Awesome ChatGPT](https://github.com/humanloop/awesome-chatgpt) - Curated list of resources for ChatGPT and GPT-3 from OpenAI.
- [Chain-of-Thoughts Papers](https://github.com/Timothyxxx/Chain-of-ThoughtsPapers) - A trend starts from "Chain of Thought Prompting Elicits Reasoning in Large Language Models.
- [Instruction-Tuning-Papers](https://github.com/SinclairCoder/Instruction-Tuning-Papers) - A trend starts from `Natrural-Instruction` (ACL 2022), `FLAN` (ICLR 2022) and `T0` (ICLR 2022).
- [LLM Reading List](https://github.com/crazyofapple/Reading_groups/) - A paper & resource list of large language models.
- [Reasoning using Language Models](https://github.com/atfortes/LM-Reasoning-Papers) - Collection of papers and resources on Reasoning using Language Models.
- [Chain-of-Thought Hub](https://github.com/FranxYao/chain-of-thought-hub) - Measuring LLMs' Reasoning Performance
- [Awesome GPT](https://github.com/formulahendry/awesome-gpt) - A curated list of awesome projects and resources related to GPT, ChatGPT, OpenAI, LLM, and more.
- [Awesome GPT-3](https://github.com/elyase/awesome-gpt3) - a collection of demos and articles about the [OpenAI GPT-3 API](https://openai.com/blog/openai-api/).
## Licenses[](https://lbesson.mit-license.org/)
本项目遵循 [MIT License](https://lbesson.mit-license.org/).
[](http://creativecommons.org/licenses/by-nc-sa/4.0/)
本项目遵循 [Creative Commons Attribution-NonCommercial-ShareAlike 4.0 International License](http://creativecommons.org/licenses/by-nc-sa/4.0/).
## 引用如果我们的项目对您有帮助,请引用我们的项目。
```
@misc{junwang2023,
author = {Jun Wang, Pengyong Li, Liwei Liu, Xuebin Qin, Junchi Yan},
title = {Awesome-LLM-Eval: a curated list of tools, benchmarks, demos, papers for Large Language Models Evaluation},
year = {2023},
publisher = {GitHub},
journal = {GitHub repository},
howpublished = {\url{https://github.com/onejune2018/Awesome-LLM-Eval}},
}
```作者信息:
- 简介:500强企业AI平台部算法研发负责人,前IBM研究院研究员,ETH访问学者,北京大学博士
- 研发:图网络/视觉、深度学习、大模型、空间科学等,医药发现大模型[MPG](https://github.com/onejune2018/MPG)作者
- 荣誉:国际竞赛冠军(SemEval2022习语判别赛道、MIT AI-Cure、VQA2021、TREC2021、EAD2019等)
- 主页:https://onejune2018.github.io/homepage/
- Google Scholar: https://scholar.google.com/citations?user=0Be01PgAAAAJ&hl=en