https://github.com/xiaoganghan/awesome-feature-engineering
A curated list of feature engineering techniques for image and text machine learning
https://github.com/xiaoganghan/awesome-feature-engineering
List: awesome-feature-engineering
deep-learning feature-engineering machine-learning
Last synced: 6 months ago
JSON representation
A curated list of feature engineering techniques for image and text machine learning
- Host: GitHub
- URL: https://github.com/xiaoganghan/awesome-feature-engineering
- Owner: xiaoganghan
- License: mit
- Created: 2017-02-28T06:21:40.000Z (over 8 years ago)
- Default Branch: master
- Last Pushed: 2017-04-11T06:52:22.000Z (about 8 years ago)
- Last Synced: 2024-05-23T03:09:18.022Z (about 1 year ago)
- Topics: deep-learning, feature-engineering, machine-learning
- Homepage:
- Size: 4.88 KB
- Stars: 50
- Watchers: 7
- Forks: 11
- Open Issues: 0
-
Metadata Files:
- Readme: README.md
- License: LICENSE
Awesome Lists containing this project
- ultimate-awesome - awesome-feature-engineering - A curated list of feature engineering techniques for image and text machine learning. (Other Lists / Julia Lists)
README
# awesome-feature-engineering [](https://github.com/jtoy/awesome)
A curated list of feature engineering techniques for image and text machine learning.## Table of Contents
- [Image](#image)
- [Text](#text)
- [General](#general)## Image
### brightness, contrast, saturation
#### sample implementations
1. [mxnet](https://github.com/dmlc/mxnet/blob/master/python/mxnet/image.py)#### use cases
1. [Updated! My 99.40% solution to Udacity Nanodegree project P2 (Traffic Sign Classification)](https://medium.com/@hengcherkeng/updated-my-99-40-solution-to-udacity-nanodegree-project-p2-traffic-sign-classification-5580ae5bd51f#.4hwecy9m6)
### spatial transformer
#### sample implementations
1. [Traffic sign recognition with Torch](https://github.com/Moodstocks/gtsrb.torch)#### use cases
1. [The power of Spatial Transformer Networks](http://torch.ch/blog/2015/09/07/spatial_transformers.html)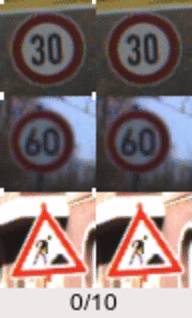
### histogram equalization
#### sample implementations
1. [Traffic signs classification with a convolutional network](http://navoshta.com/traffic-signs-classification/)#### use cases
1. [Traffic signs classification with a convolutional network](http://navoshta.com/traffic-signs-classification/)

### flipping
#### sample implementations
1. [Traffic signs classification with a convolutional network](http://navoshta.com/traffic-signs-classification/)#### use cases
1. [Traffic signs classification with a convolutional network](http://navoshta.com/traffic-signs-classification/)

### rotation and projection
#### sample implementations
1. [Traffic signs classification with a convolutional network](http://navoshta.com/traffic-signs-classification/)#### use cases
1. [Traffic signs classification with a convolutional network](http://navoshta.com/traffic-signs-classification/)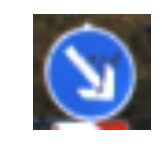

### others
zooming, cropping, panning, minor color changes### Libraries
* [imgaug: Image augmentation for machine learning experiments.](https://github.com/aleju/imgaug)
## Text
### stemmer
#### sample implementations
1. [nltk](http://www.nltk.org/_modules/nltk/stem/porter.html)#### use cases
1. [Q&A With Job Salary Prediction First Prize Winner Vlad Mnih](http://blog.kaggle.com/2013/05/06/qa-with-job-salary-prediction-first-prize-winner-vlad-mnih/)### tf-idf
#### sample implementations
1. [sklearn](http://scikit-learn.org/stable/modules/generated/sklearn.feature_extraction.text.TfidfVectorizer.html)#### use cases
1. [Is That a Duplicate Quora Question?](https://www.linkedin.com/pulse/duplicate-quora-question-abhishek-thakur)### svd
#### sample implementations
1. [sklearn](http://scikit-learn.org/stable/modules/generated/sklearn.decomposition.TruncatedSVD.html)#### use cases
1. [Is That a Duplicate Quora Question?](https://www.linkedin.com/pulse/duplicate-quora-question-abhishek-thakur)### PCA
### word2vec
#### sample implementations
1. [gensim](http://radimrehurek.com/gensim/)#### use cases
1. [Is That a Duplicate Quora Question?](https://www.linkedin.com/pulse/duplicate-quora-question-abhishek-thakur)
2. [Do-it-yourself NLP for bot developers](https://conversations.golastmile.com/do-it-yourself-nlp-for-bot-developers-2e2da2817f3d#.9yz22bhzp) (text classification, entity recognition)#### pipeline
* [Document Classification with scikit-learn](http://zacstewart.com/2015/04/28/document-classification-with-scikit-learn.html)
* use separate bags of words for different attributes of text#### general feature engineering
* [特征工程到底是什么?(in Chinese)](https://www.zhihu.com/question/29316149)
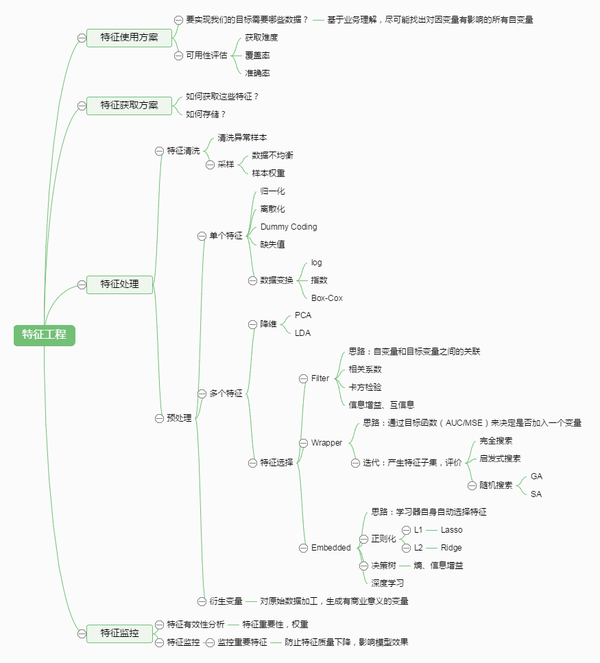
* [Image augmentation for machine learning experiments.](https://github.com/aleju/imgaug)
### feature engieering pipelines
* [Tips & Tricks for Feature Engineering / Applied Machine Learning](https://www.slideshare.net/HJvanVeen/feature-engineering-72376750)
* represent categorical features using 1-of-K encoding### data exploration with Pandas
* [Data Wrangling with Pandas](http://nbviewer.jupyter.org/urls/gist.github.com/fonnesbeck/5850413/raw/3a9406c73365480bc58d5e75bc80f7962243ba17/2.+Data+Wrangling+with+Pandas.ipynb)
* [A Rubric for Data Wrangling and Exploration](http://nbviewer.jupyter.org/github/cs109/content/blob/master/lec_04_wrangling.ipynb)### feed data to model
1. [Is That a Duplicate Quora Question?](https://www.linkedin.com/pulse/duplicate-quora-question-abhishek-thakur)